IDM-Follower: A Model-Informed Deep Learning Method for Long-Sequence Car-Following Trajectory Prediction
arxiv(2022)
摘要
Model-based and learning-based methods are two major types of methodologies to model car following behaviors. Model-based methods describe the car-following behaviors with explicit mathematical equations, while learning-based methods focus on getting a mapping between inputs and outputs. Both types of methods have advantages and weaknesses. Meanwhile, most car-following models are generative and only consider the inputs of the speed, position, and acceleration of the last time step. To address these issues, this study proposes a novel framework called IDM-Follower that can generate a sequence of following vehicle trajectory by a recurrent autoencoder informed by a physical car-following model, the Intelligent Driving Model (IDM).We implement a novel structure with two independent encoders and a self-attention decoder that could sequentially predict the following trajectories. A loss function considering the discrepancies between predictions and labeled data integrated with discrepancies from model-based predictions is implemented to update the neural network parameters. Numerical experiments with multiple settings on simulation and NGSIM datasets show that the IDM-Follower can improve the prediction performance compared to the model-based or learning-based methods alone. Analysis on different noise levels also shows good robustness of the model.
更多查看译文
关键词
deep learning,prediction,idm-follower,model-informed,long-sequence,car-following
AI 理解论文
溯源树
样例
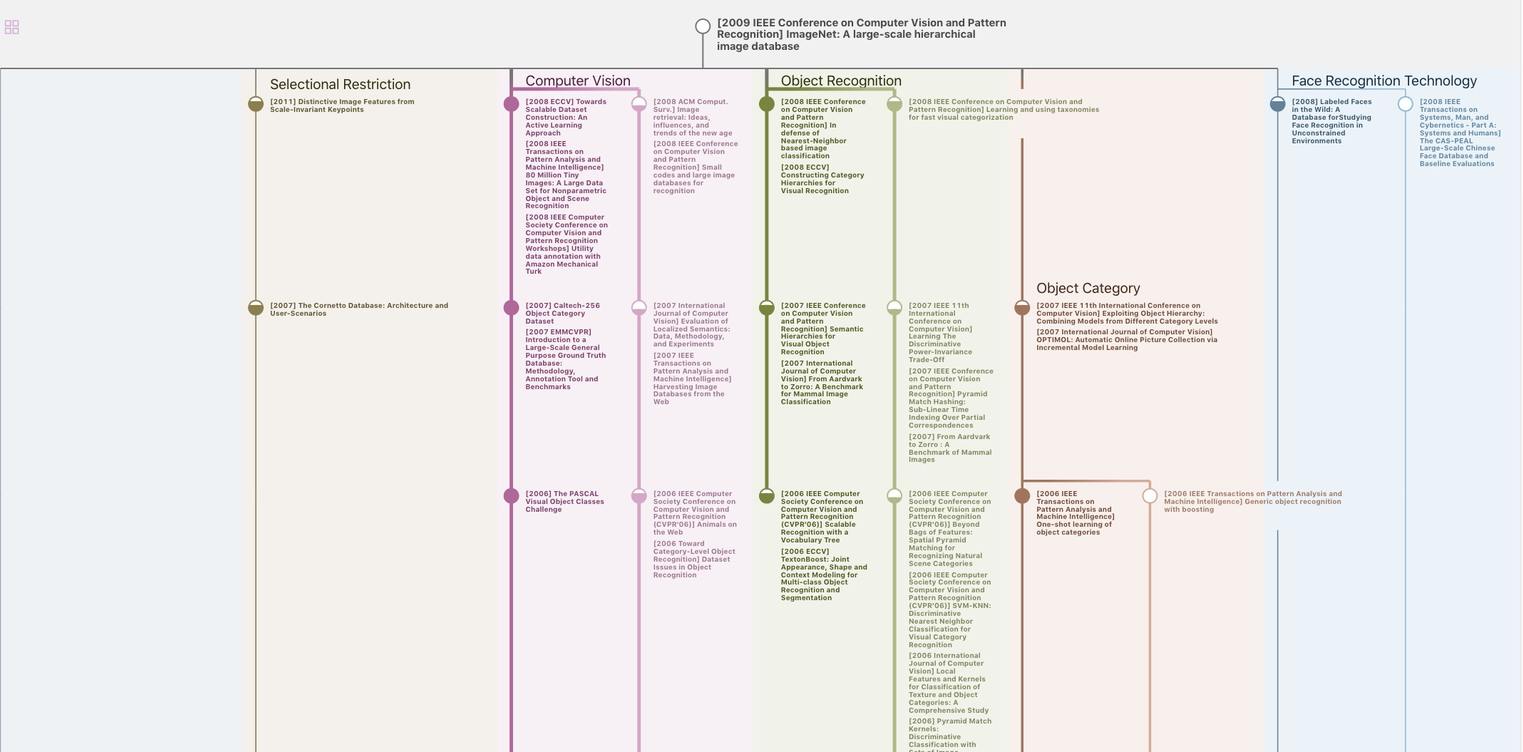
生成溯源树,研究论文发展脉络
Chat Paper
正在生成论文摘要