Machine-Learning-Assisted Analysis of TCR Profiling Data Unveils Cross-Reactivity between SARS-CoV-2 and a Wide Spectrum of Pathogens and Other Diseases
BIOLOGY-BASEL(2022)
摘要
Simple Summary For the last two years, COVID-19 has been rigorously studied aiming to identify novel prognostic and therapeutic avenues. Recently, T cell receptor profiling has emerged as a method to associate adaptive immunity with COVID-19 progression and severity. Such data are typically analyzed to explore T cell receptor properties and characteristics in the context of SARS-CoV-2 infection. However, the equally informative alternative analytic strategy of identifying any preferential recognition of viral antigens by the T-cell-mediated immune response is mostly overlooked. In this study, we propose a novel Machine-Learning-oriented approach for analyzing T cell receptor repertoires that is based on the concept of utilising the level at which each SARS-CoV-2 antigen is recognised by the available T cell receptors in each sample from COVID-19-convalescent and healthy cohorts. This approach also allowed us to observe a group of T cell receptors capable of recognising SARS-CoV-2 antigens that were already established in samples from the healthy cohort, leading us to the cross-reactivity phenomenon hypothesis. To explore this, all T cell receptors were examined for being able to recognise antigens from other pathogens and diseases, unveiling evidence of putative cross-reactivity with M. tuberculosis and Influenza virus, among others. During the last two years, the emergence of SARS-CoV-2 has led to millions of deaths worldwide, with a devastating socio-economic impact on a global scale. The scientific community's focus has recently shifted towards the association of the T cell immunological repertoire with COVID-19 progression and severity, by utilising T cell receptor sequencing (TCR-Seq) assays. The Multiplexed Identification of T cell Receptor Antigen (MIRA) dataset, which is a subset of the immunoACCESS study, provides thousands of TCRs that can specifically recognise SARS-CoV-2 epitopes. Our study proposes a novel Machine Learning (ML)-assisted approach for analysing TCR-Seq data from the antigens' point of view, with the ability to unveil key antigens that can accurately distinguish between MIRA COVID-19-convalescent and healthy individuals based on differences in the triggered immune response. Some SARS-CoV-2 antigens were found to exhibit equal levels of recognition by MIRA TCRs in both convalescent and healthy cohorts, leading to the assumption of putative cross-reactivity between SARS-CoV-2 and other infectious agents. This hypothesis was tested by combining MIRA with other public TCR profiling repositories that host assays and sequencing data concerning a plethora of pathogens. Our study provides evidence regarding putative cross-reactivity between SARS-CoV-2 and a wide spectrum of pathogens and diseases, with M. tuberculosis and Influenza virus exhibiting the highest levels of cross-reactivity. These results can potentially shift the emphasis of immunological studies towards an increased application of TCR profiling assays that have the potential to uncover key mechanisms of cell-mediated immune response against pathogens and diseases.
更多查看译文
关键词
COVID-19,T cell receptor,pathogens,diseases,cross-reactivity phenomenon,Machine Learning,MIRA dataset
AI 理解论文
溯源树
样例
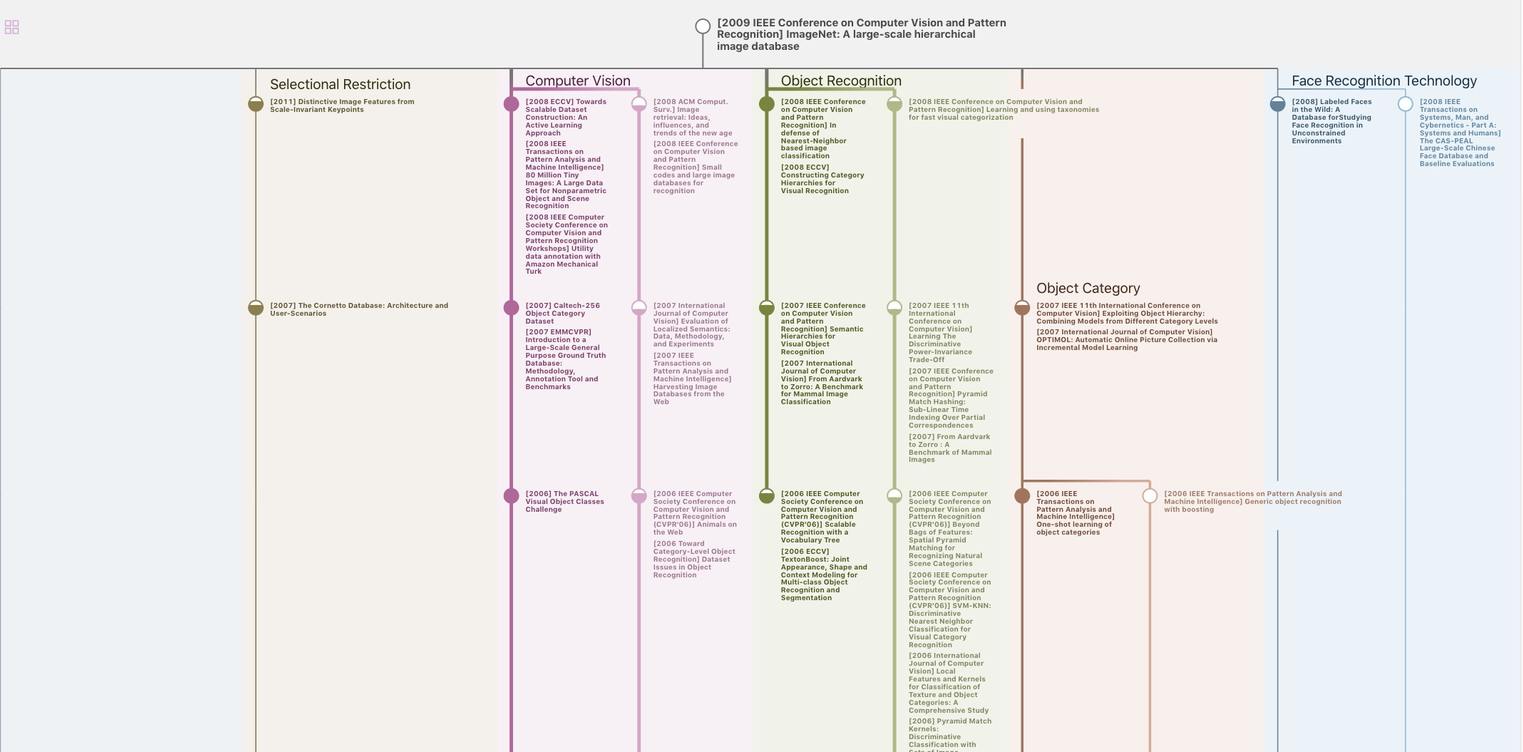
生成溯源树,研究论文发展脉络
Chat Paper
正在生成论文摘要