On the Power of Pre-training for Generalization in RL: Provable Benefits and Hardness
arxiv(2022)
摘要
Generalization in Reinforcement Learning (RL) aims to learn an agent during training that generalizes to the target environment. This paper studies RL generalization from a theoretical aspect: how much can we expect pre-training over training environments to be helpful? When the interaction with the target environment is not allowed, we certify that the best we can obtain is a near-optimal policy in an average sense, and we design an algorithm that achieves this goal. Furthermore, when the agent is allowed to interact with the target environment, we give a surprising result showing that asymptotically, the improvement from pre-training is at most a constant factor. On the other hand, in the non-asymptotic regime, we design an efficient algorithm and prove a distribution-based regret bound in the target environment that is independent of the state-action space.
更多查看译文
关键词
Reinforcement Learning,Generalization,Learning Theory
AI 理解论文
溯源树
样例
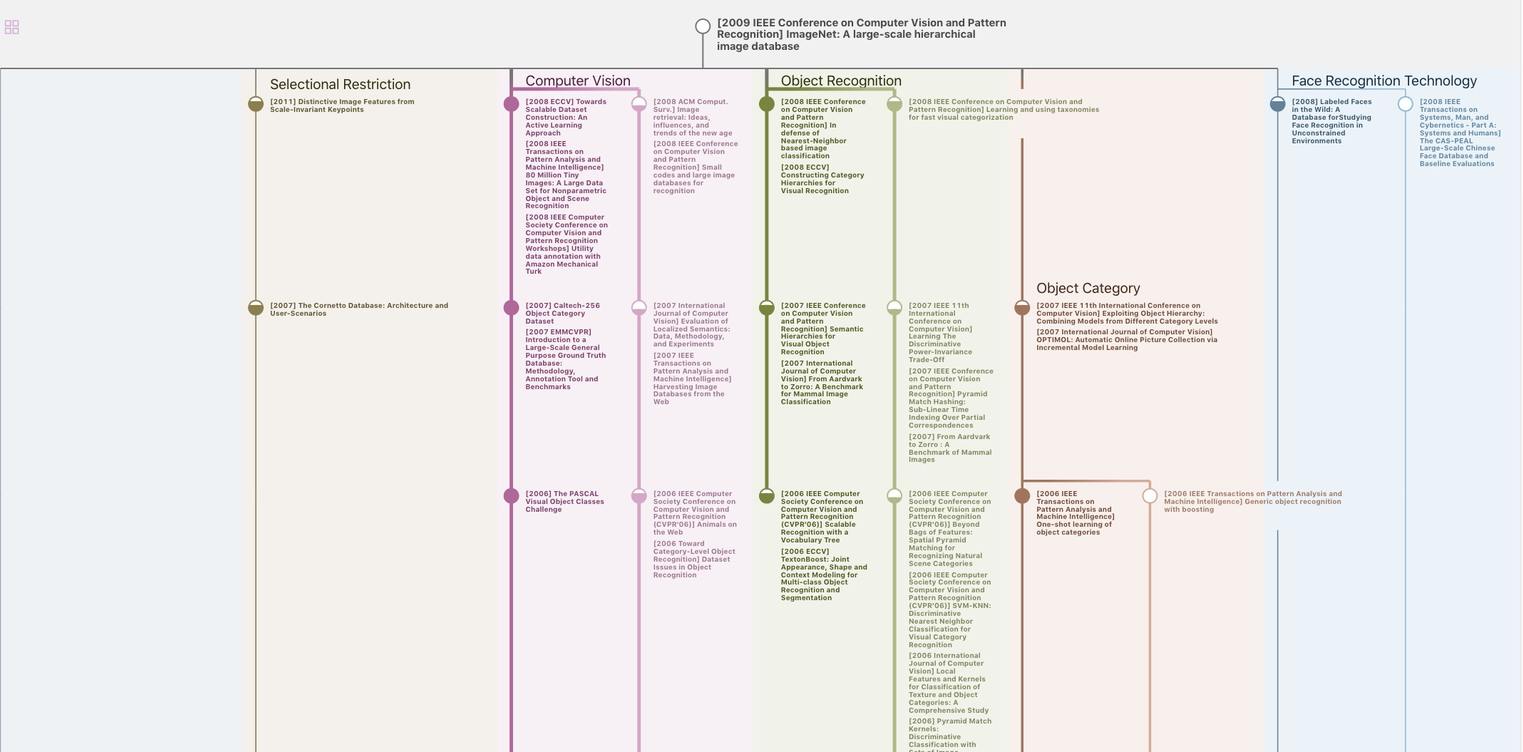
生成溯源树,研究论文发展脉络
Chat Paper
正在生成论文摘要