Discovering Limitations of Image Quality Assessments with Noised Deep Learning Image Sets
arxiv(2023)
摘要
Image quality is important, and can affect overall performance in image processing and computer vision as well as for numerous other reasons. Image quality assessment (IQA) is consequently a vital task in different applications from aerial photography interpretation to object detection to medical image analysis. In previous research, the BRISQUE algorithm and the PSNR algorithm were evaluated with high resolution (atleast 512x384 pixels), but relatively small image sets (no more than 4,744 images). However, scientists have not evaluated IQA algorithms on low resolution (no more than 32x32 pixels), multi-perturbation, big image sets (for example, tleast 60,000 different images not counting their perturbations). This study explores these two IQA algorithms through experimental investigation. We first chose two deep learning image sets, CIFAR-10 and MNIST. Then, we added 68 perturbations that add noise to the images in specific sequences and noise intensities. In addition, we tracked the performance outputs of the two IQA algorithms with singly and multiply noised images. After quantitatively analyzing experimental results, we report the limitations of the two IQAs with these noised CIFAR-10 and MNIST image sets. We also explain three potential root causes for performance degradation. These findings point out weaknesses of the two IQA algorithms. The research results provide guidance to scientists and engineers developing accurate, robust IQA algorithms. All source codes, related image sets, and figures are shared on the website (https://github.com/caperock/imagequality) to support future scientific and industrial projects.
更多查看译文
关键词
image quality assessments,deep learning
AI 理解论文
溯源树
样例
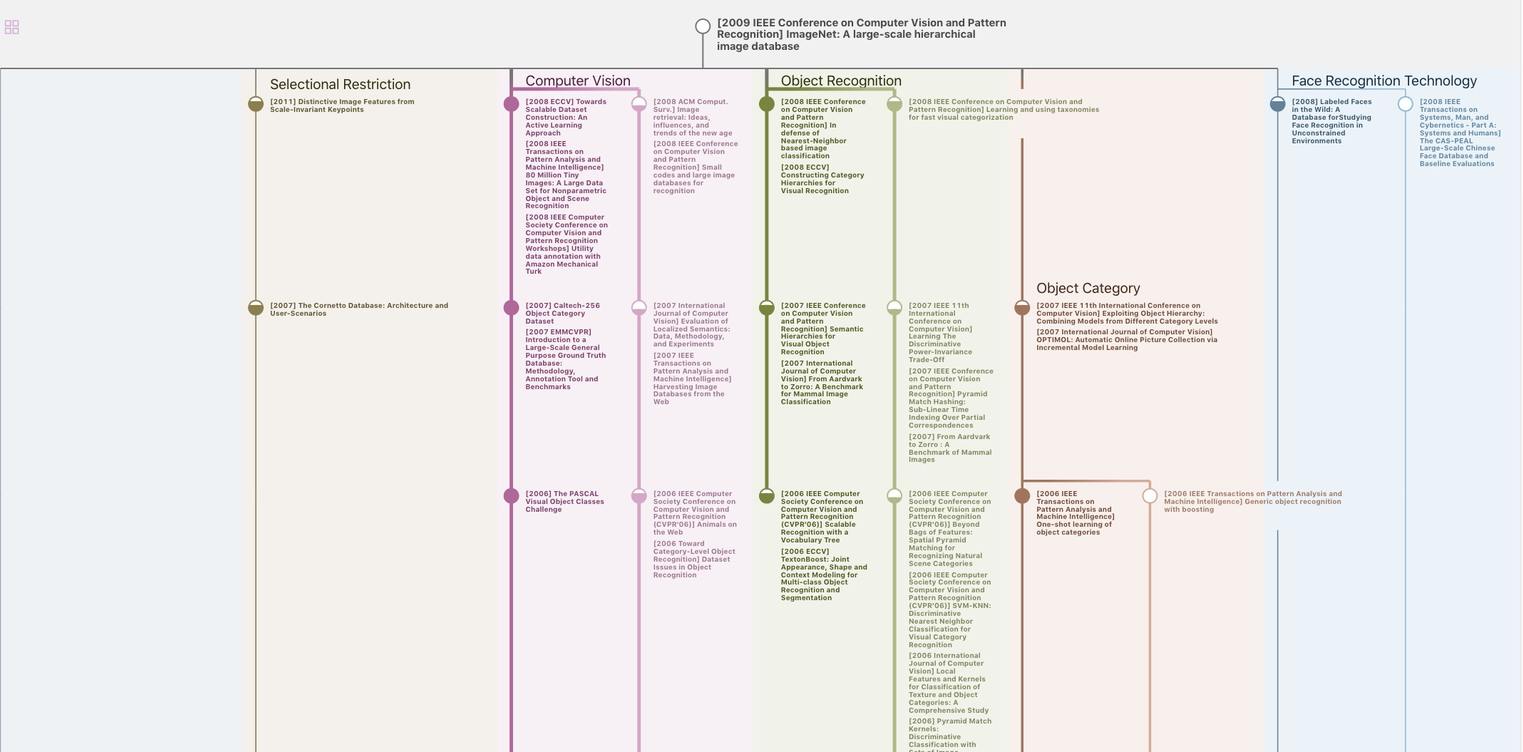
生成溯源树,研究论文发展脉络
Chat Paper
正在生成论文摘要