EMS-CDNet: an efficient multi-scale-fusion change detection network for very high-resolution remote sensing images
International Journal of Remote Sensing(2022)
摘要
Remote sensing image change detection (RSICD) is an essential measure for monitoring the earth's surface changes. In recent years, the explosive growth of very high-resolution (VHR) satellite sensors and the booming innovations in deep learning technology have significantly boosted RSICD development. However, most of the current RSICD models focus on locating accurate change areas while ignoring the efficiency of their method, which limits the practical application of RSICD models, especially for large-scale and emergency RSICD tasks. In this paper, we propose an Efficient Multi-scale-fusion Change Detection Network (EMS-CDNet) for bi-temporal RSICD tasks. Our EMS-CDNet pays more attention to the model's inference speed and the accuracy-efficiency trade-off rather than only pursuing detection accuracy. We designed a multi-scale fusion module for EMS-CDNet, which adopts multi-scale and multi-branch operations to extract multi-scale features simultaneously and aggregate features at different feature levels. In addition to EMS-CDNet's ability to achieve sufficient feature extraction, the multi-scale image input within the designed module alleviates the influence of image registration errors in practical applications, thereby strengthening EMS-CDNet's value for practical RSICD tasks. We also integrated a novel partition unit in EMS-CDNet to lighten the model while maintaining the detection ability of small targets, thus shortening its processing time without a severe accuracy decrease. We conducted experiments on two state-of-the-art (SOTA) public RSICD datasets and our own collected dataset. The public datasets were utilized to comparatively measure the overall accuracy and efficiency measurement of EMS-CDNet, and the dataset of images we collected was used to observe EMS-CDNet's performance under the influence of image registration errors. Our experimental results show that EMS-CDNet achieved a better accuracy-efficiency trade-off than the SOTA public datasets methods. For example, EMS-CDNet reduced the inference time by about 33% while maintaining identical detection accuracy to CLNet (the optimal method among the comparison methods). Furthermore, EMS-CDNet achieved higher accuracy on our collected dataset, with an F1 of 74% and mIoU of 0.806, demonstrating its robustness to image registration errors and showing its value for practical RSICD applications.
更多查看译文
关键词
Change detection (CD), Multi, scale, fusion module, partition unit, accuracy, efficiency trade, off
AI 理解论文
溯源树
样例
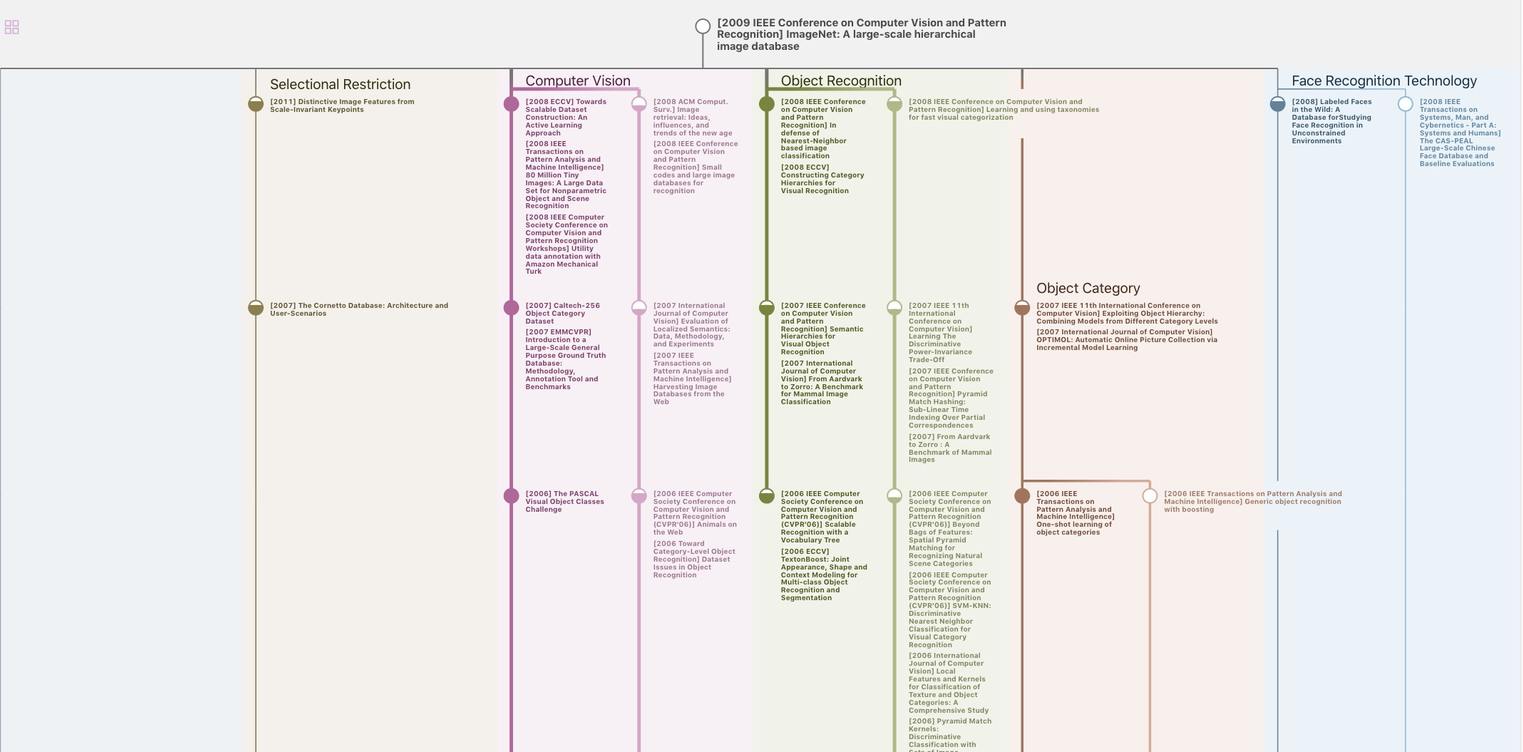
生成溯源树,研究论文发展脉络
Chat Paper
正在生成论文摘要