Dynamic-scale graph neural network for fault detection
Process Safety and Environmental Protection(2022)
摘要
Traditional graph-based dynamic fault detection methods describe the dynamic characteristic through constructing a single neighborhood graph at the current sample with some history samples. However, they ignore the diversity of dynamic properties of the variables in complex chemical processes. To overcome this problem, a novel neural network structure combining multiscale subgraphs is proposed, named dynamic-scale graph neural network (DSGNN), which divides variables into multiple groups according to their dynamic properties. DSGNN constructs a subgraph in each group. In traditional graph-based methods, the scale of the graph is usually manually designed. In DSGNN, the scale of each subgraph is decided by the dynamic properties of the variables in this subgraph. To aggregate the dynamic information, DSGNN utilizes convolution operations. The weights assigned to the neighbors in each subgraph are determined according to the similarity between the current data and its neighbors. Low-dimensional features are extracted through the back-propagation technique from the updated high-dimensional features produced by convolution operations. Two case studies on a multivariate dynamic process and the Tennessee Eastman process are conducted to show the superiority of DSGNN.
更多查看译文
关键词
Process monitoring,Fault detection,Dynamic feature extraction,Dimension reduction
AI 理解论文
溯源树
样例
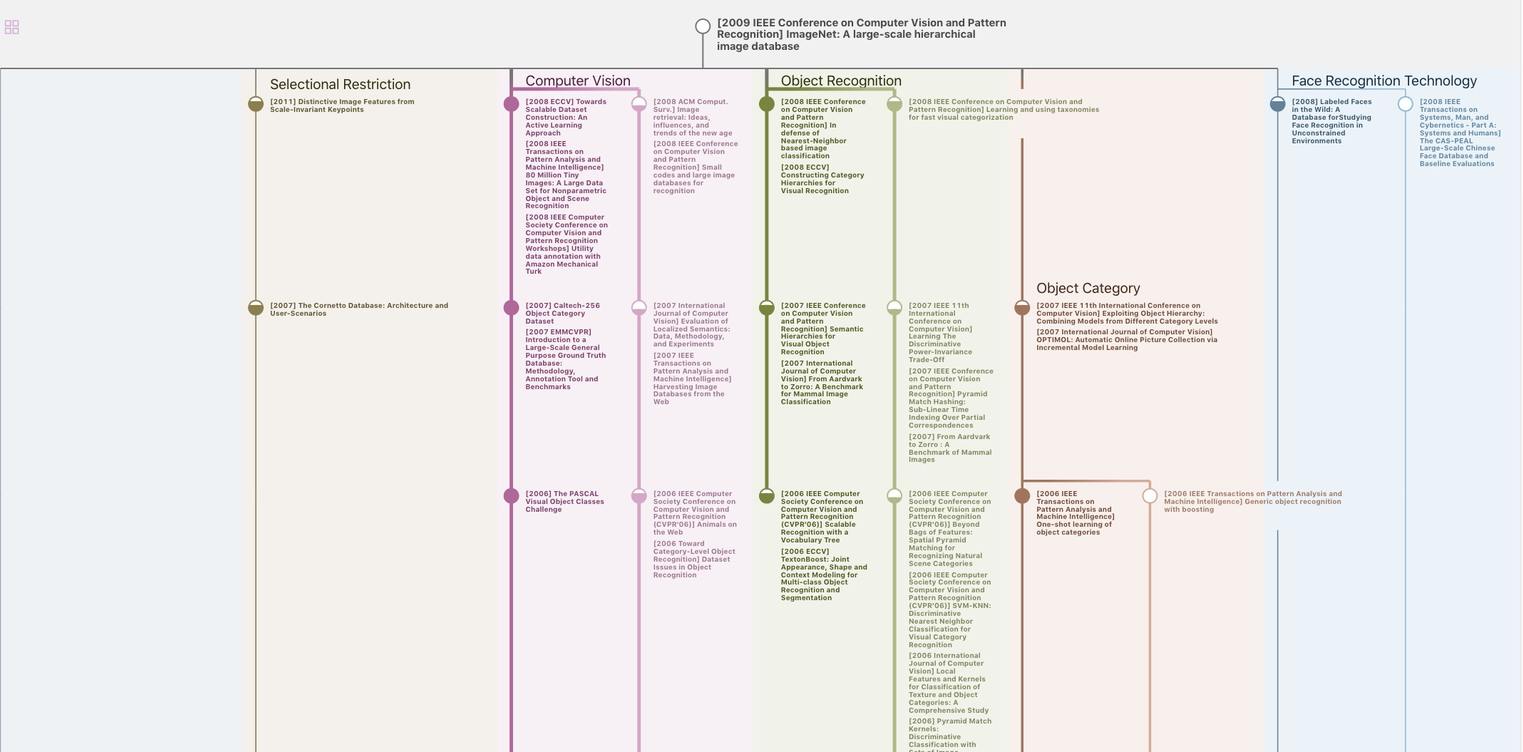
生成溯源树,研究论文发展脉络
Chat Paper
正在生成论文摘要