MEED: A novel robust contrast enhancement procedure yielding highly-convergent thresholding of biofilm images
COMPUTERS IN BIOLOGY AND MEDICINE(2022)
摘要
Morphological and statistical investigation of biofilm images may be even more critical than the image acqui-sition itself, in particular in the presence of morphologically complex distributions, due to the unavoidable impact of the measurement technique too. Hence, digital image pre-processing is mandatory for reliable feature extraction and enhancement preliminary to segmentation. Also, pattern recognition in automated deep learning (both supervised and unsupervised) models often requires a preliminary effective contrast-enhancement. How-ever, no universal consensus exists on the optimal contrast enhancement approach.This paper presents and discusses a new general, robust, reproducible, accurate and easy to implement contrast enhancement procedure, briefly named MEED-procedure, able to work on images with different bac-terial coverages and biofilm structures, coming from different imaging instrumentations (herein stereomicro-scope and transmission microscope). It exploits a proper succession of basic morphological operations (erosion and dilation) and a horizontal line structuring element, to minimize the impact on size and shape of the even finer bacterial features. It systematically enhances the objects of interest, without histogram stretching and/or undesirable artifacts yielded by common automated methods.The quality of the MEED-procedure is ascertained by segmentation tests which demonstrate its robustness regarding the determination of threshold and convergence of the thresholding algorithm. Extensive validation tests over a rich image database, comparison with the literature and comprehensive discussion of the conceptual background support the superiority of the MEED-procedure over the existing methods and demonstrate it is not a routine application of morphological operators.
更多查看译文
关键词
Biofilm images,MEED-Procedure,Morphological operators,Contrast enhancement,Highly-convergent thresholding,Segmentation tests
AI 理解论文
溯源树
样例
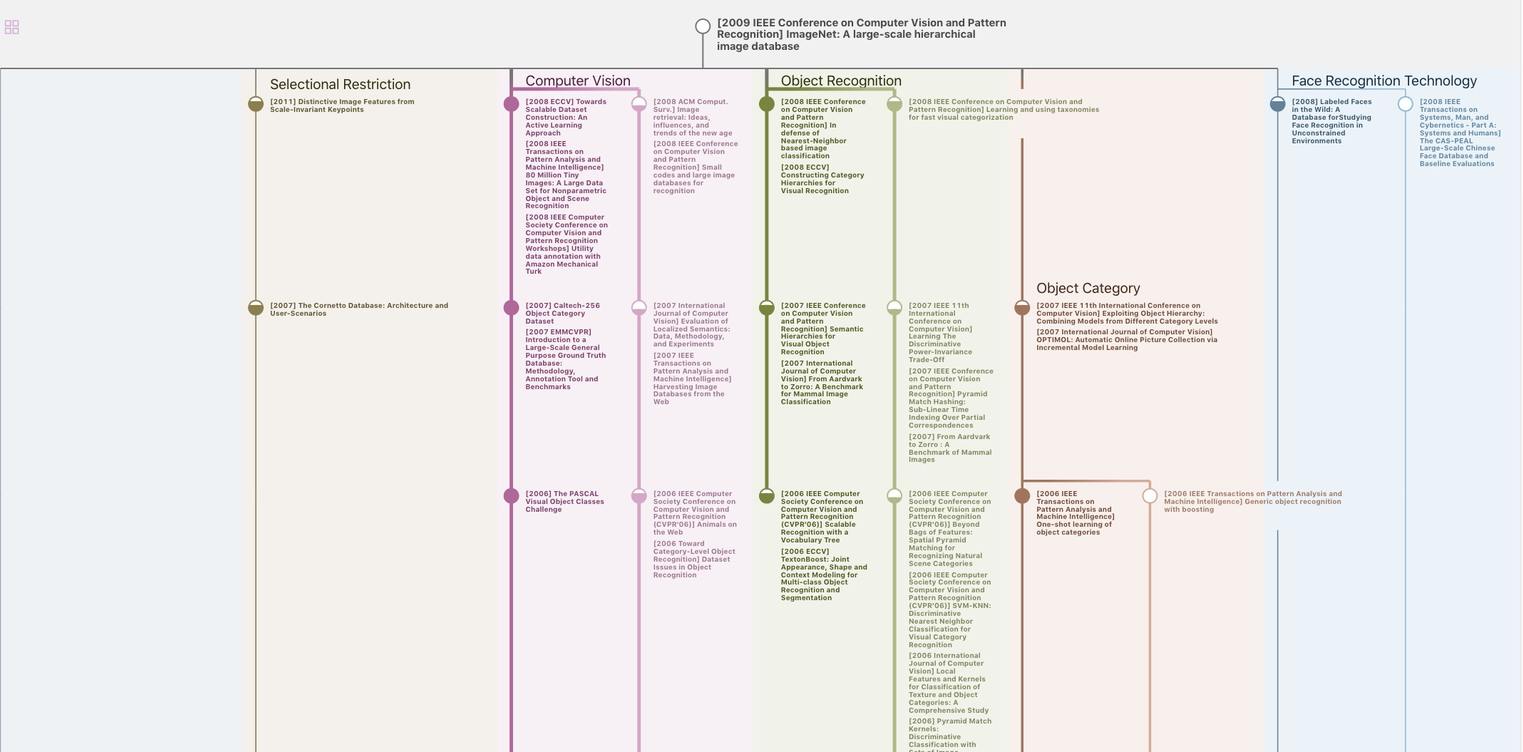
生成溯源树,研究论文发展脉络
Chat Paper
正在生成论文摘要