Rethinking Closed-Loop Training for Autonomous Driving.
European Conference on Computer Vision(2022)
摘要
Recent advances in high-fidelity simulators have enabled closed-loop training of autonomous driving agents, potentially solving the distribution shift in training v.s. deployment and allowing training to be scaled both safely and cheaply. However, there is a lack of understanding of how to build effective training benchmarks for closed-loop training. In this work, we present the first empirical study which analyzes the effects of different training benchmark designs on the success of learning agents, such as how to design traffic scenarios and scale training environments. Furthermore, we show that many popular RL algorithms cannot achieve satisfactory performance in the context of autonomous driving, as they lack long-term planning and take an extremely long time to train. To address these issues, we propose trajectory value learning (TRAVL), an RL-based driving agent that performs planning with multistep look-ahead and exploits cheaply generated imagined data for efficient learning. Our experiments show that TRAVL can learn much faster and produce safer maneuvers compared to all the baselines. For more information, visit the project website: https://waabi.ai/research/travl
更多查看译文
关键词
Closed-loop learning, Autonomous driving, RL
AI 理解论文
溯源树
样例
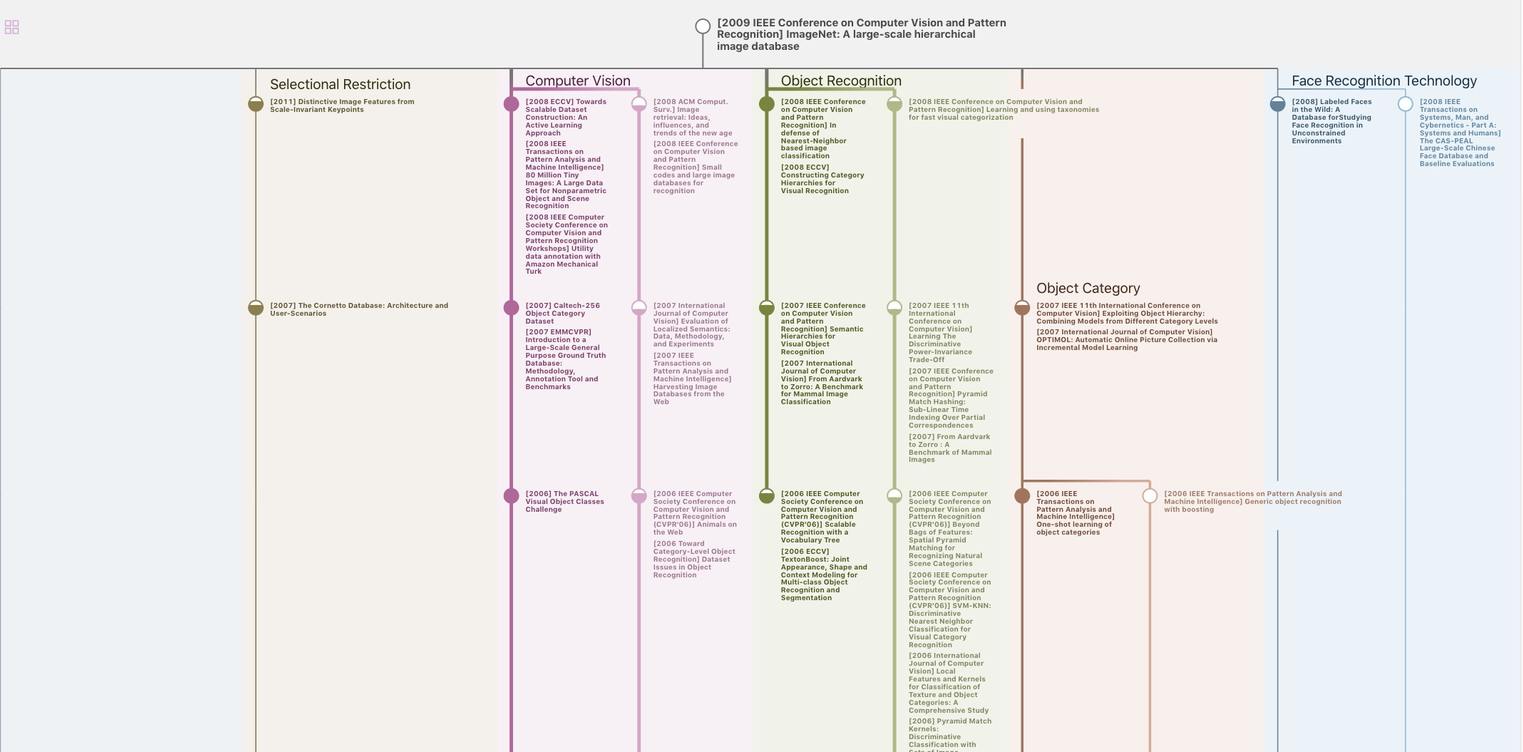
生成溯源树,研究论文发展脉络
Chat Paper
正在生成论文摘要