SpatialDETR: Robust Scalable Transformer-Based 3D Object Detection From Multi-view Camera Images With Global Cross-Sensor Attention.
European Conference on Computer Vision(2022)
摘要
Based on the key idea of DETR this paper introduces an object-centric 3D object detection framework that operates on a limited number of 3D object queries instead of dense bounding box proposals followed by non-maximum suppression. After image feature extraction a decoder-only transformer architecture is trained on a set-based loss. SpatialDETR infers the classification and bounding box estimates based on attention both spatially within each image and across the different views. To fuse the multi-view information in the attention block we introduce a novel geometric positional encoding that incorporates the view ray geometry to explicitly consider the extrinsic and intrinsic camera setup. This way, the spatially-aware cross-view attention exploits arbitrary receptive fields to integrate cross-sensor data and therefore global context. Extensive experiments on the nuScenes benchmark demonstrate the potential of global attention and result in state-of-the-art performance. Code available at https://github.com/cgtuebingen/SpatialDETR.
更多查看译文
关键词
3D object detection, Cross-sensor attention, Autonomous driving
AI 理解论文
溯源树
样例
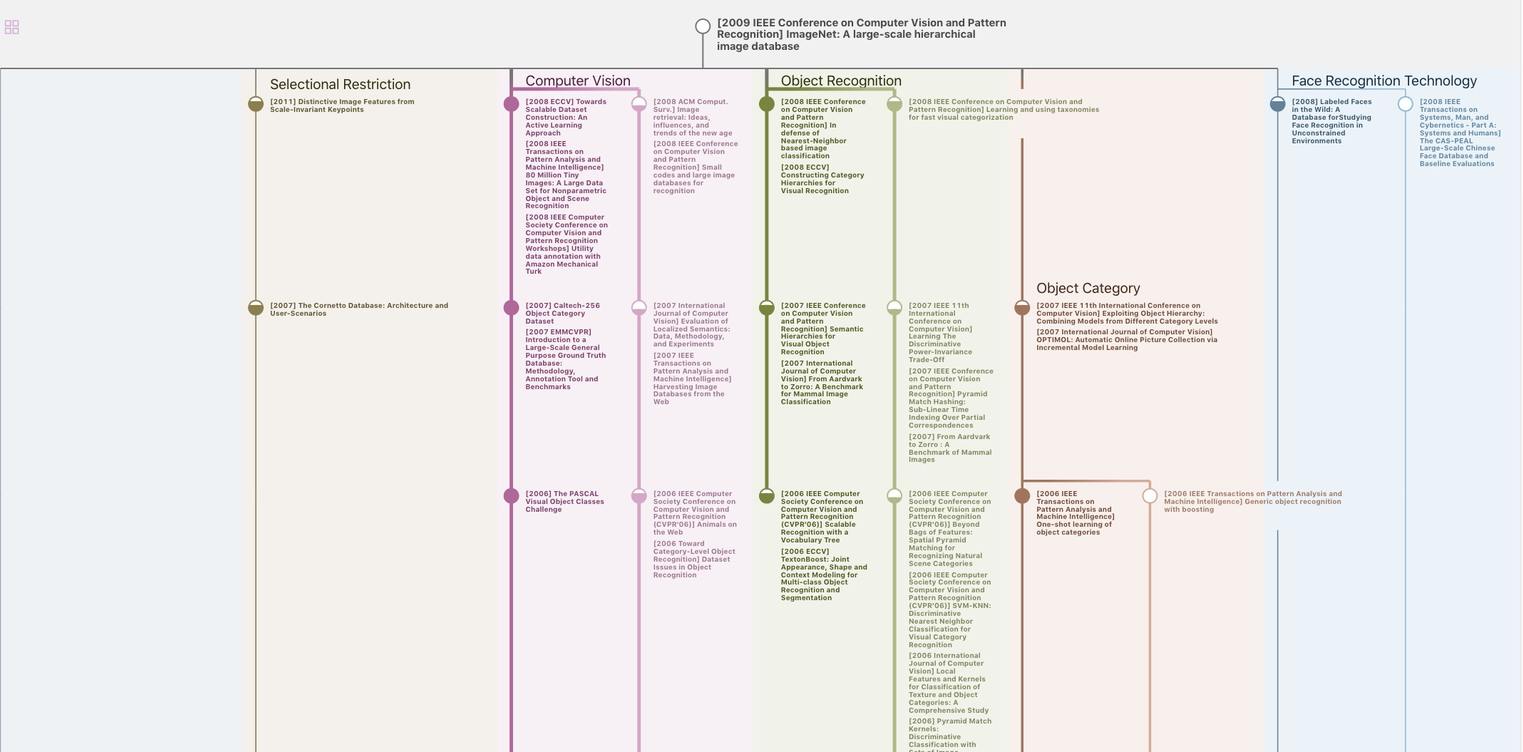
生成溯源树,研究论文发展脉络
Chat Paper
正在生成论文摘要