Dual Contrastive Learning with Anatomical Auxiliary Supervision for Few-Shot Medical Image Segmentation.
European Conference on Computer Vision(2022)
摘要
Few-shot semantic segmentation is a promising solution for scarce data scenarios, especially for medical imaging challenges with limited training data. However, most of the existing few-shot segmentation methods tend to over rely on the images containing target classes, which may hinder its utilization of medical imaging data. In this paper, we present a few-shot segmentation model that employs anatomical auxiliary information from medical images without target classes for dual contrastive learning. The dual contrastive learning module performs comparison among vectors from the perspectives of prototypes and contexts, to enhance the discriminability of learned features and the data utilization. Besides, to distinguish foreground features from background features more friendly, a constrained iterative prediction module is designed to optimize the segmentation of the query image. Experiments on two medical image datasets show that the proposed method achieves performance comparable to state-of-the-art methods. Code is available at: https://github.com/cvszusparkle/AAS-DCL_FSS.
更多查看译文
关键词
Few-shot segmentation, Medical image segmentation, Contrastive learning, Anatomical information
AI 理解论文
溯源树
样例
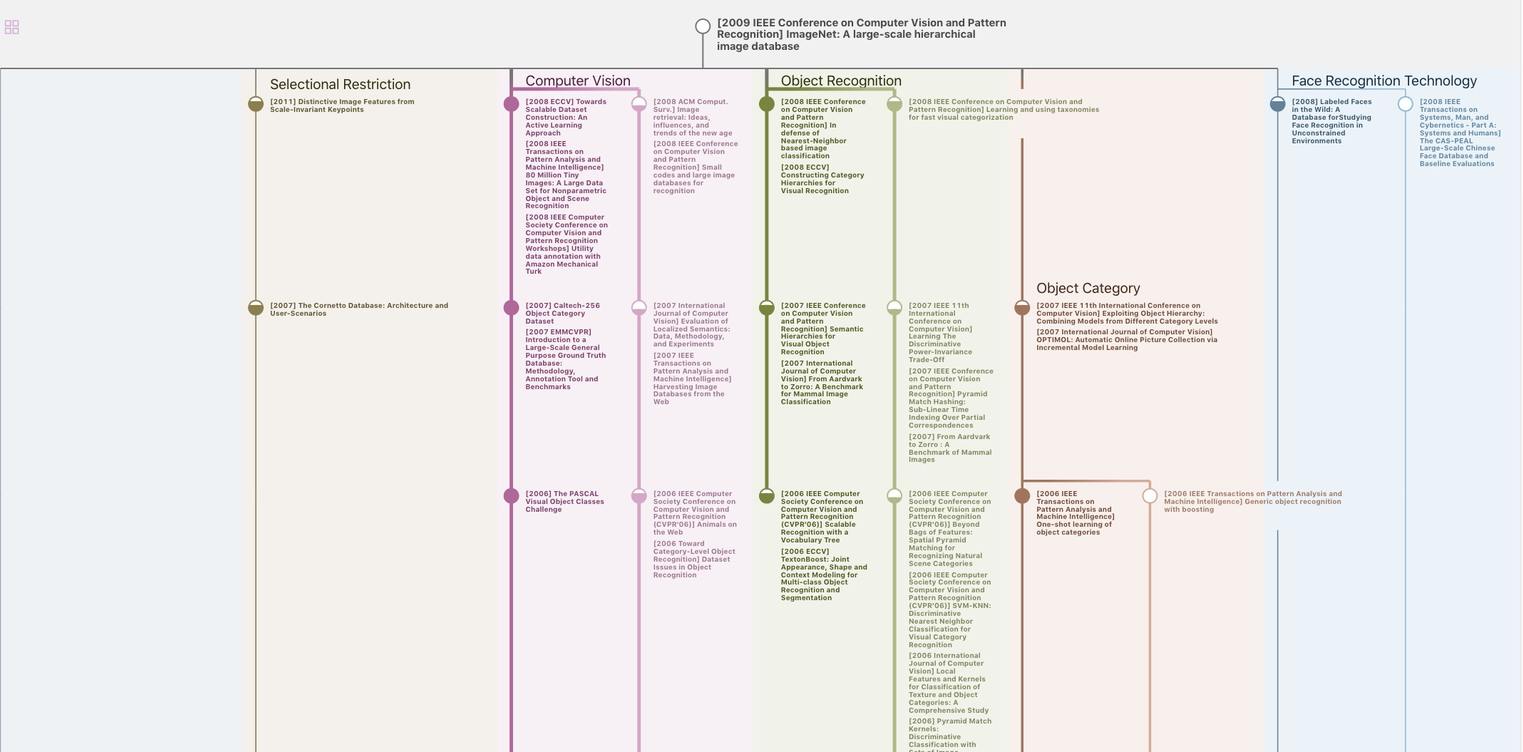
生成溯源树,研究论文发展脉络
Chat Paper
正在生成论文摘要