Cornerformer: Purifying Instances for Corner-Based Detectors.
European Conference on Computer Vision(2022)
Abstract
Corner-based object detectors enjoy the potential of detecting arbitrarily-sized instances, yet the performance is mainly harmed by the accuracy of instance construction. Specifically, there are three factors, namely, 1) the corner keypoints are prone to false-positives; 2) incorrect matches emerge upon corner keypoint pull-push embeddings; and 3) the heuristic NMS cannot adjust the corners pull-push mechanism. Accordingly, this paper presents an elegant framework named Cornerformer that is composed of two factors. First, we build a Corner Transformer Encoder (CTE, a self-attention module) in a 2D-form to enhance the information aggregated by corner keypoints, offering stronger features for the pull-push loss to distinguish instances from each other. Second, we design an Attenuation-Auto-Adjusted NMS (A3-NMS) to maximally leverage the semantic outputs and avoid true objects from being removed. Experiments on object detection and human pose estimation show the superior performance of Cornerformer in terms of accuracy and inference speed.
MoreTranslated text
Key words
Object detection,Corner-based,Corner Transformer Encoder,Attenuation-Auto-Adjusted NMS
AI Read Science
Must-Reading Tree
Example
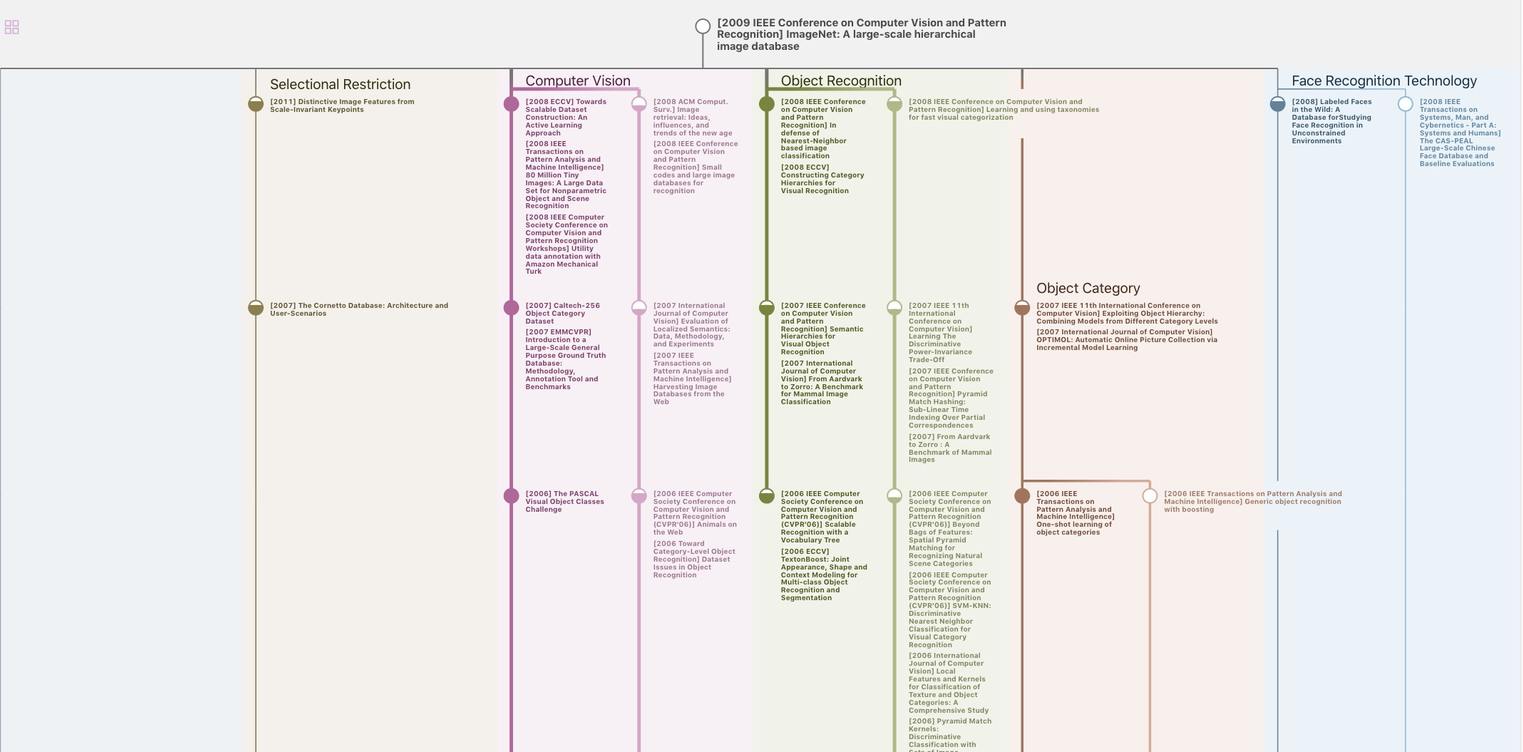
Generate MRT to find the research sequence of this paper
Chat Paper
Summary is being generated by the instructions you defined