Active Label Correction Using Robust Parameter Update and Entropy Propagation.
European Conference on Computer Vision(2022)
摘要
Label noise is prevalent in real-world visual learning applications and correcting all label mistakes can be prohibitively costly. Training neural network classifiers on such noisy datasets may lead to significant performance degeneration. Active label correction (ALC) attempts to minimize the re-labeling costs by identifying examples for which providing correct labels will yield maximal performance improvements. Existing ALC approaches typically select the examples that the classifier is least confident about (e.g. with the largest entropies). However, such confidence estimates can be unreliable as the classifier itself is initially trained on noisy data. Also, naively selecting a batch of low confidence examples can result in redundant labeling of spatially adjacent examples. We present a new ALC algorithm that addresses these challenges. Our algorithm robustly estimates label confidence values by regulating the contributions of individual examples in the parameter update of the network. Further, our algorithm avoids redundant labeling by promoting diversity in batch selection through propagating the confidence of each newly labeled example to the entire dataset. Experiments involving four benchmark datasets and two types of label noise demonstrate that our algorithm offers a significant improvement in re-labeling efficiency over state-of-the-art ALC approaches.
更多查看译文
关键词
Active label correction, Uncertainty sampling, Diffusion
AI 理解论文
溯源树
样例
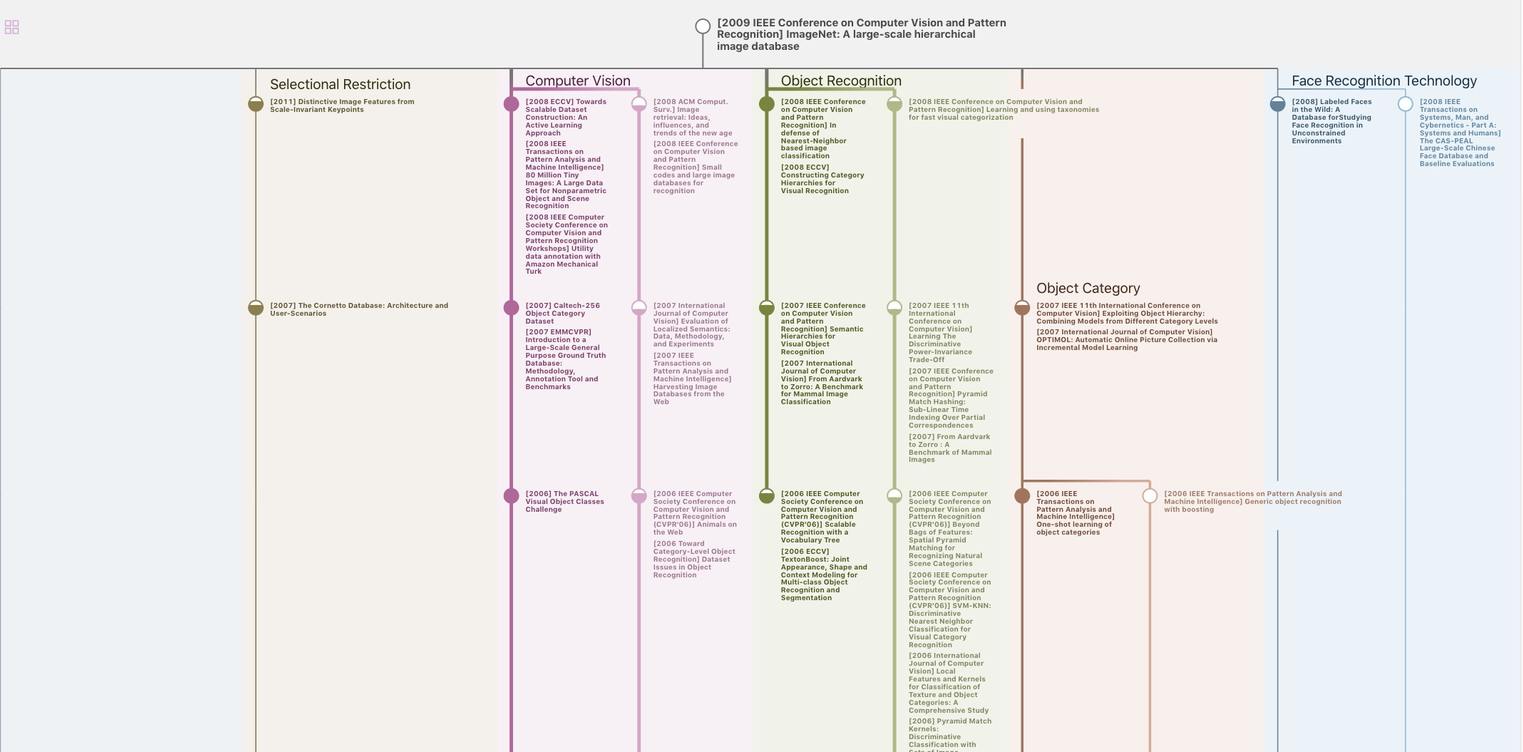
生成溯源树,研究论文发展脉络
Chat Paper
正在生成论文摘要