Self-supervised Sparse Representation for Video Anomaly Detection.
European Conference on Computer Vision(2022)
摘要
Video anomaly detection (VAD) aims at localizing unexpected actions or activities in a video sequence. Existing mainstream VAD techniques are based on either the one-class formulation, which assumes all training data are normal, or weakly-supervised, which requires only video-level normal/anomaly labels. To establish a unified approach to solving the two VAD settings, we introduce a self-supervised sparse representation (S3R) framework that models the concept of anomaly at feature level by exploring the synergy between dictionary-based representation and self-supervised learning. With the learned dictionary, S3R facilitates two coupled modules, en-Normal and de-Normal, to reconstruct snippet-level features and filter out normal-event features. The self-supervised techniques also enable generating samples of pseudo normal/anomaly to train the anomaly detector. We demonstrate with extensive experiments that S3R achieves new state-of-the-art performances on popular benchmark datasets for both one-class and weakly-supervised VAD tasks. Our code is publicly available at https://github.com/louisYen/S3R.
更多查看译文
关键词
Sparse representation,Video anomaly detection
AI 理解论文
溯源树
样例
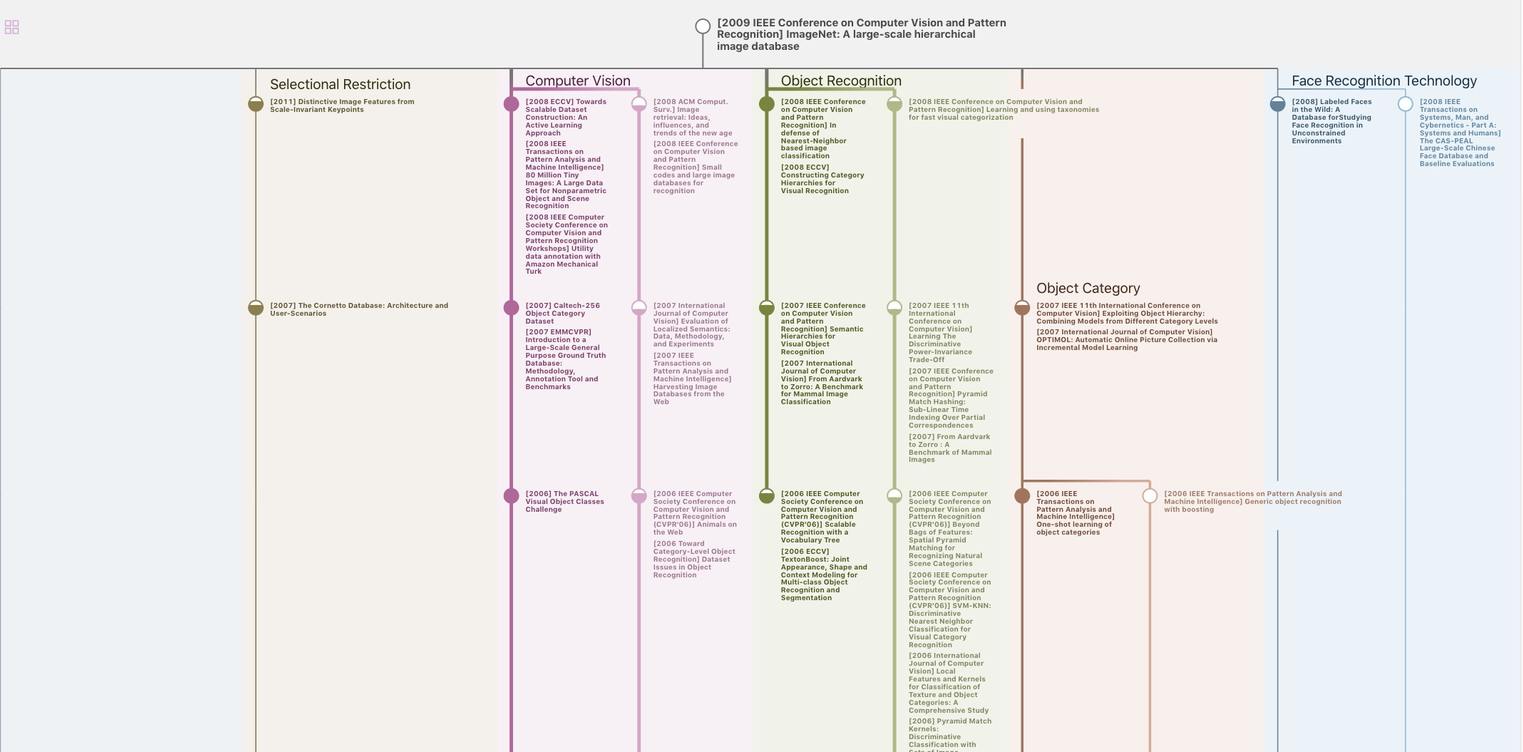
生成溯源树,研究论文发展脉络
Chat Paper
正在生成论文摘要