Adaptive Cross-domain Learning for Generalizable Person Re-identification.
European Conference on Computer Vision(2022)
摘要
Domain Generalizable Person Re-Identification (DG-ReID) is a more practical ReID task that is trained from multiple source domains and tested on the unseen target domains. Most existing methods are challenged for dealing with the shared and specific characteristics among different domains, which is called the domain conflict problem. To address this problem, we present an Adaptive Cross-domain Learning (ACL) framework equipped with a CrOss-Domain Embedding Block (CODE-Block) to maintain a common feature space for capturing both the domain-invariant and the domain-specific features, while dynamically mining the relations across different domains. Moreover, our model adaptively adjusts the architecture to focus on learning the corresponding features of a single domain at a time without interference from the biased features of other domains. Specifically, the CODE-Block is composed of two complementary branches, a dynamic branch for extracting domain-adaptive features and a static branch for extracting the domain-invariant features. Extensive experiments demonstrate that the proposed approach achieves state-of-the-art performances on the popular benchmarks. Under Protocol-2, our method outperforms previous SOTA by 7.8% and 7.6% in terms of mAP and rank-1 accuracy.
更多查看译文
关键词
Adaptive cross-domain learning, Common feature space, Dynamic network, Domain conflict, Domain generalizable person, re-identification
AI 理解论文
溯源树
样例
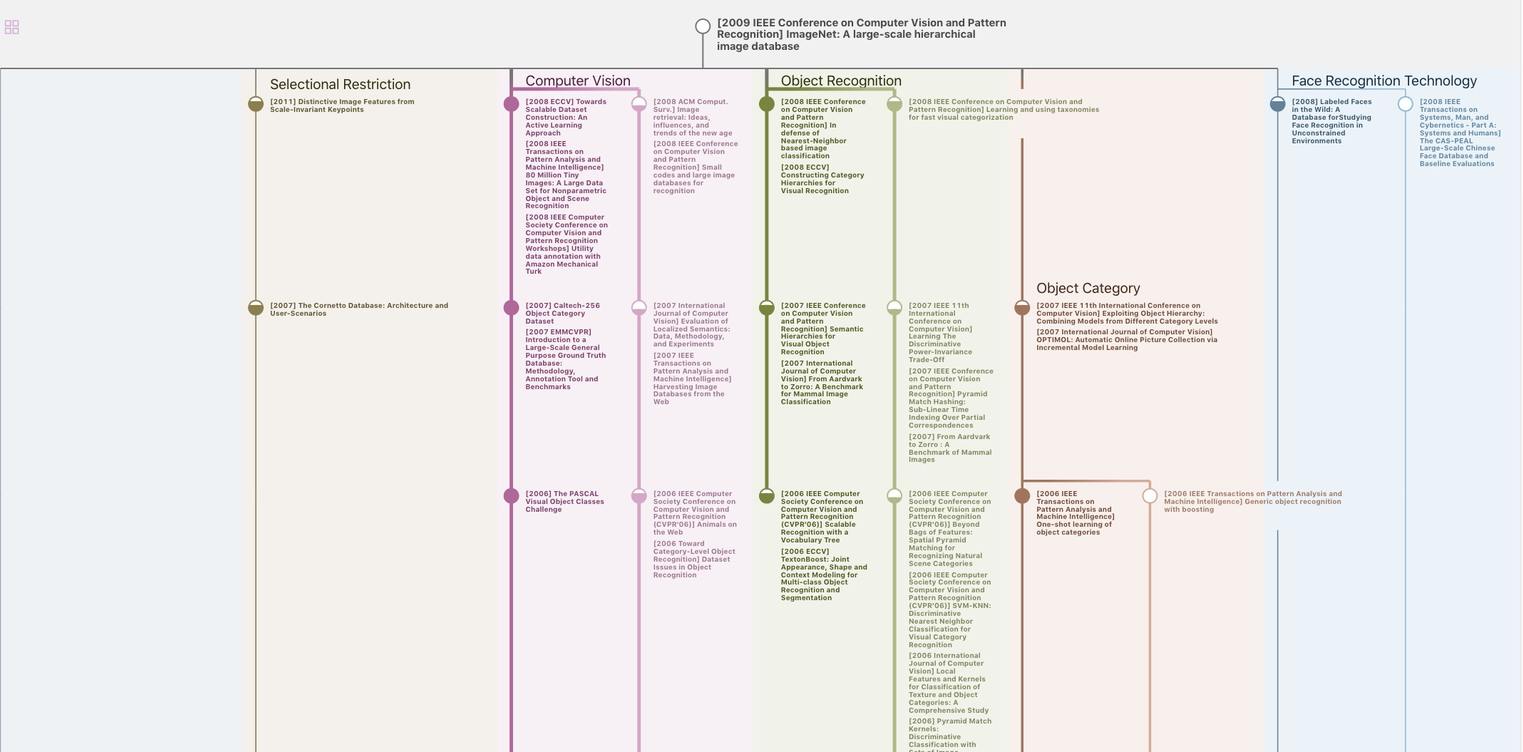
生成溯源树,研究论文发展脉络
Chat Paper
正在生成论文摘要