Similarity-Guided and Multi-Layer Fusion Network for Few-shot Semantic Segmentation
2022 3rd International Conference on Information Science, Parallel and Distributed Systems (ISPDS)(2022)
摘要
Few-shot semantic segmentation aims to tackle the problem that segmenting unseen object class using only a few support images with the same object class. At present, most related methods focus on prototype learning or feature similarity. However, these few-shot segmentation methods do not make good use of high-level features to enhance the prediction results. In this paper, we propose a lightweight Similarity-Guided and Multi-layer Fusion Network (SMNet) with two modules including Similarity-Guided Module (SGM) and Multi-Layer Fusion Module (MLFM). Specifically, the SGM utilizes cosine similarities in multiple high-level feature layers to augment the features in middle-level from query and support image, and then augmented features are refined via a residual attention module. In order to enhance the diversity of features, we reformulate the refined features as a spatiotemporal sequence problem. Then, we introduce the MLFM, which combines two ConvLSTMs to obtain fused feature from different scales. Finally, the decoder takes fused features to obtain predicted mask. Experiment results demonstrate that our model can achieve superior or competitive performances in several datasets.
更多查看译文
关键词
component,Semantic Segmentation,Few-shot Segme ntation,Few-shot Learning
AI 理解论文
溯源树
样例
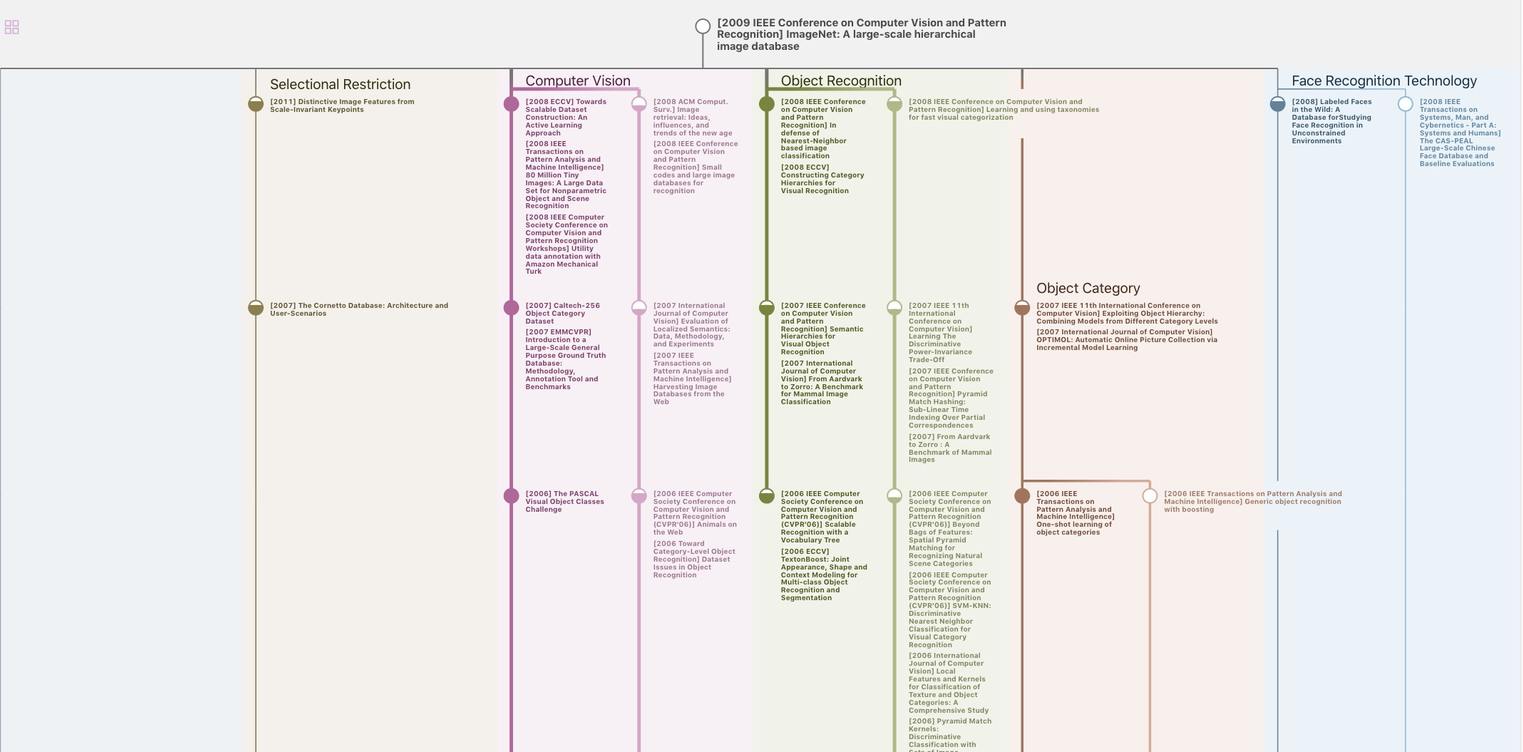
生成溯源树,研究论文发展脉络
Chat Paper
正在生成论文摘要