Information-Theoretic Characterizations of Generalization Error for the Gibbs Algorithm
IEEE TRANSACTIONS ON INFORMATION THEORY(2024)
摘要
Various approaches have been developed to upper bound the generalization error of a supervised learning algorithm. However, existing bounds are often loose and even vacuous when evaluated in practice. As a result, they may fail to characterize the exact generalization ability of a learning algorithm. Our main contributions are exact characterizations of the expected generalization error of the well-known Gibbs algorithm (a.k.a. Gibbs posterior) using different information measures, in particular, the symmetrized KL information between the input training samples and the output hypothesis. Our result can be applied to tighten existing expected generalization errors and PAC-Bayesian bounds. Our information-theoretic approach is versatile, as it also characterizes the generalization error of the Gibbs algorithm with a data-dependent regularizer and that of the Gibbs algorithm in the asymptotic regime, where it converges to the standard empirical risk minimization algorithm. Of particular relevance, our results highlight the role the symmetrized KL information plays in controlling the generalization error of the Gibbs algorithm.
更多查看译文
关键词
Empirical risk minimization,generalization error,Gibbs algorithm,PAC-Bayesian learning,symmetrized KL information
AI 理解论文
溯源树
样例
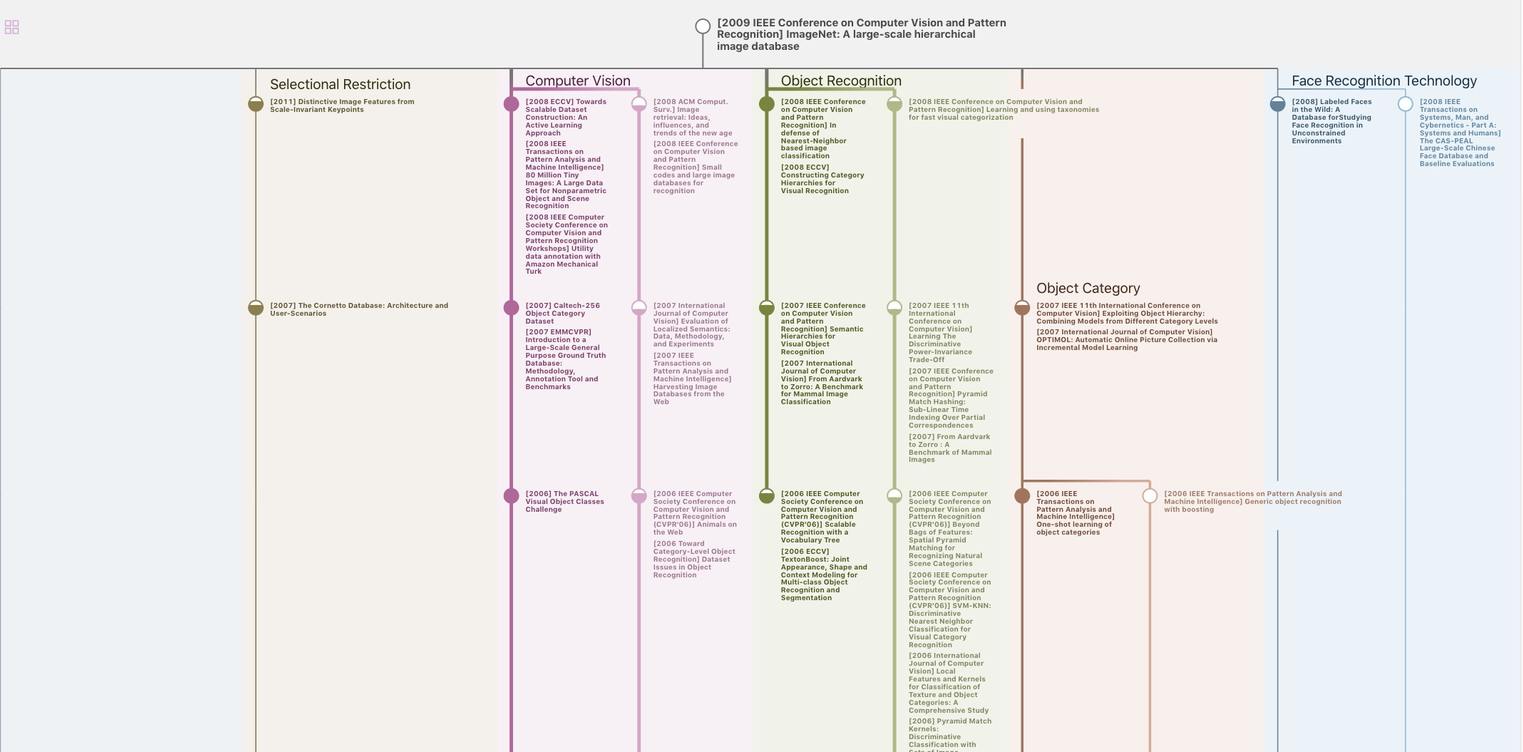
生成溯源树,研究论文发展脉络
Chat Paper
正在生成论文摘要