An Incremental Input-to-State Stability Condition for a Class of Recurrent Neural Networks
IEEE TRANSACTIONS ON AUTOMATIC CONTROL(2024)
摘要
This article proposes a novel sufficient condition for the incremental input-to-state stability of a class of recurrent neural networks (RNNs). The established condition is compared with others available in the literature, showing to be less conservative. Moreover, it can be applied for the design of incremental input-to-state stable RNN-based control systems, resulting in a linear matrix inequality constraint for some specific RNN architectures. The formulation of nonlinear observers for the considered system class, as well as the design of control schemes with explicit integral action, are also investigated. The theoretical results are validated through simulation on a referenced nonlinear system.
更多查看译文
关键词
Control systems,Stability criteria,Recurrent neural networks,Asymptotic stability,Artificial neural networks,Symmetric matrices,Feedforward systems,Linear matrix inequalities,neural networks (NNs),nonlinear control systems,stability of nonlinear systems
AI 理解论文
溯源树
样例
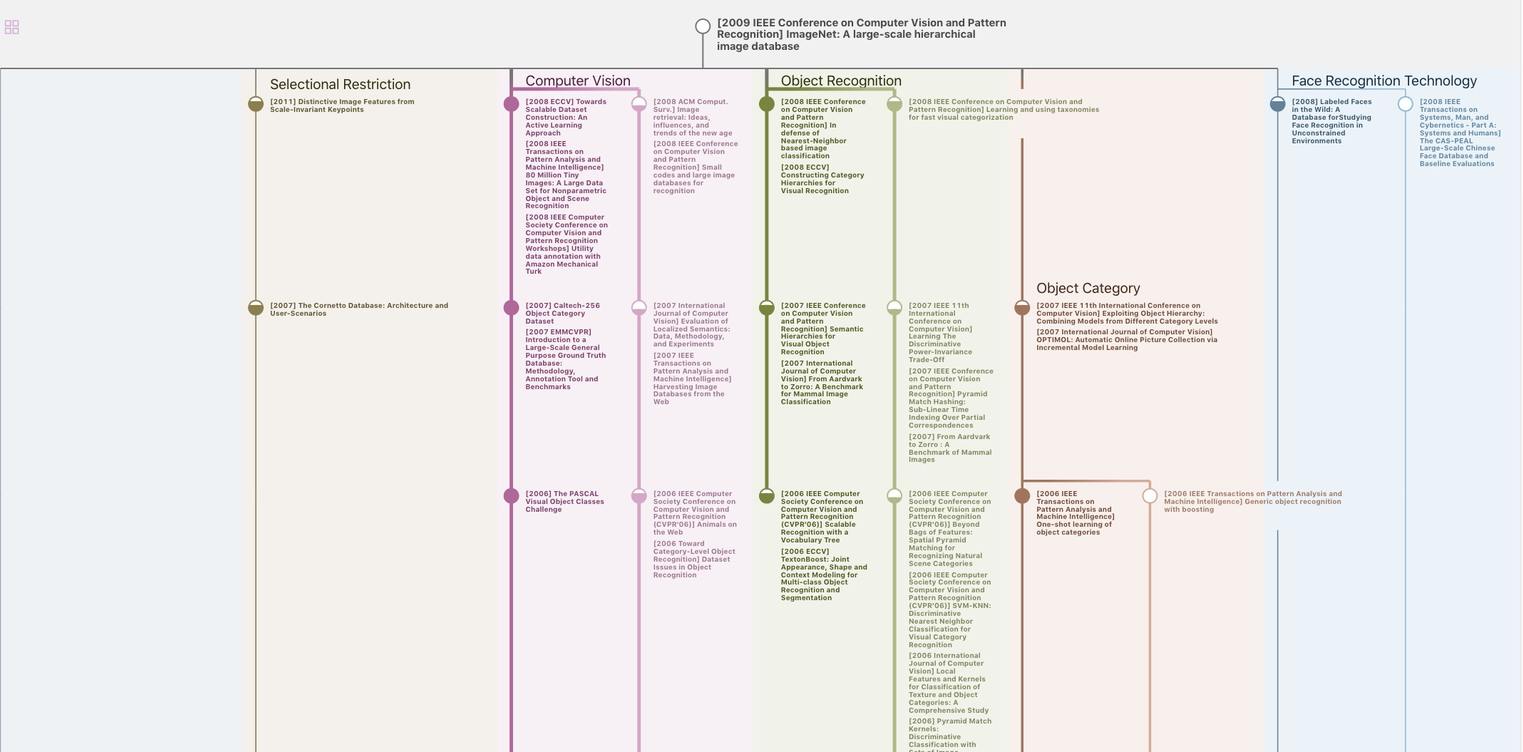
生成溯源树,研究论文发展脉络
Chat Paper
正在生成论文摘要