Use of Classification Algorithms to Predict the Grade of Geomagnetic Disturbance
Advances in Neural Computation, Machine Learning, and Cognitive Research VI(2022)
摘要
This paper presents different approaches for predicting the grade of geomagnetic Kp index using machine learning algorithms. The Kp index is considered to be an indicator of the energy input from the solar wind into the Earth’s magnetosphere. In this study, a wide range of machine learning algorithms were tested for the purpose of classifying Kp index grade, such as gradient boosting models, linear models, and neural networks. The main challenge of this classification task is a strong class imbalance, due to the fact that extreme values of Kp index are rarely observed. To overcome the issue, the SMOTE technique for minority classes oversampling was utilized. It is shown that SMOTE improves quality of the classification at far horizons. We also test time-series cross-validation for hyperparameters optimization and show that it does not improve the quality. All the models are scored against an out-of-sample test set to assess their quality and compare the results. Finally, we highlight the directions of further research based on the results obtained in this study.
更多查看译文
关键词
Kp geomagnetic index, Geomagnetic disturbance, Classification, Class imbalance, SMOTE, Time-series cross-validation, Neural networks, Gradient boosting, Random forest, Ridge regression
AI 理解论文
溯源树
样例
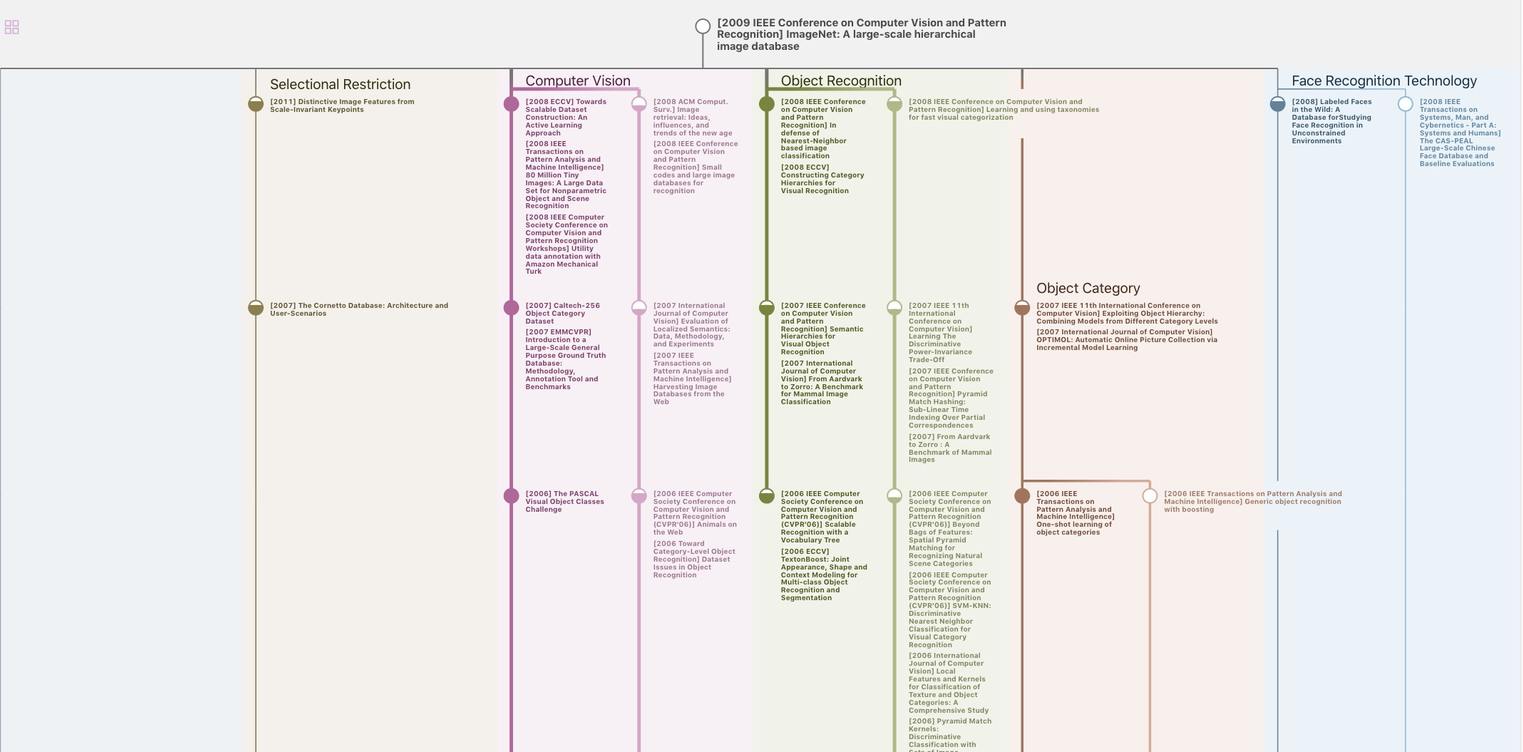
生成溯源树,研究论文发展脉络
Chat Paper
正在生成论文摘要