Towards Reliable Solar Atmospheric Parameters Neural-Based Inference
Advances in Neural Computation, Machine Learning, and Cognitive Research VI(2022)
摘要
Methods of Stokes profile inversion based on spectral polarization analysis represent a powerful tool for obtaining information on magnetic and thermodynamic properties in the solar atmosphere. However, these methods involve solving the radiation transport equation. Over the past decades, several approaches have been developed to provide an analytical solution to the inverse problem, but despite its advantages, in many cases it requires large computing resources. Neural networks have been shown to be a good alternative to these methods, but in general they tend to be overly confident in their predictions. In this paper, the uncertainty estimation of atmospheric parameters prediction is presented. It is shown that deterministic networks containing partially-independent MLP blocks allow one to estimate uncertainty in predictions achieving the high accuracy results.
更多查看译文
关键词
Magnetic fields, Inverse problem, Spectral lines, Deep learning, Multi-task learning
AI 理解论文
溯源树
样例
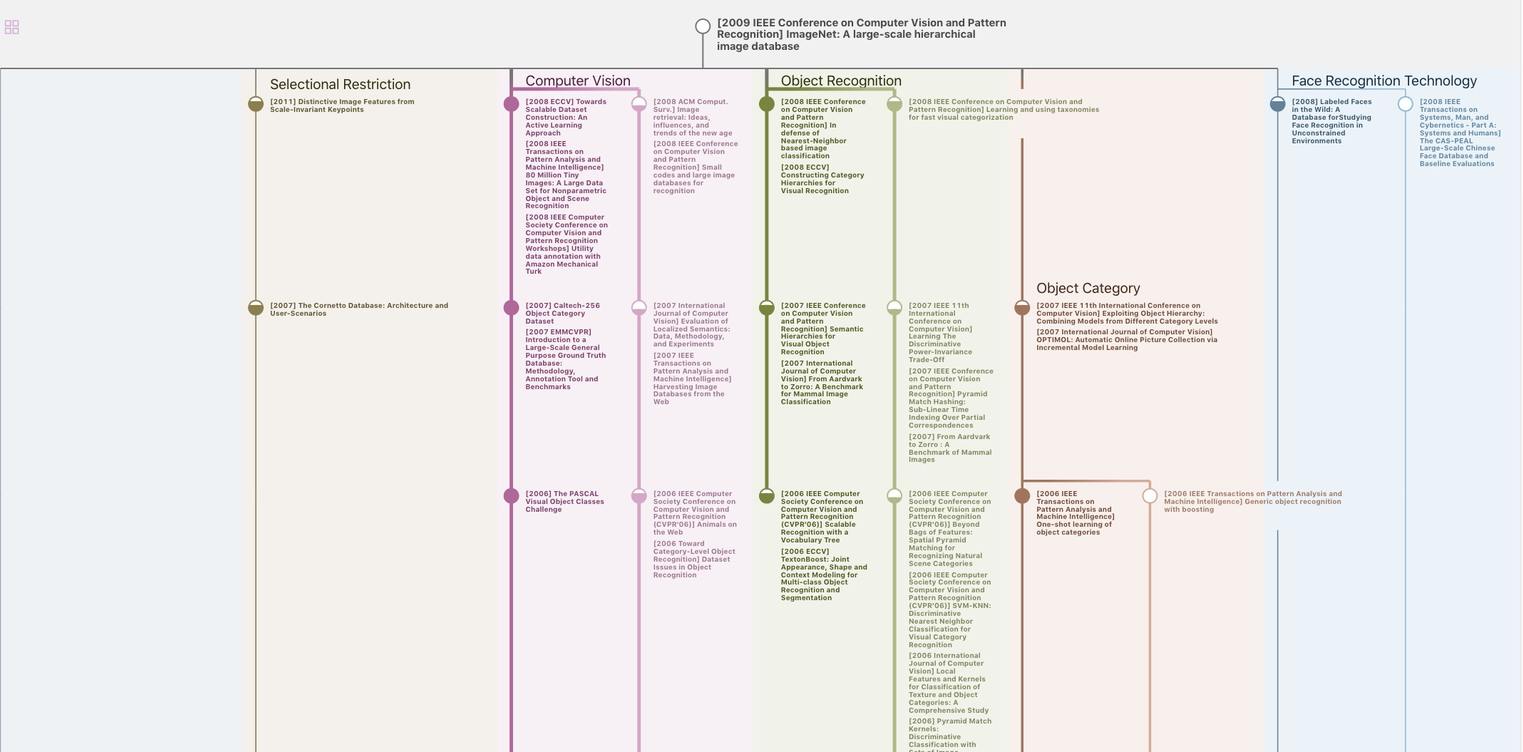
生成溯源树,研究论文发展脉络
Chat Paper
正在生成论文摘要