Multi-scale convolutional networks for traffic forecasting with spatial-temporal attention.
Pattern Recognit. Lett.(2022)
摘要
Traffic forecasting is a classical spatial-temporal data prediction problem with extensive applications, such as dynamic traffic management, route planning and traffic congestion alleviation. However, accurate traf-fic forecasting is challenging due to complex correlations of traffic data. Few approaches are satisfied with both long-term and short-term prediction tasks. In this paper, we propose a novel Multi-Scale Convolu-tional Networks (MSCN), an end-to-end solution to solve traffic forecasting problem. MSCN first employs an encoder with spatial-temporal attention mechanism to model both spatial and temporal correlations. Then, the decoder utilizes multiple convolutions and form groups of units to extract spatial-temporal fea-tures for different resolutions. In particular, we propose a soft thresholding fusion mechanism to adap-tively adjust the conditions of spatial and temporal correlations. Experiments on two real traffic datasets demonstrate that the proposed MSCN obtains improvements over the state-of-the-art baselines.(c) 2022 Elsevier B.V. All rights reserved.
更多查看译文
关键词
Traffic forecasting,Spatial -temporal attention,Convolutional networks
AI 理解论文
溯源树
样例
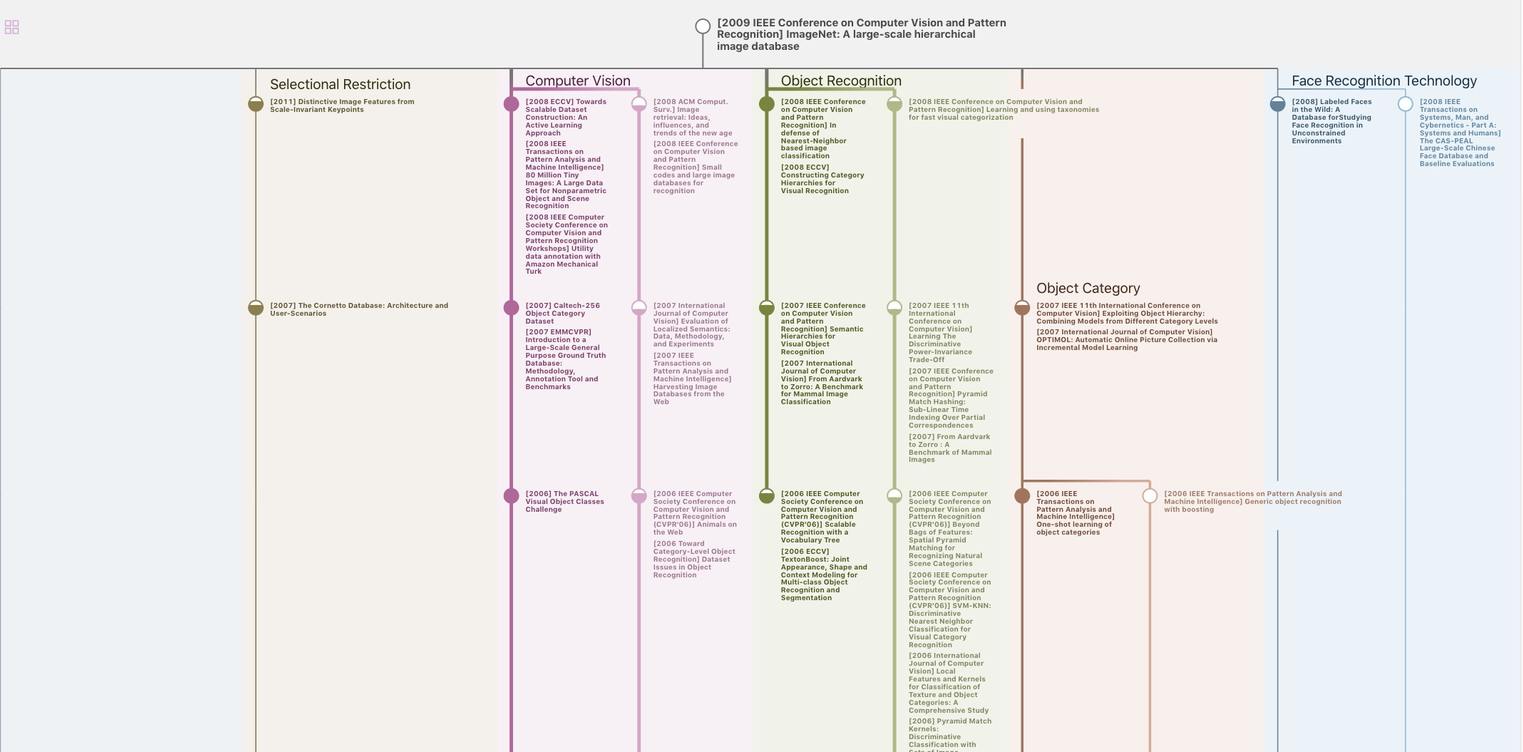
生成溯源树,研究论文发展脉络
Chat Paper
正在生成论文摘要