Using tissue-energy response to generate virtual monoenergetic images from conventional CT for computer-aided diagnosis of lesions
7th International Conference on Image Formation in X-Ray Computed Tomography(2022)
摘要
Based on the X-ray physics in computed tomography (CT) imaging, the linear attenuation coefficient (LAC) of each human tissue is described as a function of the X-ray photon energy. Different tissue types (i.e. muscle, fat, bone, and lung tissue) have their energy responses and bring more tissue contrast distribution information along the energy axis, which we call tissue-energy response (TER). In this study, we propose to use TER to generate virtual monoenergetic images (VMIs) from conventional CT for computer-aided diagnosis (CADx) of lesions. Specifically, for a conventional CT image, each tissue fraction can be identified by the TER curve at the effective energy of the setting tube voltage. Based on this, a series of VMIs can be generated by the tissue fractions multiplying the corresponding TER. Moreover, a machine learning (ML) model is developed to exploit the energy-enhanced tissue material features for differentiating malignant from benign lesions, which is based on the data-driven deep learning (DL)-CNN method. Experimental results demonstrated that the DL-CADx models with the proposed method can achieve better classification performance than the conventional CT-based CADx method from three sets of pathologically proven lesion datasets.
更多查看译文
关键词
virtual monoenergetic images,conventional ct,tissue-energy,computer-aided
AI 理解论文
溯源树
样例
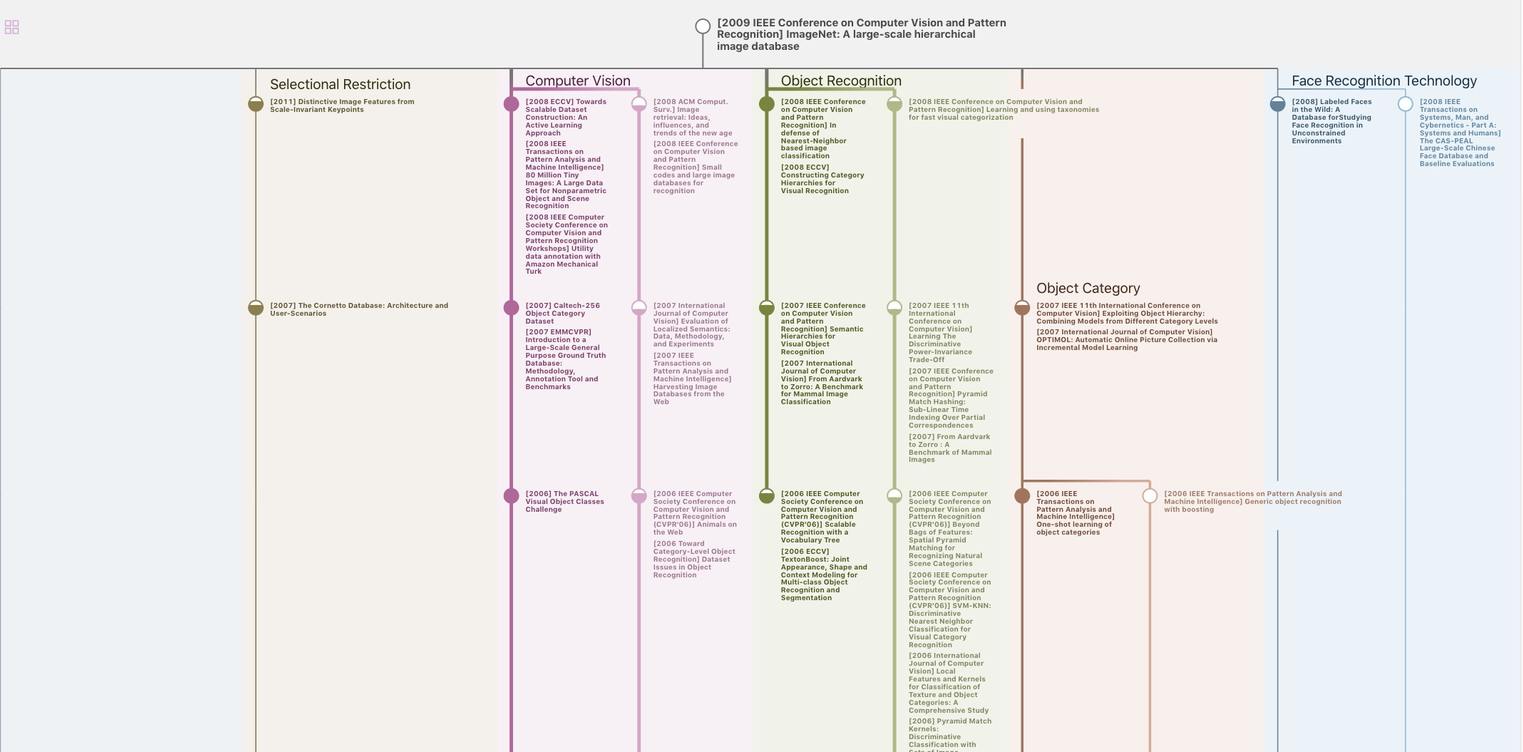
生成溯源树,研究论文发展脉络
Chat Paper
正在生成论文摘要