A probabilistic framework for uncertainty quantification in positron emission particle tracking
arxiv(2023)
摘要
Positron Emission Particle Tracking (PEPT) is an imaging method for the visualization of fluid motion, capable of reconstructing three-dimensional trajectories of small tracer particles suspended in nearly any medium, including fluids that are opaque or contained within opaque vessels. The particles are labeled radioactively, and their positions are reconstructed from the detection of pairs of back-to-back photons emitted by positron annihilation. Current reconstruction algorithms are heuristic and typically based on minimizing the distance between the particles and the so-called lines of response (LoRs) joining the detection points, while accounting for spurious LoRs generated by scattering. Here we develop a probabilistic framework for the Bayesian inference and uncertainty quantification of particle positions from PEPT data. We formulate a likelihood by describing the emission of photons and their noisy detection as a Poisson process in the space of LoRs. We derive formulas for the corresponding Poisson rate in the case of cylindrical detectors, accounting for both undetected and scattered photons. We illustrate the formulation by quantifying the uncertainty in the reconstruction of the position of a single particle on a circular path from data generated by state-of-the-art Monte Carlo simulations. The results show how the observation time $\Delta t$ can be chosen optimally to balance the need for a large number of LoRs with the requirement of small particle displacement imposed by the assumption that the particle is static over $\Delta t$. We further show how this assumption can be relaxed by inferring jointly the position and velocity of the particle, with clear benefits for the accuracy of the reconstruction.
更多查看译文
关键词
uncertainty quantification,probabilistic framework,particle
AI 理解论文
溯源树
样例
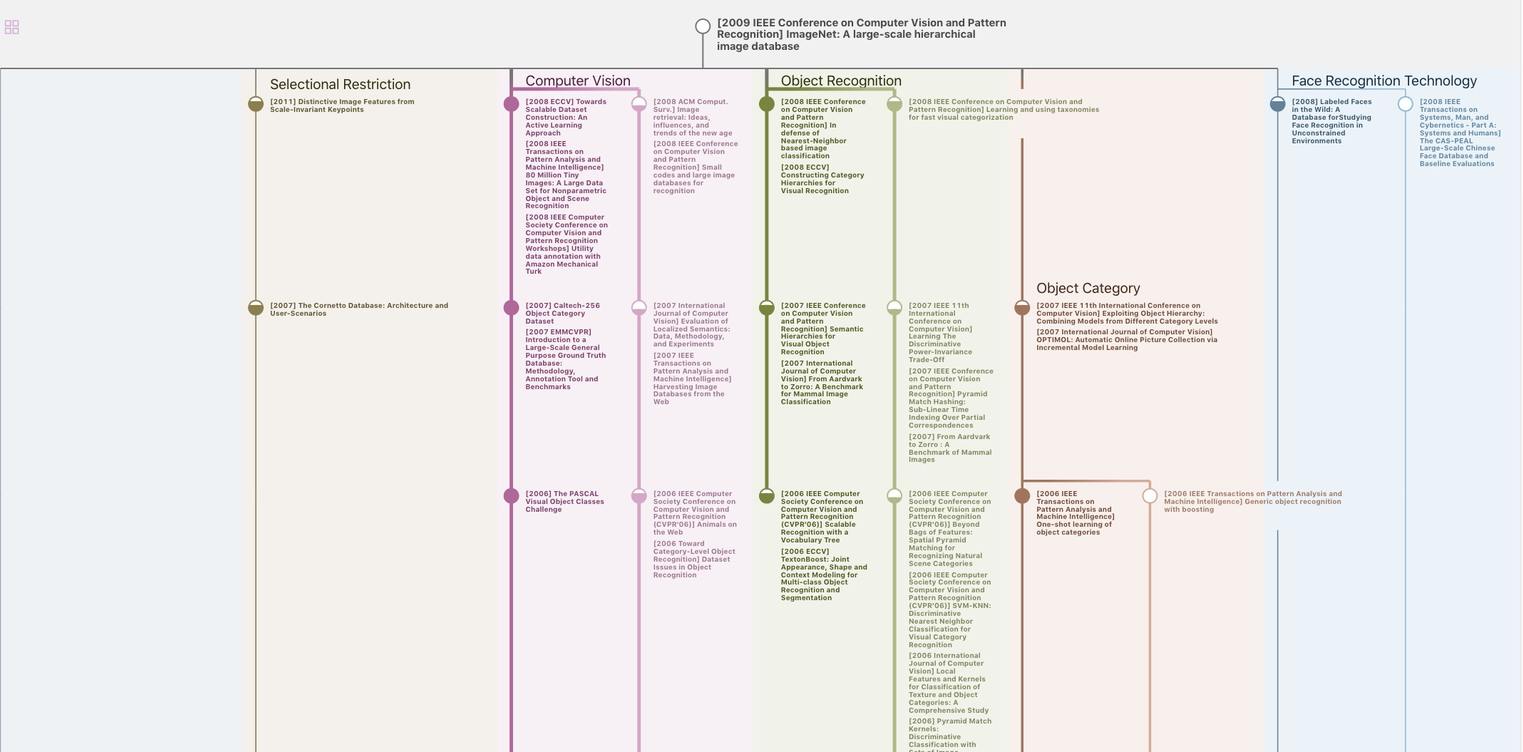
生成溯源树,研究论文发展脉络
Chat Paper
正在生成论文摘要