A Transformer-based Generative Model for De Novo Molecular Design
CoRR(2022)
摘要
In the scope of drug discovery, the molecular design aims to identify novel compounds from the chemical space where the potential drug-like molecules are estimated to be in the order of 10^60 - 10^100. Since this search task is computationally intractable due to the unbounded search space, deep learning draws a lot of attention as a new way of generating unseen molecules. As we seek compounds with specific target proteins, we propose a Transformer-based deep model for de novo target-specific molecular design. The proposed method is capable of generating both drug-like compounds (without specified targets) and target-specific compounds. The latter are generated by enforcing different keys and values of the multi-head attention for each target. In this way, we allow the generation of SMILES strings to be conditional on the specified target. Experimental results demonstrate that our method is capable of generating both valid drug-like compounds and target-specific compounds. Moreover, the sampled compounds from conditional model largely occupy the real target-specific molecules' chemical space and also cover a significant fraction of novel compounds.
更多查看译文
关键词
generative model,transformer-based
AI 理解论文
溯源树
样例
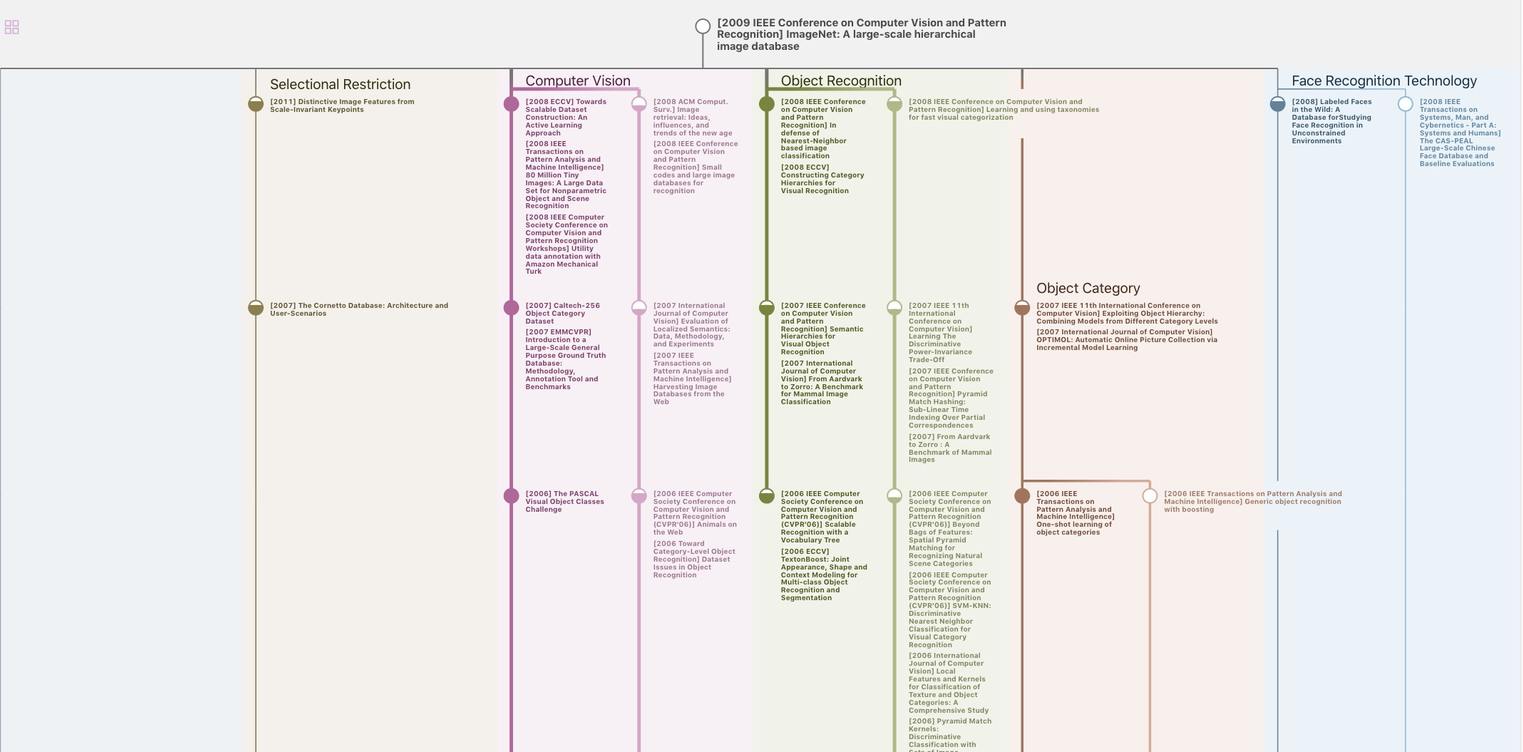
生成溯源树,研究论文发展脉络
Chat Paper
正在生成论文摘要