Generative modeling and reinforcement learning for acoustic lens design
Metamaterials, Metadevices, and Metasystems 2022(2022)
摘要
This research develops data-driven methods for metamaterial design using generative modeling and reinforcement learning (RL). Previously, both generative modeling and RL2 showed exciting results for acoustic cloak design. We want to generalize both frameworks for acoustic lens design. The proposed 2D-Global Optimization Networks (2D-GLOnets) maximize the root mean square (RMS) of the absolute pressure at the focal point at discrete wavenumber values to enable acoustic lens design. The 2D-GLOnets(1) are adapted with a reparametrization technique that constrains the scatterers' positions into a feasible region. The pressure amplitude can converge to optimal values faster because of the gradients computed analytically from a multiple scattering solver. % The loss function with respect to the weights is utilized to update the generator's weights. In addition, Deep Deterministic Policy Gradient (DDPG) algorithm is applied to the acoustic lens design. DDPG controls the positions of the cylinders and assigns rewards based on the absolute RMS pressure amplitude at the focal point. The reward function assigns a higher value to the state of absolute pressure amplitude. As the agent iteratively completes episodes, the reward is maximized. The agent searches for the configuration of the scatterers that produce the enhanced focusing effect. The numerical results are presented for both models considering uniform configurations of scatterers with a varying number of scatterers and wavenumbers.
更多查看译文
关键词
Acoustic Metamaterials,Inverse Design,Machine Learning,Generative Modeling,2D-GLOnets,Deep Reinforcement Learning,DDPG
AI 理解论文
溯源树
样例
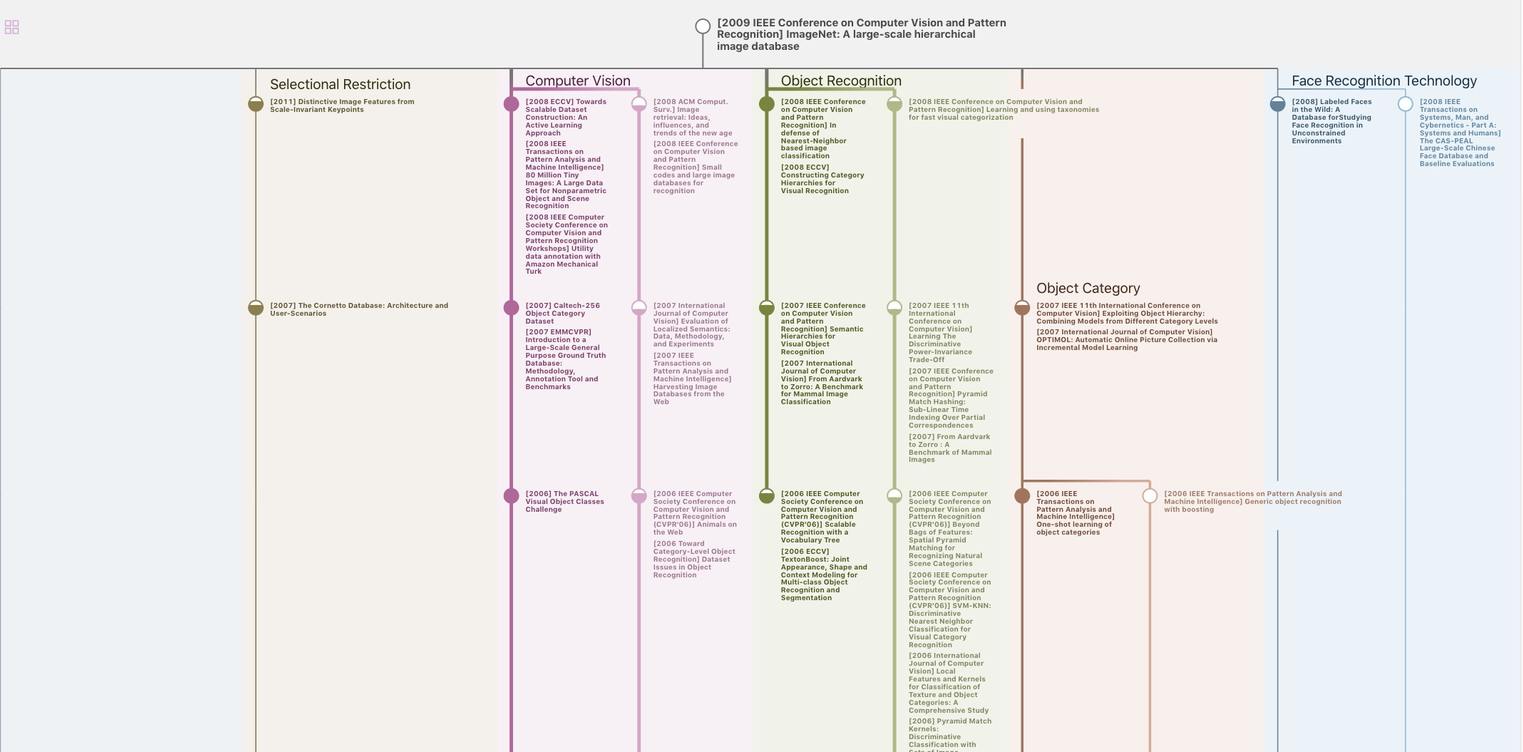
生成溯源树,研究论文发展脉络
Chat Paper
正在生成论文摘要