Variable Stiffness Actuator Control based on Linear Model Predictive Control in a Low Stiffness Regime
ISR Europe 2022; 54th International Symposium on Robotics(2022)
摘要
Humans perform dexterous and highly dynamic movements like throwing, running, hitting or jumping by adjusting the dynamic characteristics of their musculoskeletal system. While the human muscle stiffness varies across different tasks, adjustable stiffness ensures the safety, saves energy, and provides comfort of to the human while maintaining performance. Roboticists try to integrate similar capabilities into robotic manipulators by using dedicated actuators such as Variable Stiffness Actuators (VSAs). VSAs integrate elastic elements with either fixed or variable mechanical compliance to resemble human muscles. The usage of such compliance elements also allows to actively control the stiffness. However, the full potential of such actuation has currently not been realized because it is notoriously difficult to control VSAs, specifically in the low-stiffness regime which, however, is particularly safe and also most suited to store energy for highly dynamic motions release. In this paper, we therefore consider control of an VSA manufactured by qbrobotics. The qbmove VSA consists of two motors which connect to the output shaft by nonlinear elastic elements such that both motors contribute torques symmetrically to produce shaft rotation, while antagonistic motor actuation regulates the stiffness. We realize and evaluate to this aim conventional PID control, Sliding Mode Control (SMC) and Model Predictive Control (MPC). Based on a dynamical model of the VSA their performance is evaluated for tracking a sinusoidal and a square wave. To apply linear MPC, an approximate linear VSA model is created through system identification of the actual hardware and likewise applied. The results show superior performance of the MPC as compared to the standard PID baseline and Sliding Mode Control.
更多查看译文
AI 理解论文
溯源树
样例
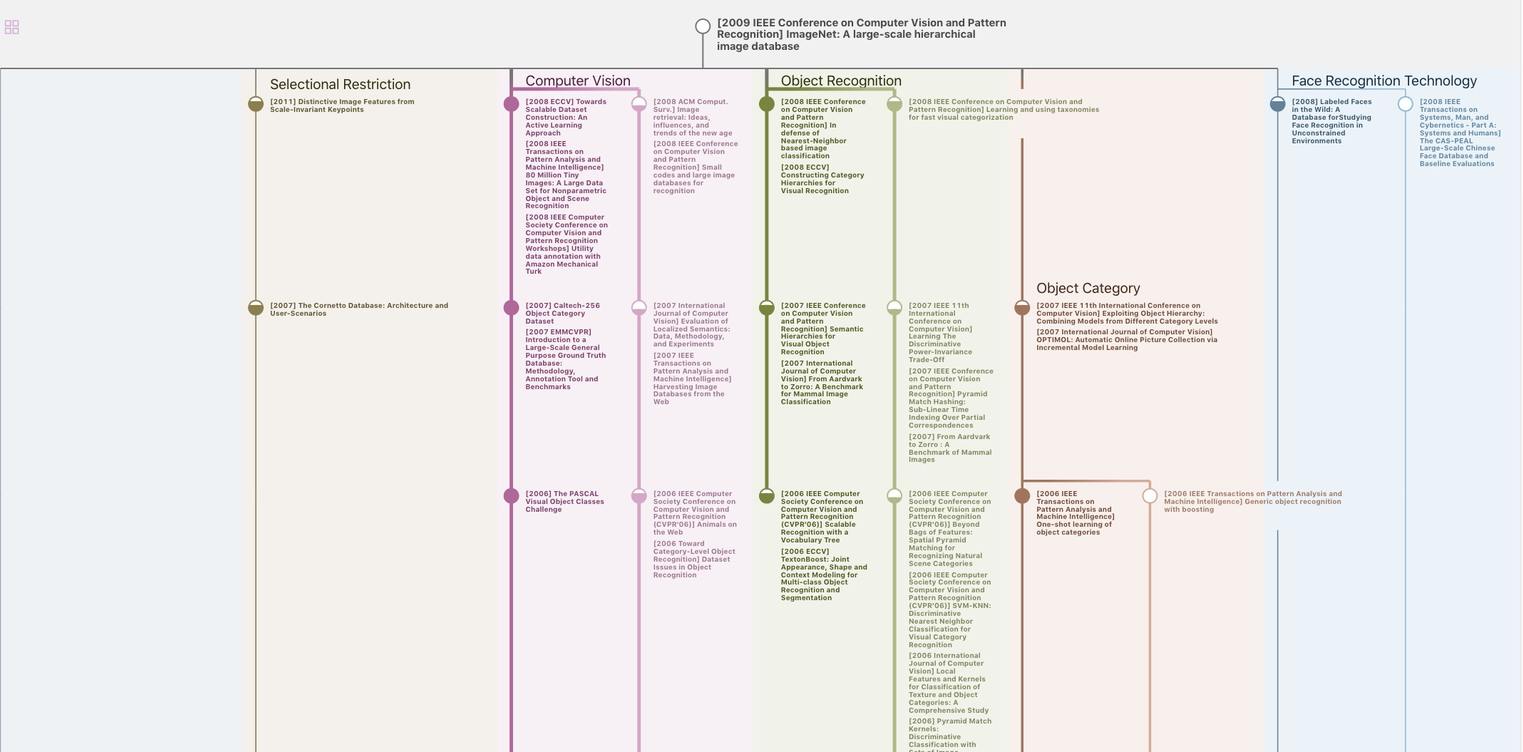
生成溯源树,研究论文发展脉络
Chat Paper
正在生成论文摘要