Feature Enhanced Graph Neural Network for Few-Shot Image Classification
2022 IEEE 25th International Conference on Computer Supported Cooperative Work in Design (CSCWD)(2022)
摘要
There has been a rising trend of solving few-shot image classification problems utilizing graph neural networks (GNN) in recent years. However, most GNN-based approaches fail to fully exploit relationships among samples, resulting in poor image classification. To address this problem, we propose a feature-enhanced GNN model appropriate for few-shot image classification tasks. The suggested method first exploits an efficient convolutional block to generate accurate feature maps, enhancing the expressivity of the feature extraction module. Then, our technique employs flexible combinatorial distance metric functions to compute the exact relation score between samples and determine image relevance by minimizing the matching cost. Moreover, a multilayer perceptron based on the residual structure with an attention mechanism is developed to produce focused feature representations, allowing the model to obtain selective and relevant information among the samples. The proposed model is evaluated on a supervised few-shot image classification task utilizing four benchmark datasets, with the corresponding results demonstrating that our model achieves a higher accuracy performance than traditional few-shot image classification methods.
更多查看译文
关键词
attention mechanism,feature enhancement,few-shot learning,graph neural network,image classification
AI 理解论文
溯源树
样例
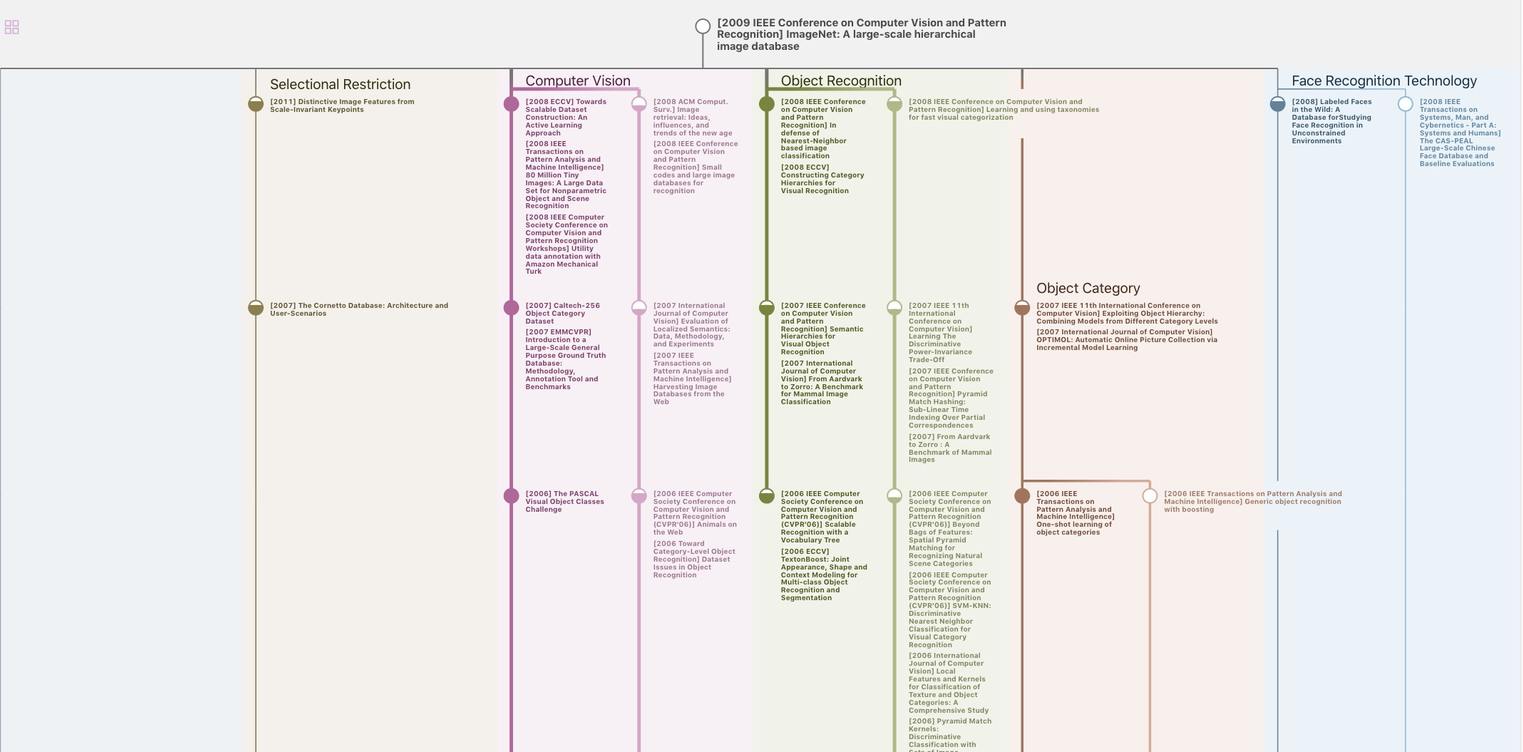
生成溯源树,研究论文发展脉络
Chat Paper
正在生成论文摘要