The Hyperbolic Temporal Attention Based Differentiable Neural Turing Machines for Diachronic Graph Embedding in Cyber Threat Intelligence
International Conference on Computer Supported Cooperative Work in Design (CSCWD)(2022)
摘要
Cyber Threat Intelligence (CTI) is an effective approach to solve cyber security problems, finding unknown threats is becoming a problem to be solved. Research based on threat intelligence knowledge graphs has gradually increased for its capability to capture entity characteristics and better predict unknown threats. Most of the current research focuses on Euclidean space, however, the Euclidean space is insufficient to capture the hierarchical information of the knowledge graph. In this paper, we propose a novel Hyperbolic Temporal Attention based Differential Neural Turing Machines for diachronic graph embedding framework (HTA-DNTM), which adopts a graph attention network model based on hyperbolic space, simultaneously uses multi-head self-attention to map the temporal graph into hyperbolic space and incorporates hyperbolic graph neural network and hyperbolic gated recurrent neural network, capturing the evolving behaviors and implicitly preserve hierarchical information simultaneously. Moreover, we demonstrate significantly improved performance over various approaches on CTI. A series of benchmark experiments illustrate HTA-DNTM has ability to generate higher quality than state-of-the-art word embedding models in CTI fields.
更多查看译文
关键词
Cyber Threat Intelligence,diachronic graph embedding,hyperbolic,temporal attention,knowledge graph
AI 理解论文
溯源树
样例
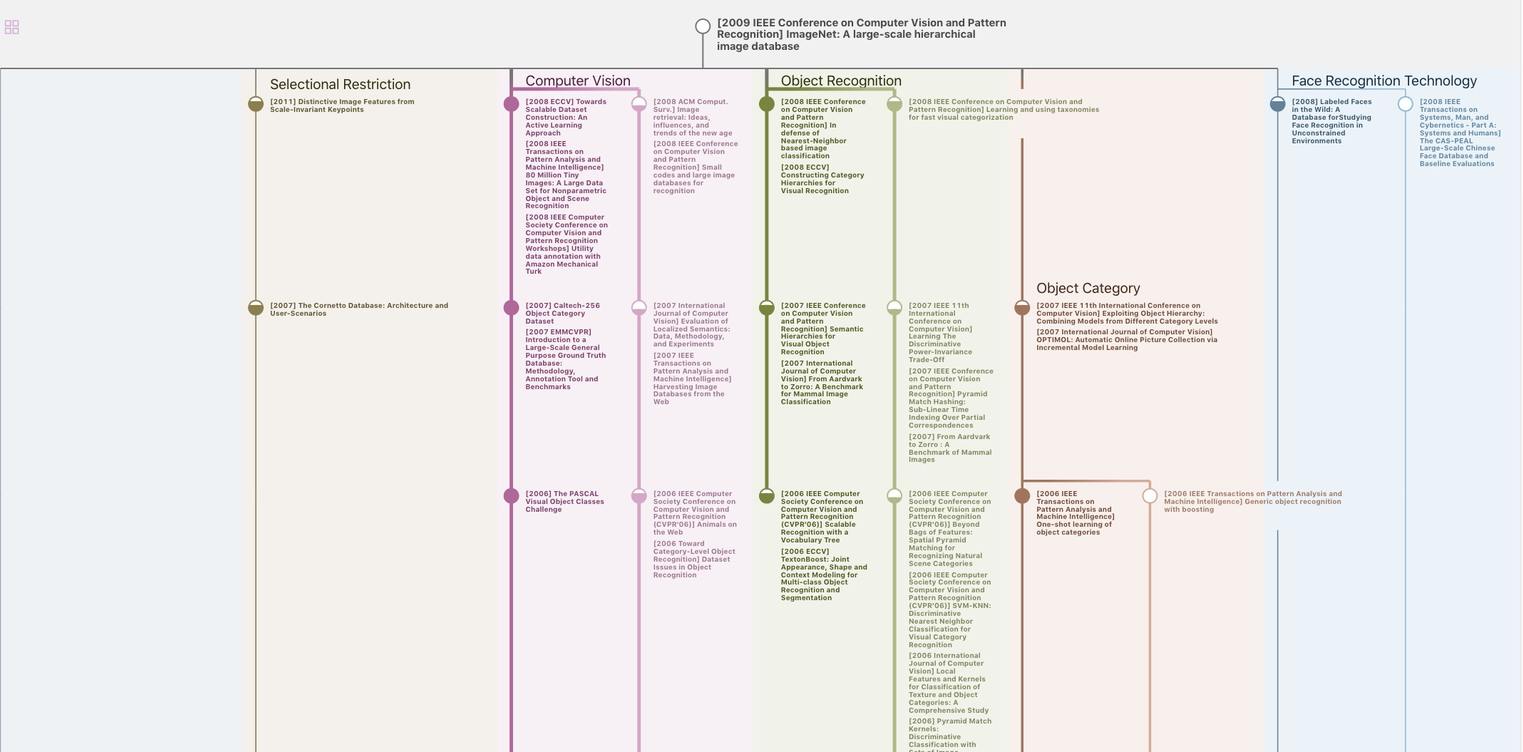
生成溯源树,研究论文发展脉络
Chat Paper
正在生成论文摘要