Grokking-like effects in counterfactual inference
IEEE International Joint Conference on Neural Network (IJCNN)(2022)
摘要
We show that a typical neural network, which ignores any covariate/feature re-balancing, can be as effective as any explicit counterfactual method. We adopt the architecture of TARNet-a simple neural network with two heads (one for treatment, one for control) which is trained with a relatively high batch size. Combined with ensemble methods, this produces competitive results in four counterfactual inference benchmarks: IHDP, NEWS, JOBS, and TWINS. Our results indicate that relatively simple methods might be good enough for counterfactual prediction, with quality constraints coming from hyperparameter tuning. Our analysis indicates that the reason behind the observed phenomenon might be "grokking", a recently developed theory.
更多查看译文
关键词
neural network,ensemble methods,counterfactual inference,grokking,TARNet,covariate-feature re-balancing,IHDP benchmark,NEWS benchmark,JOBS benchmark,TWINS benchmark
AI 理解论文
溯源树
样例
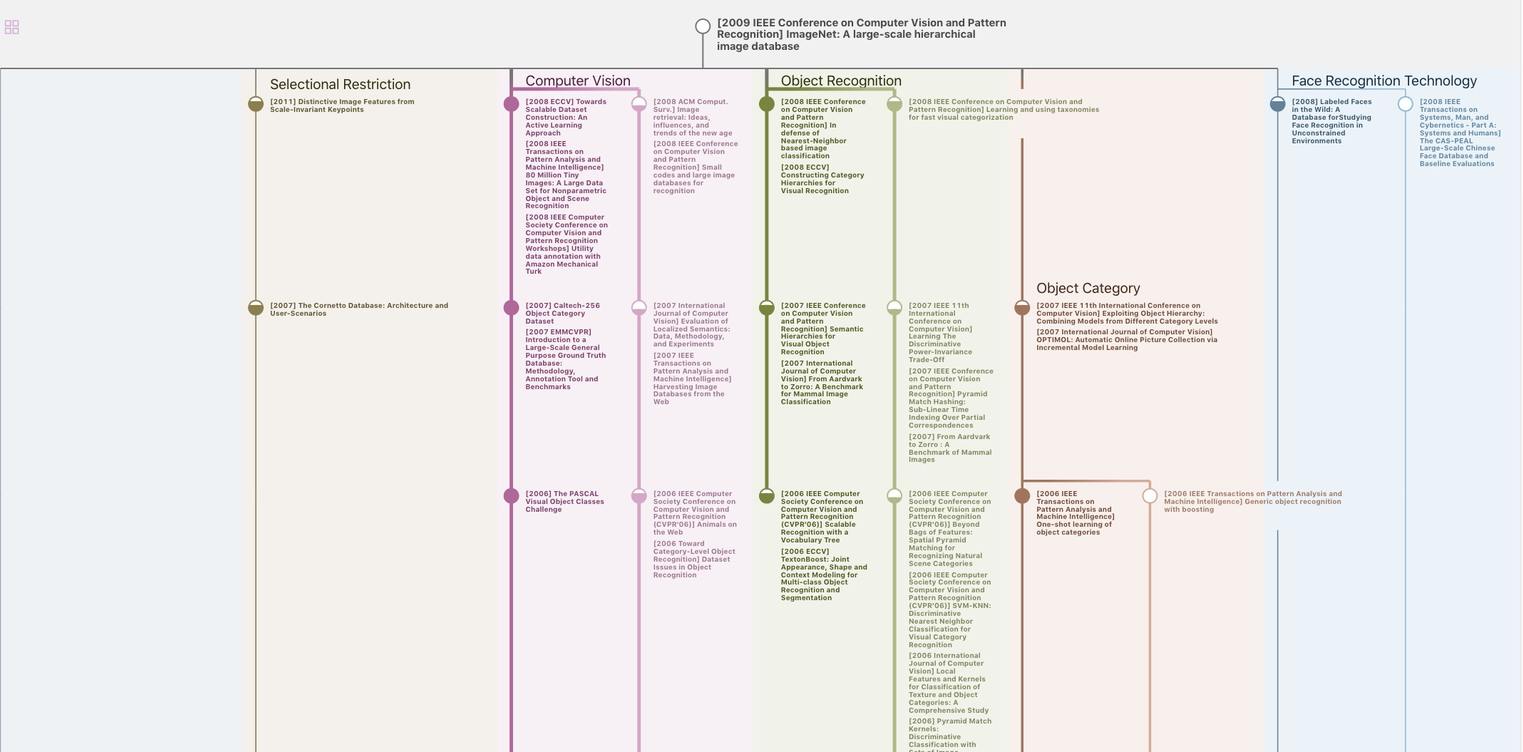
生成溯源树,研究论文发展脉络
Chat Paper
正在生成论文摘要