Contrastive Co-training for Diversified Recommendation
IEEE International Joint Conference on Neural Network (IJCNN)(2022)
摘要
Beyond accuracy, diversity has become a crucial factor to evaluate a recommendation system as higher diversity helps mitigate echo chamber issue and improve user satisfaction. Recently, great success has been made to improve diversity, but the approaches often sacrifice much lower accuracy. Herein this work, we propose contrastive co-training for diversified recommendation that improves diversity greatly and achieves comparable or even better accuracy. Specifically, we keep two user-item graph views for recommendation and contrastive learning, respectively. Pseudo edges are predicted from current graph view to augment the other graph view by mining the novel items that users might be highly interested in. However, merely leveraging co-training hurts the accuracy since the pseudo labels are sometimes noisy. Therefore, we propose diversified contrastive learning that not only is robust to the noisy pseudo edges but also improves the diversity further by alleviating the popularity and category biases by re-balancing item-level popularity and category-level advantage. The extensive experiments on three public datasets show the superiority of our proposed model in terms of accuracy and diversity compared with strong baselines.
更多查看译文
关键词
diversified recommendation,co-training
AI 理解论文
溯源树
样例
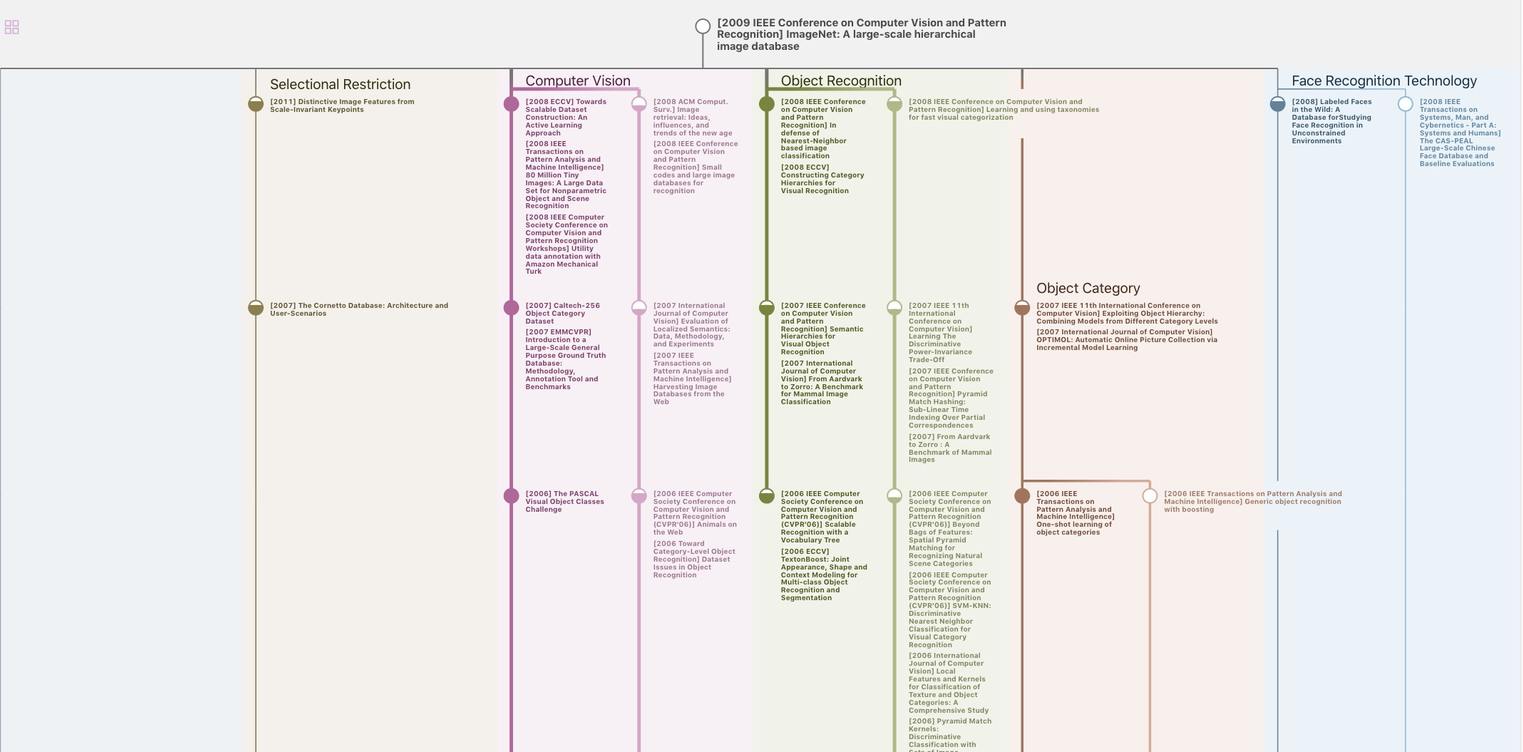
生成溯源树,研究论文发展脉络
Chat Paper
正在生成论文摘要