Understanding AutoML Search Spaces with Local Optima Networks
PROCEEDINGS OF THE 2022 GENETIC AND EVOLUTIONARY COMPUTATION CONFERENCE (GECCO'22)(2022)
摘要
AutoML tackles the problem of automatically configuring machine learning pipelines to specific data analysis problems. These pipelines may include methods for preprocessing and classifying data. There are many strategies to find the best pipeline configuration, and most of them address the problem from an optimization perspective, using Bayesian optimization and evolutionary algorithms. However, little is known about the shape of the search space these methods work upon. What is known for a fact is that these spaces include both categorical, continuous and conditional hyperparameters, and dealing with them may not be trivial. In this direction, this paper performs an analysis of AutoML search spaces using local optimal networks (LON) to better understand the global properties of the search space. Knowing these properties allow us to improve current optimization methods. By analyzing the metrics extracted from the LON we got many insights on the difficulty of the problem.
更多查看译文
关键词
AutoML, fitness landscape analysis, local optimal networks
AI 理解论文
溯源树
样例
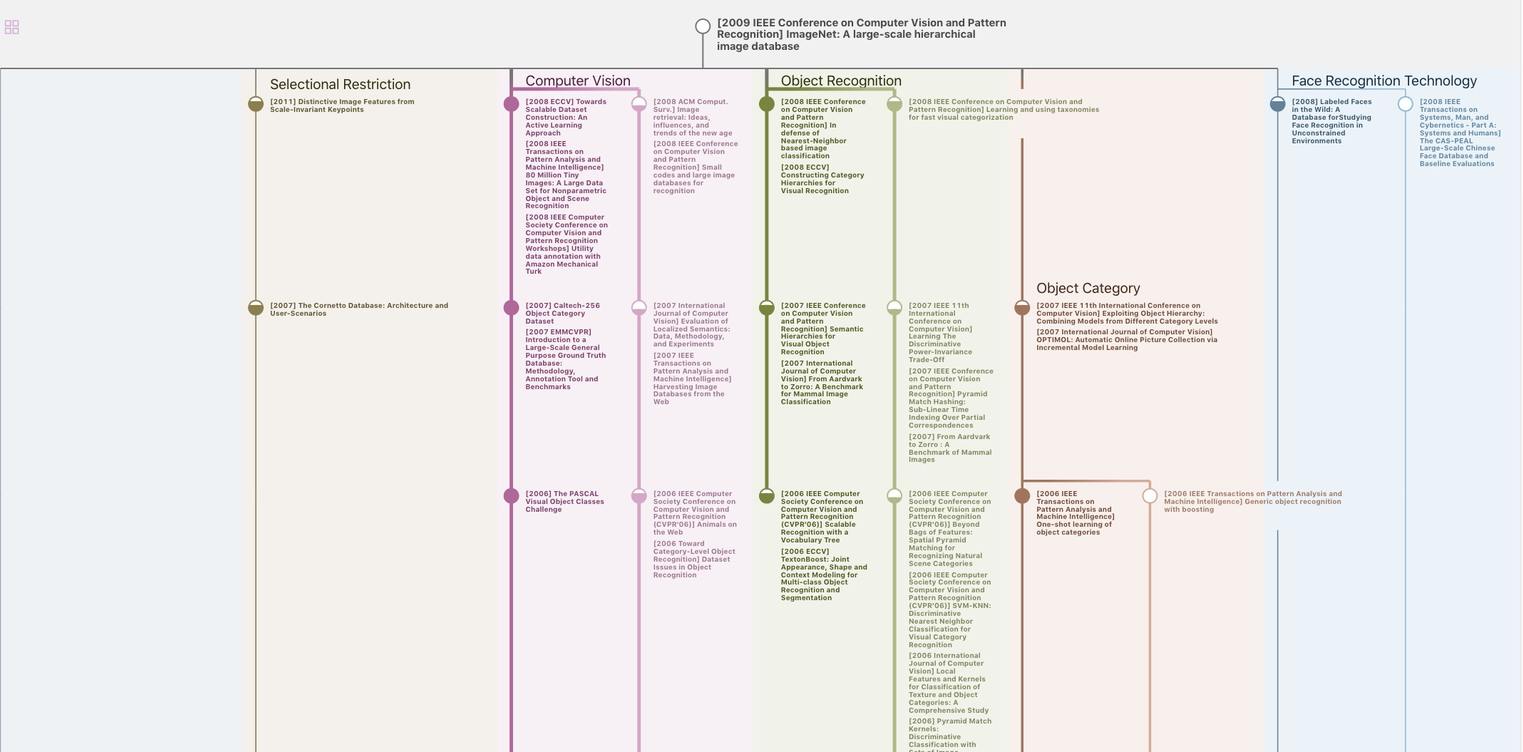
生成溯源树,研究论文发展脉络
Chat Paper
正在生成论文摘要