TAGA: A Transfer-based Black-box Adversarial Attack with Genetic Algorithms
PROCEEDINGS OF THE 2022 GENETIC AND EVOLUTIONARY COMPUTATION CONFERENCE (GECCO'22)(2022)
摘要
Deep learning has been widely adopted in many real-world applications, especially in image classification. However, researches have shown that minor distortions imperceptible to humans may mislead classifiers. One way to improve the robustness is using adversarial attacks to obtain adversarial examples and re-training the classifier with those images. However, the connections between attacks and application scenarios are rarely discussed. This paper proposes a novel black-box adversarial attack that is specifically designed for real-world application scenarios: The transfer-based black-box adversarial attack with genetic algorithms (TAGA). TAGA adopts a genetic algorithm to generate the adversarial examples and reduces the ensuing query costs with a surrogate model based on the transferability of adversarial attacks. Empirical results show that perturbing embeddings in the latent space helps the attack algorithm quickly obtain adversarial examples and that the surrogate fitness function reduces the number of function evaluations. Compared with several state-of-the-art attacks, TAGA improves the classifiers more under the application scenario in terms of the summation of natural and defense accuracy.
更多查看译文
关键词
Deep Learning, Neural Networks, Adversarial Attacks, Genetic, Algorithms
AI 理解论文
溯源树
样例
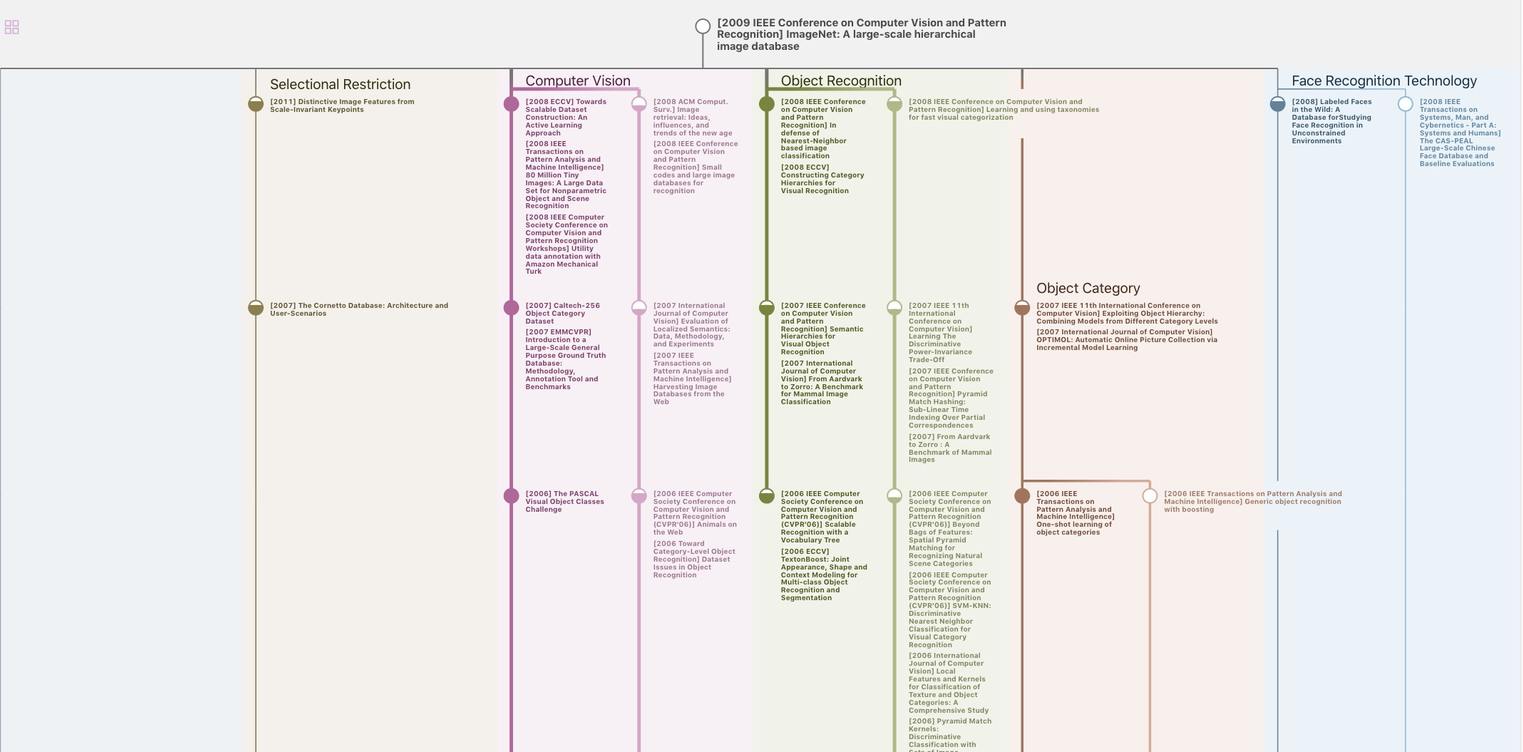
生成溯源树,研究论文发展脉络
Chat Paper
正在生成论文摘要