A Bounded Archive Based for Bi-objective Problems based on Distance and epsilon-dominance to avoid Cyclic Behavior
PROCEEDINGS OF THE 2022 GENETIC AND EVOLUTIONARY COMPUTATION CONFERENCE (GECCO'22)(2022)
摘要
One important issue in evolutionary multi-objective optimization (EMO) which still leaves room for improvement is the maintenance of the subset of the obtained candidate solutions that forms the approximation of the Pareto front (in short: selection or archiving). Existing archivers that are entirely based on the distances between the candidate solutions are known to reveal cyclic behavior and do hence not tap their full potential. On the other hand, there exist archivers based on epsilon-dominance that guarantee monotonic behaviors as well as certain approximation qualities in the limit. For such methods, however, so far the magnitudes of the final archive size heavily depend on several design parameters and cannot be bounded a priori which is desired by many EMO researchers. In this paper, we propose a new archiver, ArchiveUpdateBound, for bi-objective problems that is based both on distance and epsilon-dominance. ArchiveUpdateBound aims for a uniform spread of the solutions along the Pareto front, and the archive sizes can be bounded above. In particular, the use of epsilon-dominance eliminates the occurrence of cyclic behavior. Numerical results using NSGA-II, MOEA/D, and SMS-MOEA on selected benchmark problems indicate that the use of the novel archiver significantly increases the performance of the base MOEAs.
更多查看译文
关键词
evolutionary multi-objective optimization, archiving
AI 理解论文
溯源树
样例
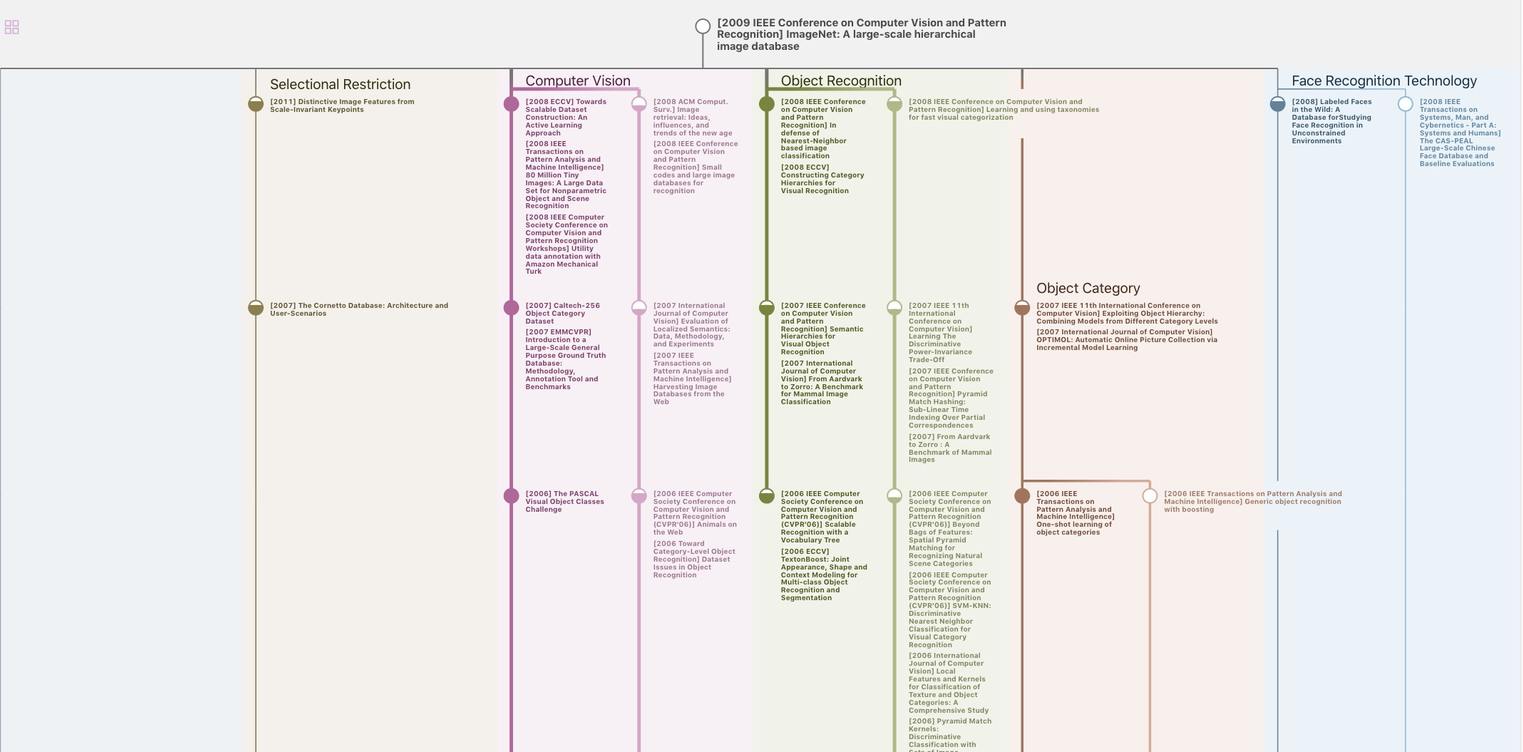
生成溯源树,研究论文发展脉络
Chat Paper
正在生成论文摘要