Learning Rate Adaptation by Line Search in Evolution Strategies with Recombination
PROCEEDINGS OF THE 2022 GENETIC AND EVOLUTIONARY COMPUTATION CONFERENCE (GECCO'22)(2022)
摘要
In this paper, we investigate the effect of a learning rate for the mean in Evolution Strategies with recombination. We study the effect of a half-line search after the mean shift direction is established, hence the learning rate value is conditioned to the direction. We prove convergence and study convergence rates in different dimensions and for different population sizes on the sphere function with the step-size proportional to the distance to the optimum. We empirically find that a perfect half-line search increases the maximal convergence rate on the sphere function by up to about 70%, assuming the line search imposes no additional costs. The speedup becomes less pronounced with increasing dimension. The line search reduces-however does not eliminate-the dependency of the convergence rate on the step-size. The optimal step-size assumes considerably smaller values with line search, which is consistent with previous results for different learning rate settings. The step-size difference is more pronounced in larger dimension and with larger population size, thereby diminishing an important advantage of a large population.
更多查看译文
关键词
Evolution Strategy, line search, convergence rate
AI 理解论文
溯源树
样例
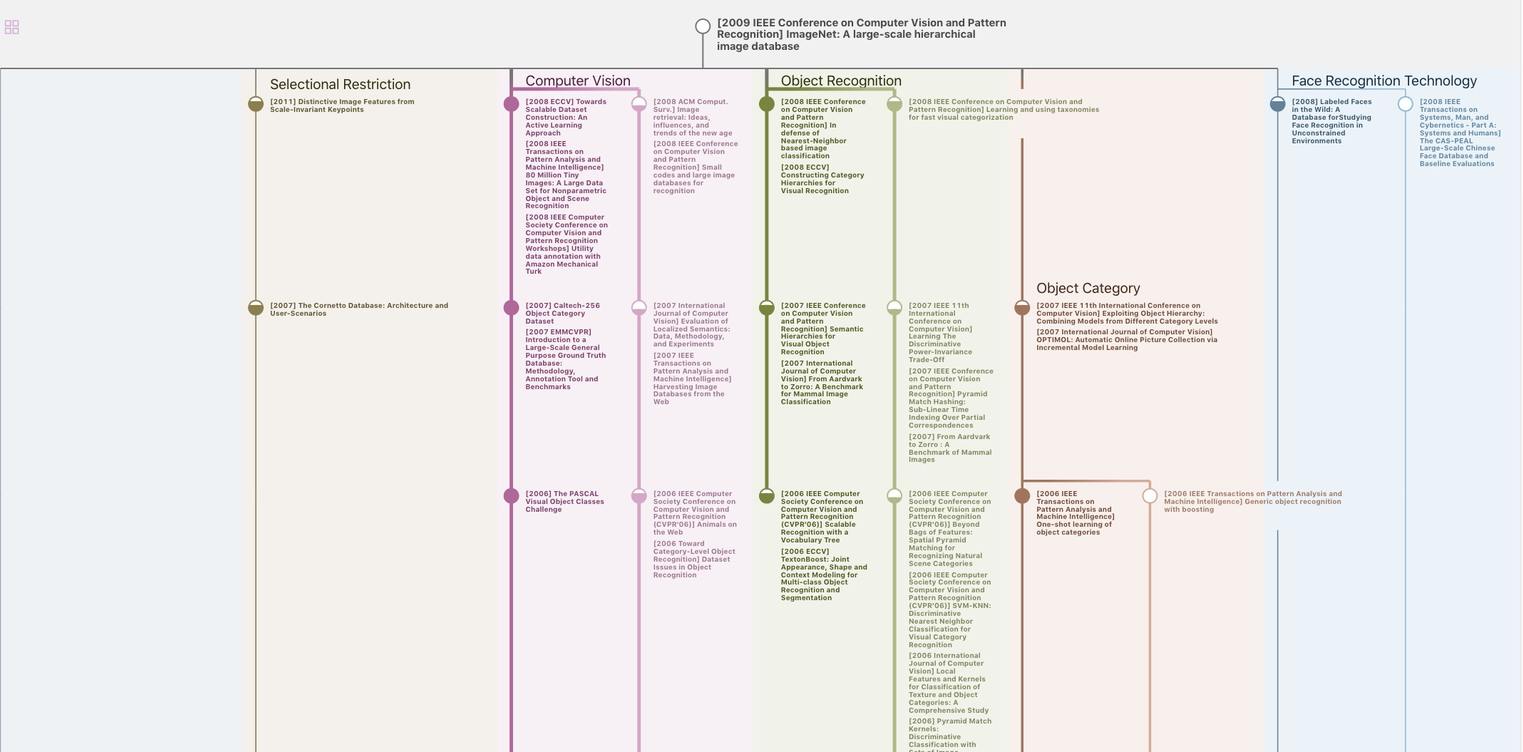
生成溯源树,研究论文发展脉络
Chat Paper
正在生成论文摘要