Fast Non-elitist Evolutionary Algorithms with Power-law Ranking Selection
PROCEEDINGS OF THE 2022 GENETIC AND EVOLUTIONARY COMPUTATION CONFERENCE (GECCO'22)(2022)
摘要
Theoretical evidence suggests that non-elitist evolutionary algorithms (EAs) with non-linear selection mechanisms can efficiently overcome broad classes of local optima where elitist EAs fail. However, the analysis assumes a weak selective pressure and mutation rates carefully chosen close to the "error threshold", above which they cease to be efficient. On problems easier for hill-climbing, the populations may slow down these algorithms, leading to worse runtime compared with variants of the elitist (1+1) EA. Here, we show that a non-elitist EA with power-law ranking selection leads to fast runtime on easy benchmark problems, while maintaining the capability of escaping certain local optima where the elitist EAs spend exponential time in the expectation. We derive a variant of the level-based theorem which accounts for power-law distributions. For classical theoretical benchmarks, the expected runtime is stated with small leading constants. For complex, multi-modal fitness landscapes, we provide sufficient conditions for polynomial optimisation, formulated in terms of deceptive regions sparsity and fitness valleys density. We derive the error threshold and show extreme tolerance to high mutation rates. Experiments on NK-Landscape functions, generated according to the Kauffman's model, show that the algorithm outperforms the (1+1) EA and the univariate marginal distribution algorithm ( UMDA).
更多查看译文
关键词
Runtime analysis, Power-law ranking, Selection, Local optima
AI 理解论文
溯源树
样例
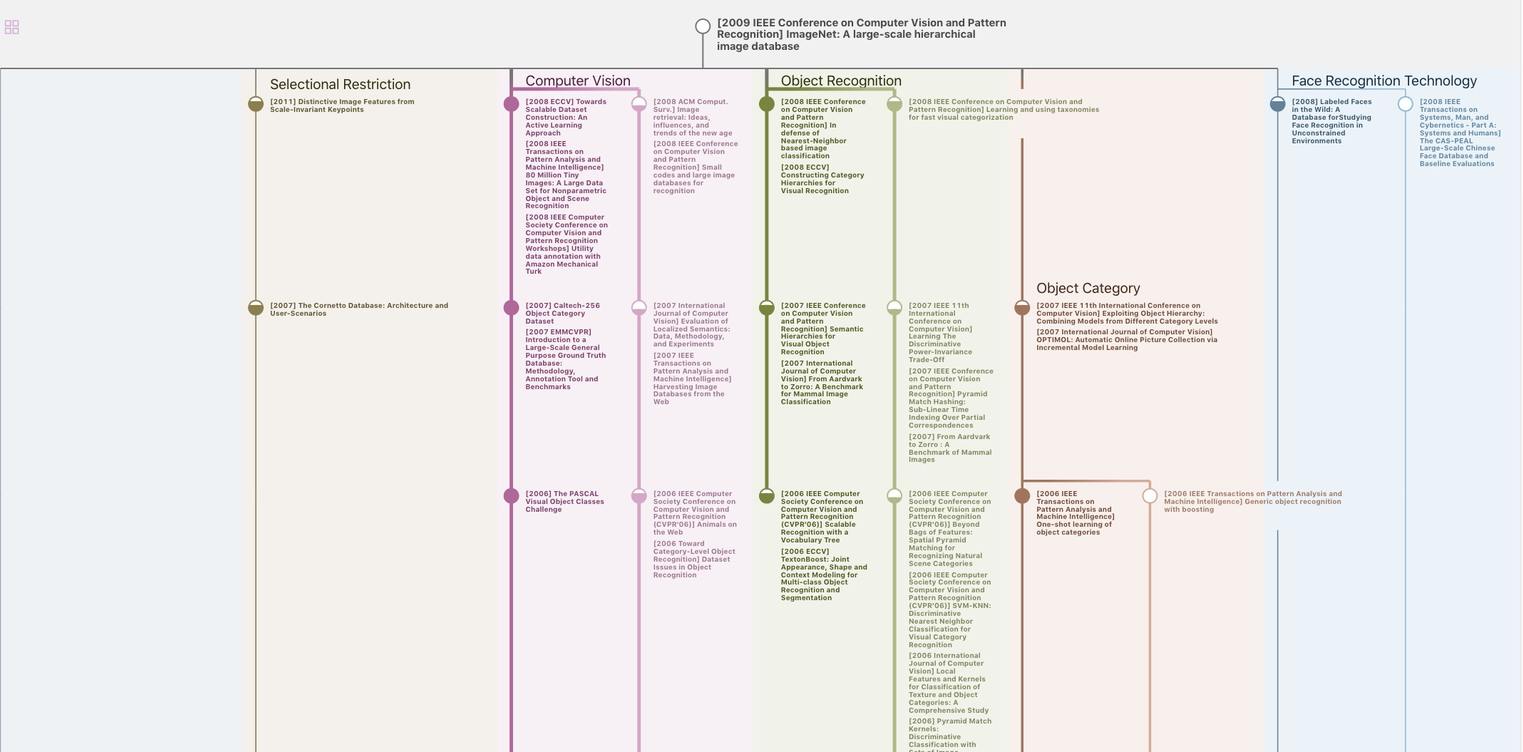
生成溯源树,研究论文发展脉络
Chat Paper
正在生成论文摘要