Modeling Temporal-Sensitive Information for Complex Question Answering over Knowledge Graphs
NATURAL LANGUAGE PROCESSING AND CHINESE COMPUTING, NLPCC 2022, PT I(2022)
摘要
Question answering over temporal knowledge graphs (TKGQA) has attracted great attentions in natural language processing community. One of the key challenges is how to effectively model the representations of questions and the candidate answers associated with timestamp constraints. Many existing methods attempt to learn temporal knowledge graph embedding for entities, relations and timestamps. However, these existing methods cannot effectively exploiting temporal knowledge graph embeddings to capture time intervals (e.g., "WWII" refers to 1939-1945) as well as temporal relation words (e.g., "first" and "last") appeared in complex questions, resulting in the sub-optimal results. In this paper, we propose a temporal-sensitive information for complex question answering (TSIQA) framework to tackle these problems. We employ two alternative approaches to augment questions embeddings with question-specific time interval information, which consists of specific start and end timestamps. We also present auxiliary contrastive learning to contrast the answer prediction and prior knowledge regarding time approximation for questions that only differ by the temporal relation words. To evaluate the effectiveness of our proposed method, we conduct the experiments on CRONQUESTION. The results show that our proposed model achieves better improvements over the state-of-the-art models that require multiple steps of reasoning.
更多查看译文
关键词
Question answering, Temporal knowledge graphs, Knowledge graph embedding
AI 理解论文
溯源树
样例
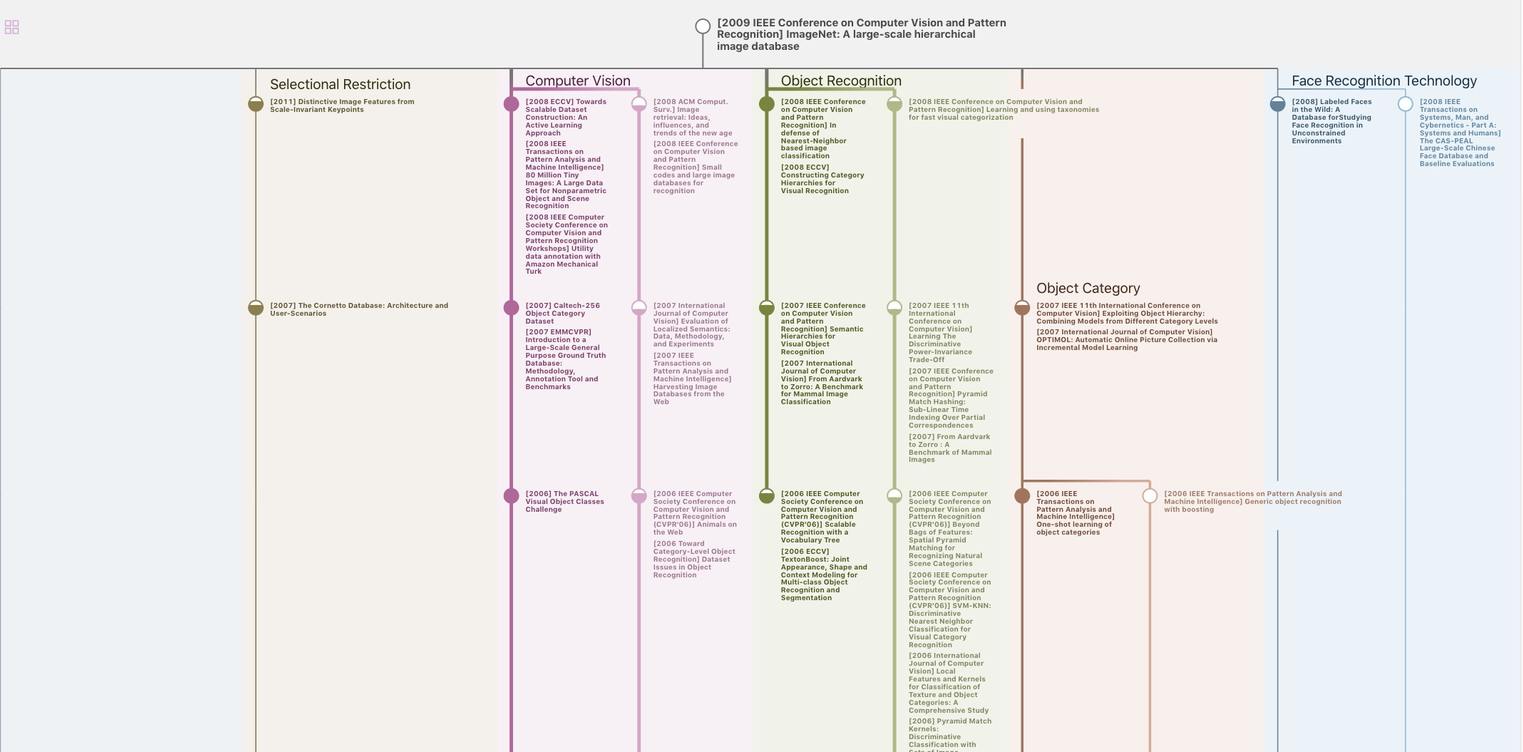
生成溯源树,研究论文发展脉络
Chat Paper
正在生成论文摘要