ADS-Cap: A Framework for Accurate and Diverse Stylized Captioning with Unpaired Stylistic Corpora
NATURAL LANGUAGE PROCESSING AND CHINESE COMPUTING, NLPCC 2022, PT I(2022)
摘要
Generating visually grounded image captions with specific linguistic styles using unpaired stylistic corpora is a challenging task, especially since we expect stylized captions with a wide variety of stylistic patterns. In this paper, we propose a novel framework to generate Accurate and Diverse Stylized Captions (ADS-Cap). Our ADS-Cap first uses a contrastive learning module to align the image and text features, which unifies paired factual and unpaired stylistic corpora during the training process. A conditional variational auto-encoder is then used to automatically memorize diverse stylistic patterns in latent space and enhance diversity through sampling. We also design a simple but effective recheck module to boost style accuracy by filtering style-specific captions. Experimental results on two widely used stylized image captioning datasets show that regarding consistency with the image, style accuracy and diversity, ADS-Cap achieves outstanding performances compared to various baselines. We finally conduct extensive analyses to understand the effectiveness of our method. (Our code is available at https://github. com/njucckevin/ADS-Cap.)
更多查看译文
关键词
Stylized image captioning, Contrastive learning, Conditional variational auto-encoder
AI 理解论文
溯源树
样例
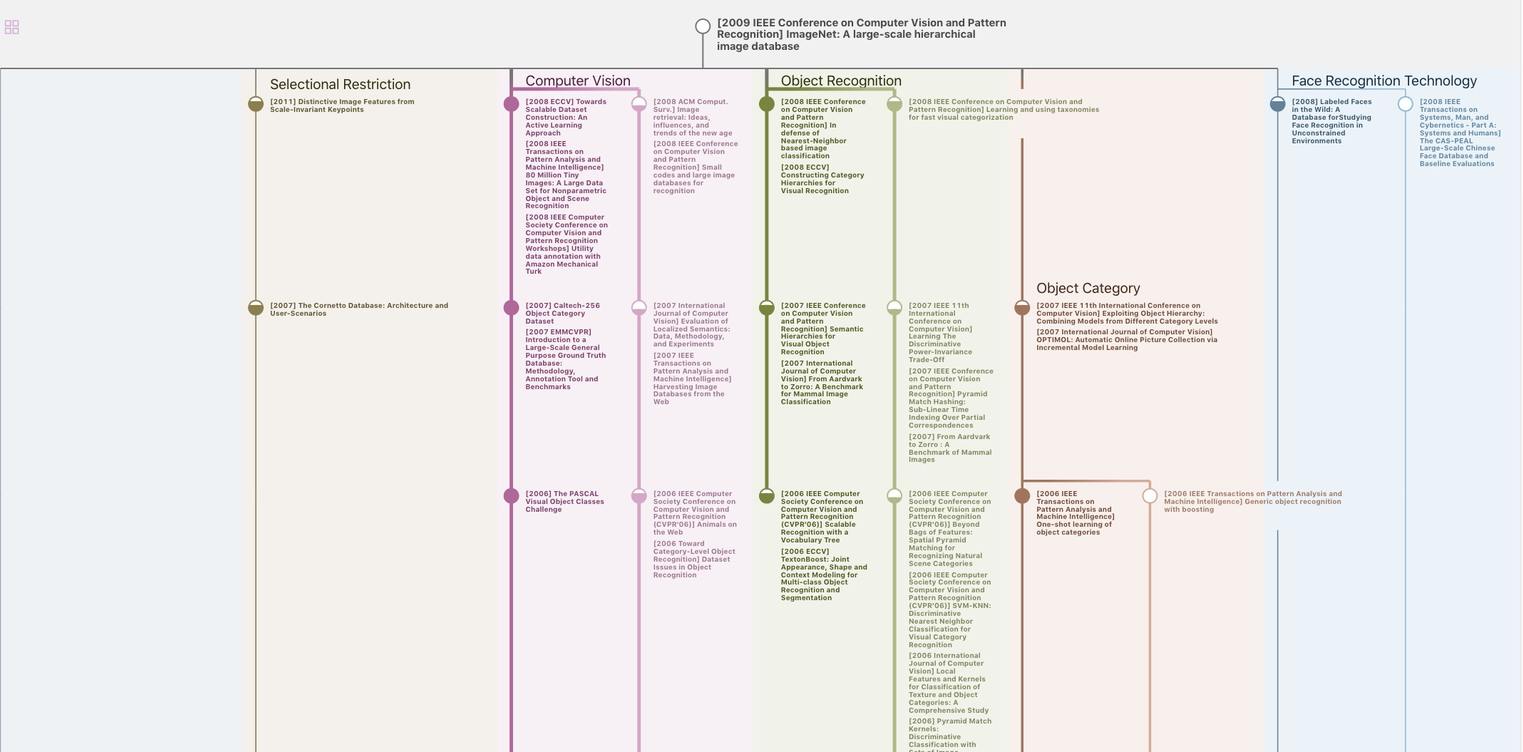
生成溯源树,研究论文发展脉络
Chat Paper
正在生成论文摘要