Learning Under Label Noise for Robust Spoken Language Understanding systems
Conference of the International Speech Communication Association (INTERSPEECH)(2022)
摘要
Most real-world datasets contain inherent label noise which leads to memorization and overfitting when such data is used to train over-parameterized deep neural networks. While memorization in DNNs has been studied extensively in computer vision literature, the impact of noisy labels and various mitigation strategies in Spoken Language Understanding tasks is largely under-explored. In this paper, we perform a systematic study on the effectiveness of five noise mitigation methods in Spoken Language text classification tasks. First, we experiment on three publicly available datasets by synthetically injecting noise into the labels and evaluate the effectiveness of various methods at different levels of noise intensity. We then evaluate these methods on a real-world data coming from a large-scale industrial Spoken Language Understanding system. Our results show that most methods are effective in mitigating the impact of noise with two of the methods showing consistently better results. For the industrial Spoken Language Understanding system, the best performing method is able to recover up to 97% of the loss in performance due to noise.
更多查看译文
关键词
label noise,learning,language
AI 理解论文
溯源树
样例
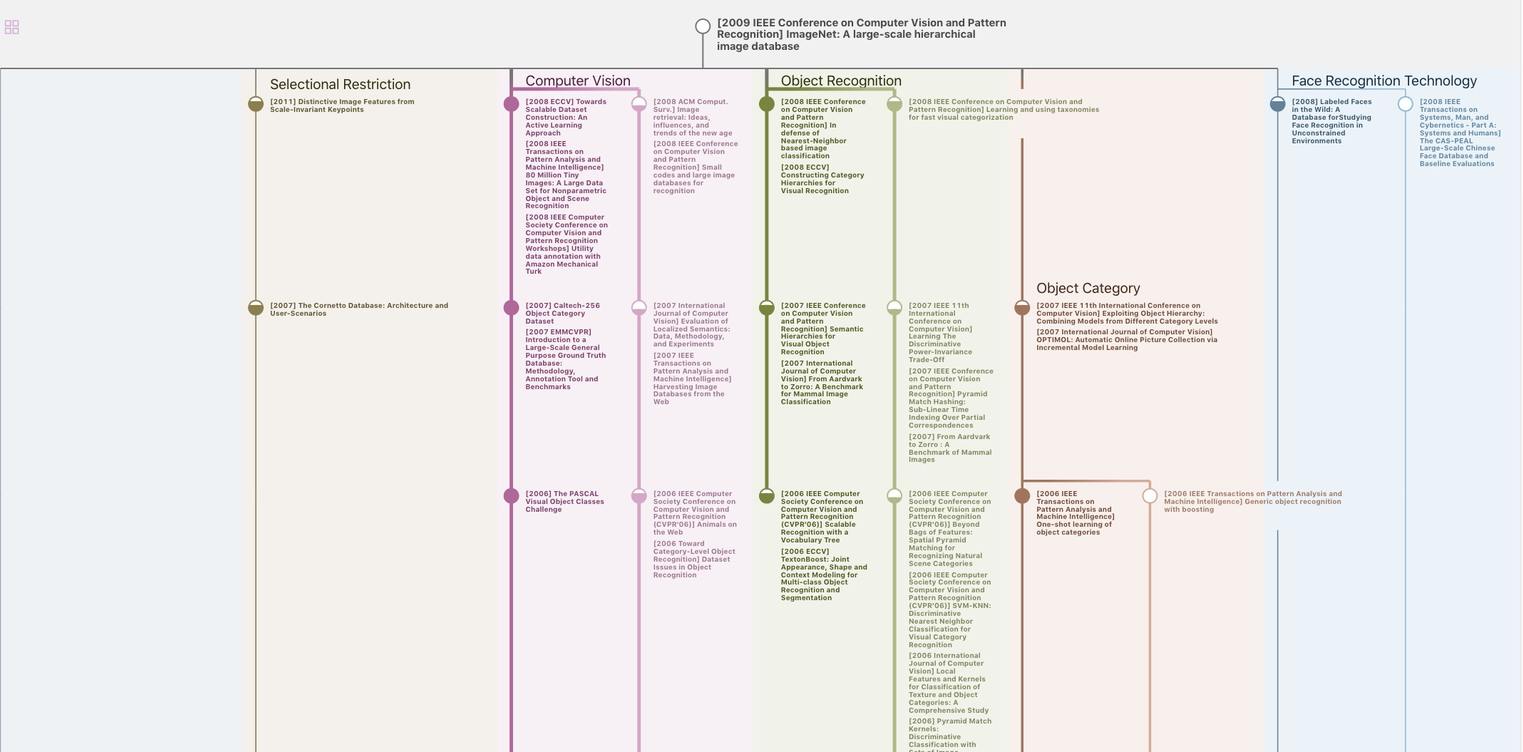
生成溯源树,研究论文发展脉络
Chat Paper
正在生成论文摘要