From Undercomplete to Sparse Overcomplete Autoencoders to Improve LF-MMI Speech Recognition
Conference of the International Speech Communication Association (INTERSPEECH)(2022)
摘要
Starting from a strong Lattice-Free Maximum Mutual Information (LF-MMI) baseline system, we explore different autoencoder configurations to enhance Mel-Frequency Cepstral Coefficients (MFCC) features. Autoencoders are expected to generate new MFCC features that can be used in our LF-MMI based baseline system (with or without retraining) towards speech recognition improvements. Starting from shallow undercomplete autoencoders, and their known equivalence with Principal Component Analysis (PCA), we go to deeper or sparser architectures. In the spirit of kernel-based learning methods, we explore alternatives where the autoencoder first goes overcomplete (i.e., expand the representation space) in a nonlinear way, and then we restrict the autoencoder by means of a sequent bottleneck layer. Finally, as a third solution, we use sparse overcomplete autoencoders where a sparsity constraint is imposed on the higher-dimensional encoding layer. Experimental results are provided on the Augmented Multiparty Interaction (AMI) dataset, where we show that all aforementioned architectures improve speech recognition performance.
更多查看译文
关键词
pca, bottleneck, sparse overcomplete autoencoder, chain models, speech recognition
AI 理解论文
溯源树
样例
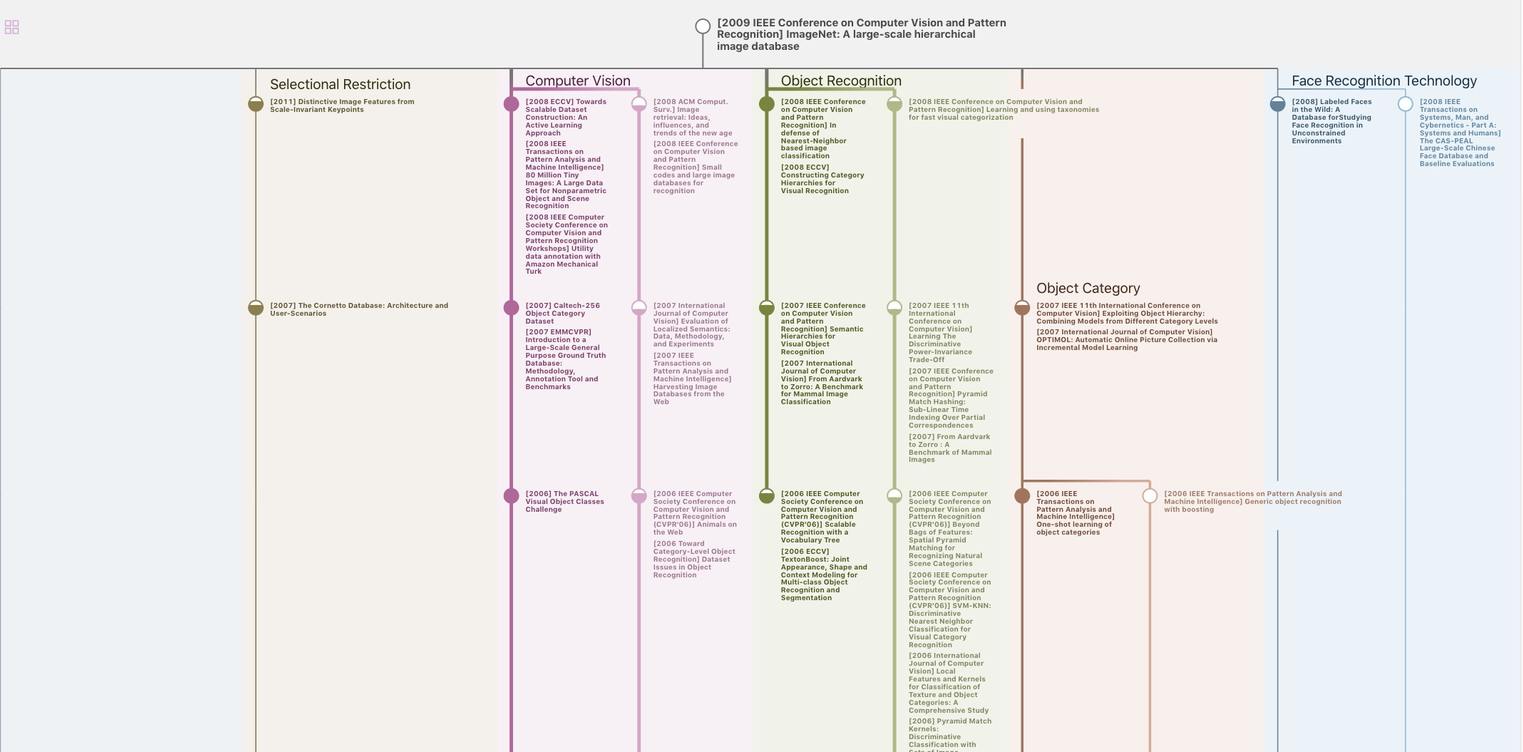
生成溯源树,研究论文发展脉络
Chat Paper
正在生成论文摘要