Multiple Enhancements to LSTM for Learning Emotion-Salient Features in Speech Emotion Recognition
Conference of the International Speech Communication Association (INTERSPEECH)(2022)
摘要
Emotion-relevant feature extraction is key to the speech emotion recognition (SER) task. Although neural network for extracting features has achieved excellent results, in particular long short-term memory (LSTM) based models, there is still ample space for improvement. In this paper, from the perspective of utilizing advantages of multiple models, we propose an approach of multiple enhancements for learning emotion-salient features in SER, which is based on the combination of LSTM, one-dimensional convolution and transformer networks. Firstly, we introduce residual-BLSTM (Bidirectional LSTM) module to make the network deeper and to increase the learning ability of the model by adding feed-forward network (FFN) to the output of BLSTM and building residual connections at the same time. Secondly, time pooling employed in residual-BLSTM module is proposed to reduce features redundancy and overcome training overfitting. Finally, we propose an E-transformer module by combining transformer and convolution neural network. This approach enables it to learn local information while capturing global dependencies. We conduct evaluations on the IEMOCAP dataset using the proposed methods, and it shows the state-of-the-art performances.
更多查看译文
关键词
emotion-salient recognition,lstm
AI 理解论文
溯源树
样例
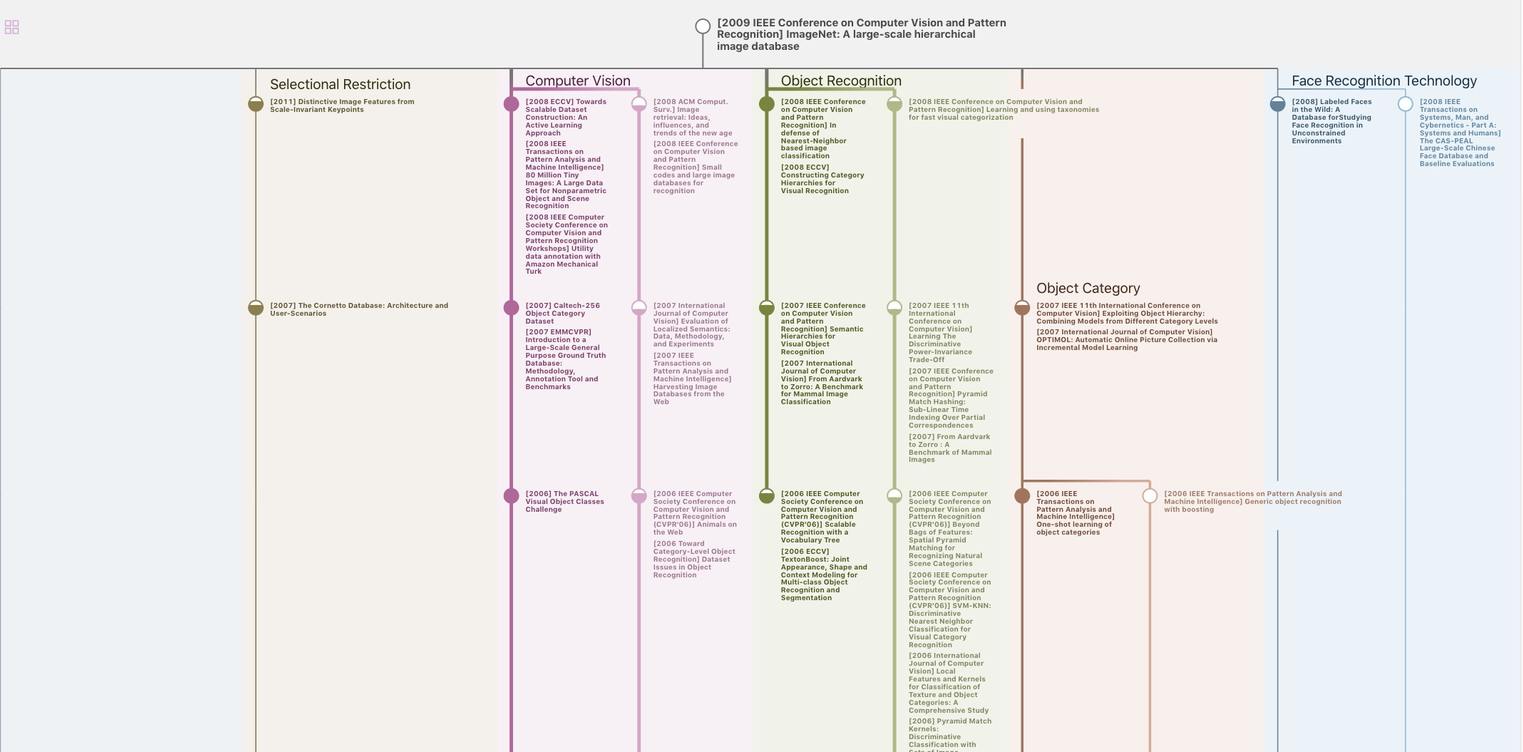
生成溯源树,研究论文发展脉络
Chat Paper
正在生成论文摘要