Class-Aware Distribution Alignment based Unsupervised Domain Adaptation for Speaker Verification
Conference of the International Speech Communication Association (INTERSPEECH)(2022)
摘要
Existing speaker verification (SV) systems usually suffer from significant performance degradation when applied to a new domain that lies outside the training distribution. Given the unlabeled target-domain dataset, most Unsupervised Domain Adaptation (UDA) methods aim to minimize the distribution divergence between different domains. However, global distribution alignment strategies fail to consider latent speaker label information and can hardly guarantee the feature discriminative capability in target domain. In this paper, we propose a novel UDA approach called WBDA (Within-class and Between-class Distribution Alignment), which aims to transfer the class-aware information (i.e., within- and between-class distributions) learned from the well-labeled source-domain, to the unlabeled target-domain. Motivated by the recent progress of self-supervised contrastive learning, positive and negative pairs are constructed separately for source and target domains, from which the within- and between-class distribution can be estimated. And the SV system can then be learned by jointly optimizing the cross-domain class-aware distribution discrepancy loss and source-domain classification loss in an end-to-end manner. Evaluations on NIST SRE16 and SRE18 achieve a relative performance improvement of about 43.7% and 26.2% over the baseline in terms of Equal Error Rate (EER) separately, significantly outperforming the previous adaption methods based on global distribution alignment.
更多查看译文
关键词
speaker verification,unsupervised domain adaptation,class-aware
AI 理解论文
溯源树
样例
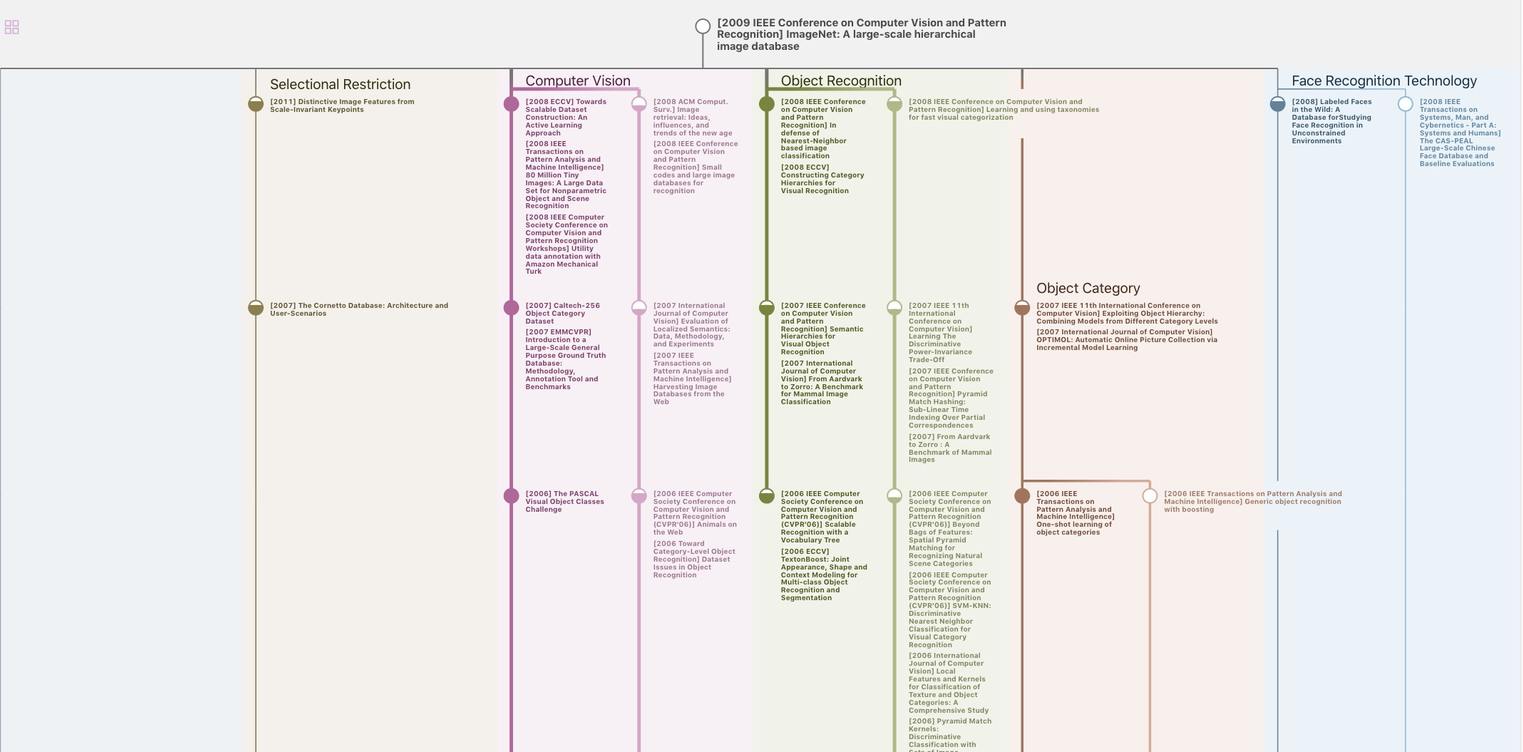
生成溯源树,研究论文发展脉络
Chat Paper
正在生成论文摘要