Transfer Learning from Multi-Lingual Speech Translation Benefits Low-Resource Speech Recognition
Conference of the International Speech Communication Association (INTERSPEECH)(2022)
摘要
In this article, we propose a simple yet effective approach to train an end-to-end speech recognition system on languages with limited resources by leveraging a large pre-trained wav2vec2.0 model fine-tuned on a multi-lingual speech translation task. We show that the weights of this model form an excellent initialization for Connectionist Temporal Classification (CTC) speech recognition, a different but closely related task. We explore the benefits of this initialization for various languages, both in-domain and out-of-domain for the speech translation task. Our experiments on the CommonVoice dataset confirm that our approach performs significantly better in-domain, and is often better out-of-domain too. This method is particularly relevant for Automatic Speech Recognition (ASR) with limited data and/or compute budget during training.
更多查看译文
关键词
transfer learning,speech recognition,translation,multi-lingual,low-resource
AI 理解论文
溯源树
样例
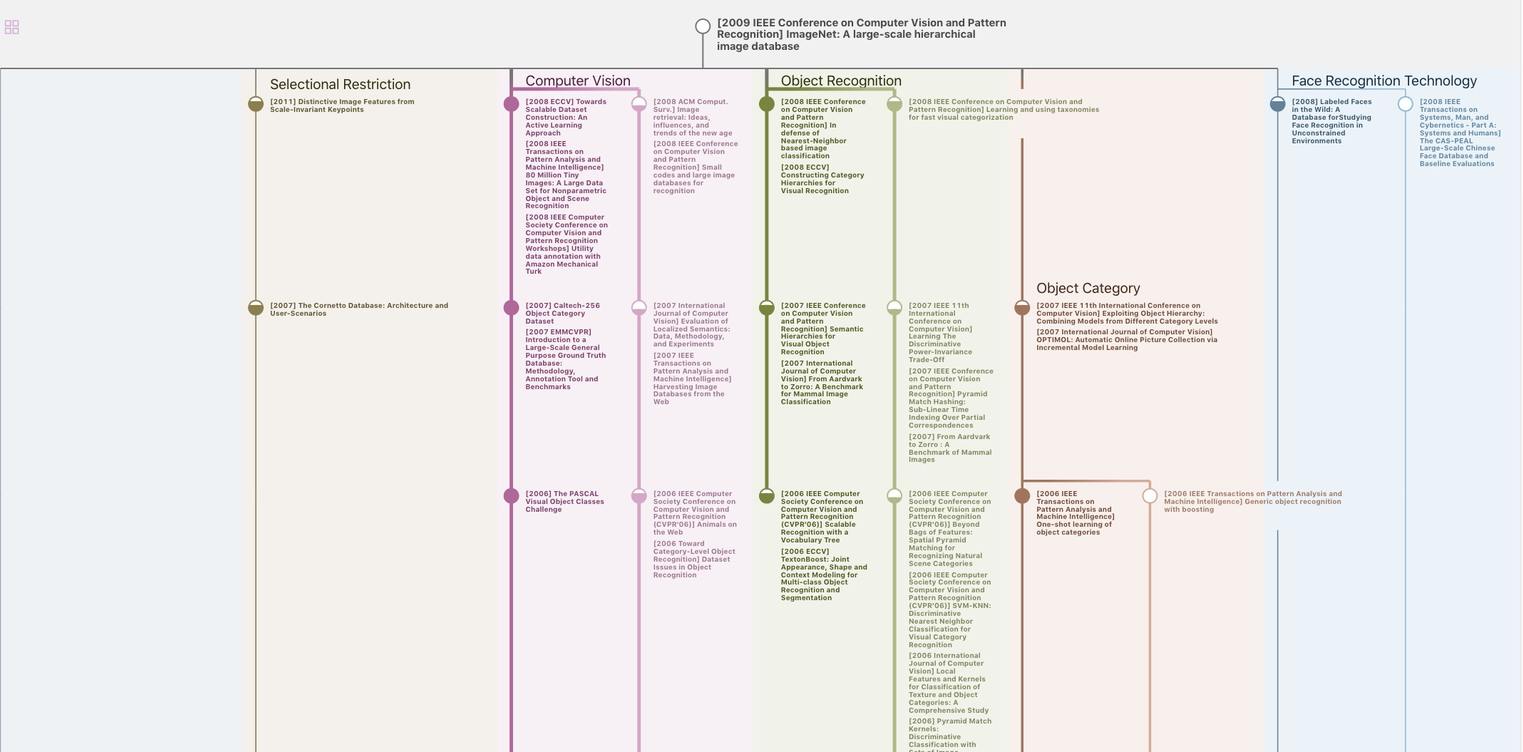
生成溯源树,研究论文发展脉络
Chat Paper
正在生成论文摘要