Efficient Kernelized UCB for Contextual Bandits
International Conference on Artificial Intelligence and Statistics (AISTATS)(2022)
摘要
In this paper, we tackle the computational efficiency of kernelized UCB algorithms in contextual bandits. While standard methods require a O(CT3) complexity where T is the horizon and the constant C is related to optimizing the UCB rule, we propose an efficient contextual algorithm for large-scale problems. Specifically, our method relies on incremental Nystrom approximations of the joint kernel embedding of contexts and actions. This allows us to achieve a complexity of O(CTm2) where m is the number of Nystrom points. To recover the same regret as the standard kernelized UCB algorithm, m needs to be of order of the effective dimension of the problem, which is at most O(root T) and nearly constant in some cases.
更多查看译文
AI 理解论文
溯源树
样例
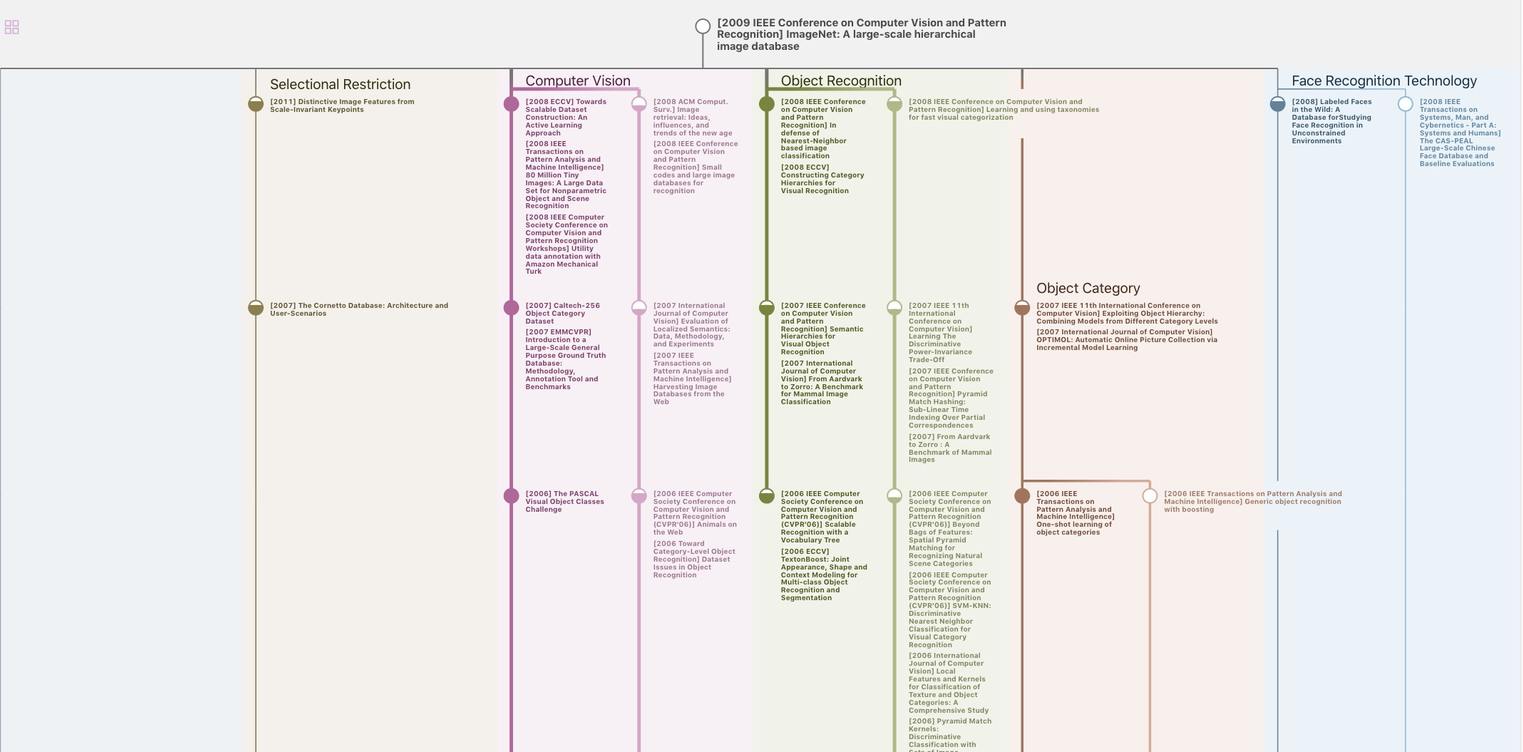
生成溯源树,研究论文发展脉络
Chat Paper
正在生成论文摘要