Warping Layer: Representation Learning for Label Structures in Weakly Supervised Learning
INTERNATIONAL CONFERENCE ON ARTIFICIAL INTELLIGENCE AND STATISTICS, VOL 151(2022)
摘要
Many learning tasks only receive weak supervision, such as semi-supervised learning and few-shot learning. With limited labeled data, prior structures become especially important, and prominent examples include hierarchies and mutual exclusions in the class space. However, most existing approaches only learn the representations separately in the feature space and the label space, and do not explicitly enforce the logical relationships. In this paper, we propose a novel warping layer that jointly learns representations in both spaces, and thanks to the modularity and differentiability, it can be directly embedded into generative models to leverage the prior hierarchical structure and unlabeled data. The effectiveness of the warping layer is demonstrated on both few-shot and semi-supervised learning, outperforming the state of the art in practice.
更多查看译文
AI 理解论文
溯源树
样例
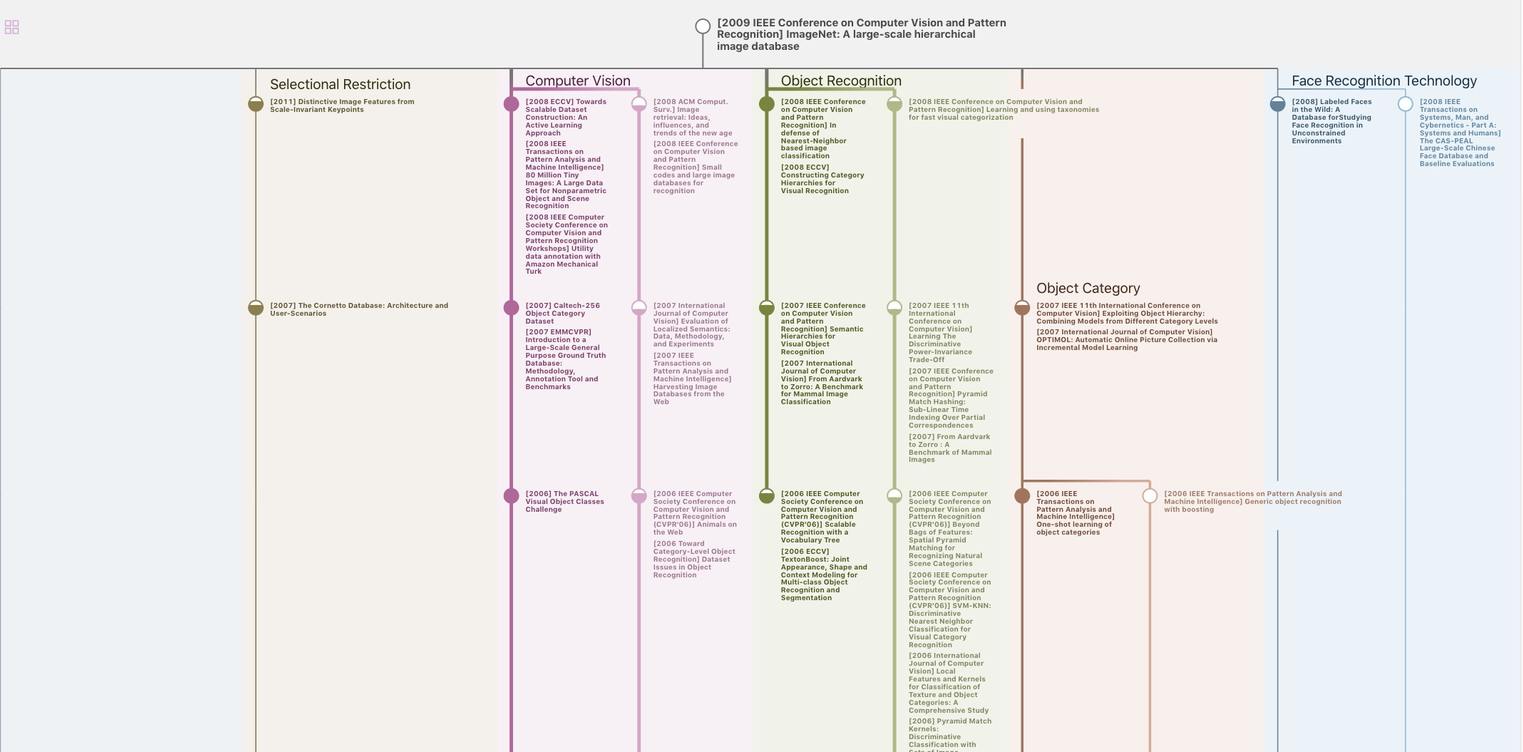
生成溯源树,研究论文发展脉络
Chat Paper
正在生成论文摘要