Firebolt: Weak Supervision Under Weaker Assumptions
INTERNATIONAL CONFERENCE ON ARTIFICIAL INTELLIGENCE AND STATISTICS, VOL 151(2022)
摘要
Modern machine learning demands a large amount of training data. Weak supervision is a promising approach to meet this demand. It aggregates multiple labeling functions (LFs) noisy, user-provided labeling heuristics to rapidly and cheaply curate probabilistic labels for large-scale unlabeled data. However, standard assumptions in weak supervision such as user-specified class bal- ance, similar accuracy of an LF in classifying different classes, and full knowledge of LF de- pendency at inference time might be undesir- able in practice. In response, we present Firebolt, a new weak supervision framework that seeks to operate under weaker assumptions. In particular, Firebolt learns the class balance and class-specific accuracy of LFs jointly from unlabeled data. It carries out inference in an efficient and interpretable manner. We analyze the parameter estimation error of Firebolt and characterize its impact on downstream model performance. Furthermore, we show that on five publicly available datasets, Firebolt outperforms a state-of-the-art weak supervision method by up to 5.8 points in AUC. We also provide a case study in the production setting of a tech company, where a Firebolt-supervised model outperforms the existing weakly-supervised production model by 1.3 points in AUC and speeds up label model training and inference from one hour to three minutes.
更多查看译文
AI 理解论文
溯源树
样例
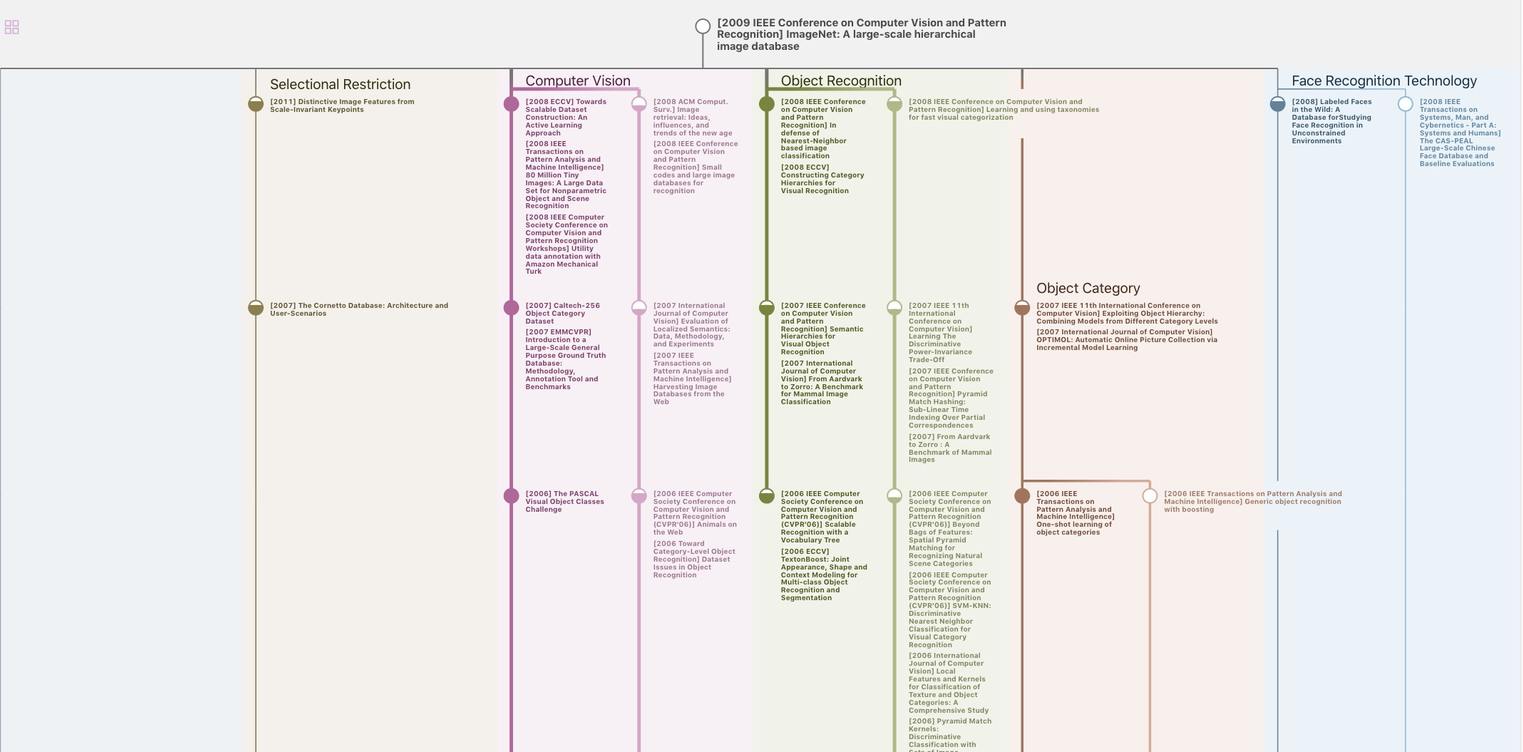
生成溯源树,研究论文发展脉络
Chat Paper
正在生成论文摘要