Multi-task Knowledge Graph Representations via Residual Functions
ADVANCES IN KNOWLEDGE DISCOVERY AND DATA MINING, PAKDD 2022, PT I(2022)
摘要
In this paper, we propose MuTATE, a Multi-Task Augmented approach to learn Transferable Embeddings of knowledge graphs. Previous knowledge graph representation techniques either employ task-agnostic geometric hypotheses to learn informative node embeddings or integrate task-specific learning objectives like attribute prediction. In contrast, our framework unifies multiple co-dependent learning objectives with knowledge graph enrichment. We define co-dependence as multiple tasks that extract covariant distributions of entities and their relationships for prediction or regression objectives. We facilitate knowledge transfer in this setting: tasks -> graph, graph -> tasks, and task-1 -> task-2 via task-specific residual functions to specialize the node embeddings for each task, motivated by domain-shift theory. We show 5% relative gains over state-of-the-art knowledge graph embedding baselines on two public multi-task datasets and show significant potential for cross-task learning.
更多查看译文
关键词
Knowledge graphs, Knowledge graph embedding, Graph neural networks, Multi-task learning, Residual learning
AI 理解论文
溯源树
样例
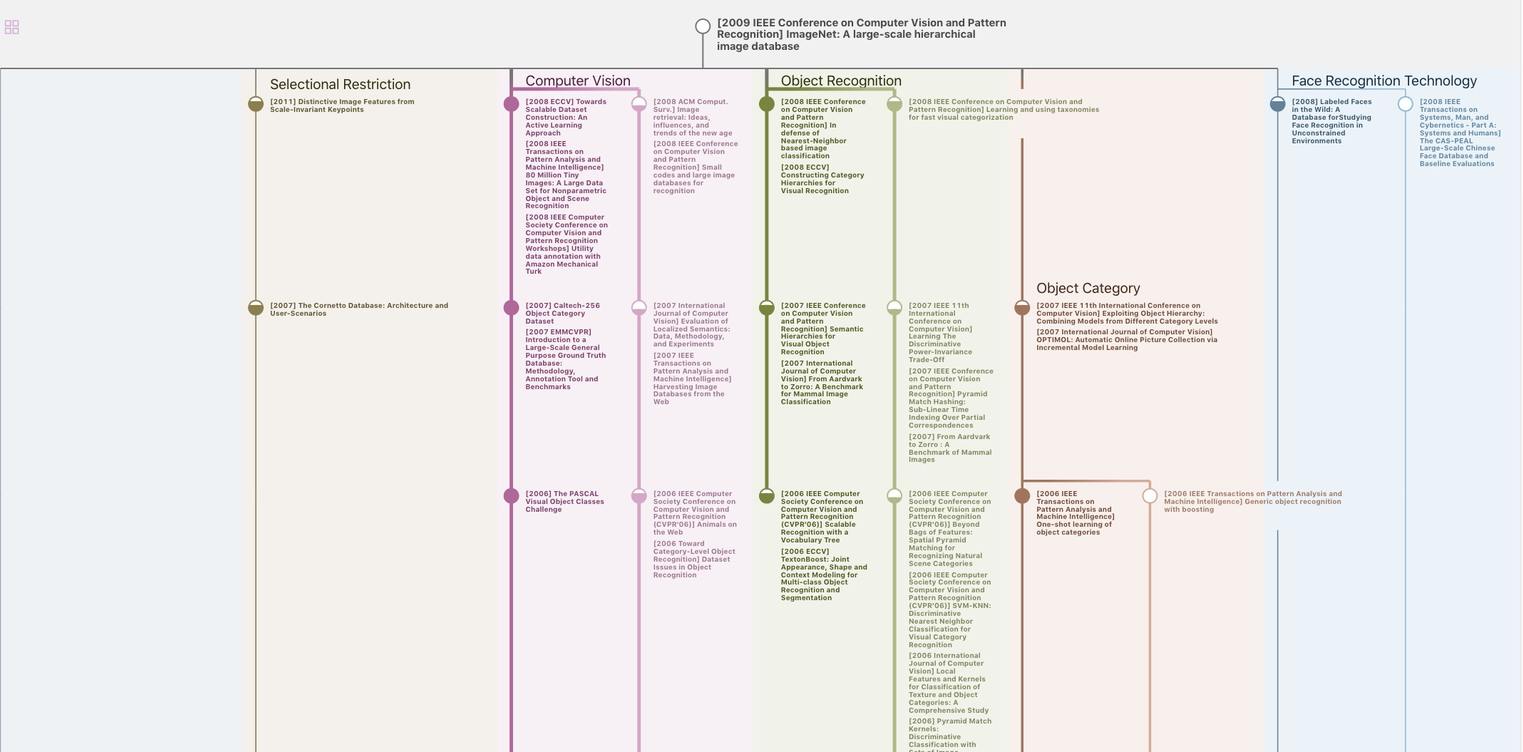
生成溯源树,研究论文发展脉络
Chat Paper
正在生成论文摘要