Grasp Position Estimation from Depth Image Using Stacked Hourglass Network Structure
2022 IEEE 46TH ANNUAL COMPUTERS, SOFTWARE, AND APPLICATIONS CONFERENCE (COMPSAC 2022)(2022)
摘要
In recent years, robots have been used not only in factories. However, most robots currently used in such places can only perform the actions programmed to perform in a predefined space. For robots to become widespread in the future, not only in factories, distribution warehouses, and other places but also in homes and other environments where robots receive complex commands and their surroundings are constantly being updated, it is necessary to make robots intelligent. Therefore, this study proposed a deep learning grasp position estimation model using depth images to achieve intelligence in pick-and-place. This study used only depth images as the training data to build the deep learning model. Some previous studies have used RGB images and depth images. However, in this study, we used only depth images as training data because we expect the inference to be based on the object's shape, independent of the color information of the object. By performing inference based on the target object's shape, the deep learning model is expected to minimize the need for re-training when the target object package changes in the production line since it is not dependent on the RGB image. In this study, we propose a deep learning model that focuses on the stacked encoder-decoder structure of the Stacked Hourglass Network. We compared the proposed method with the baseline method in the same evaluation metrics and a real robot, which shows higher accuracy than other methods in previous studies.
更多查看译文
关键词
Deep Learning, Depth image, Robotic Grasping, Grasping Point Estimation, Robust
AI 理解论文
溯源树
样例
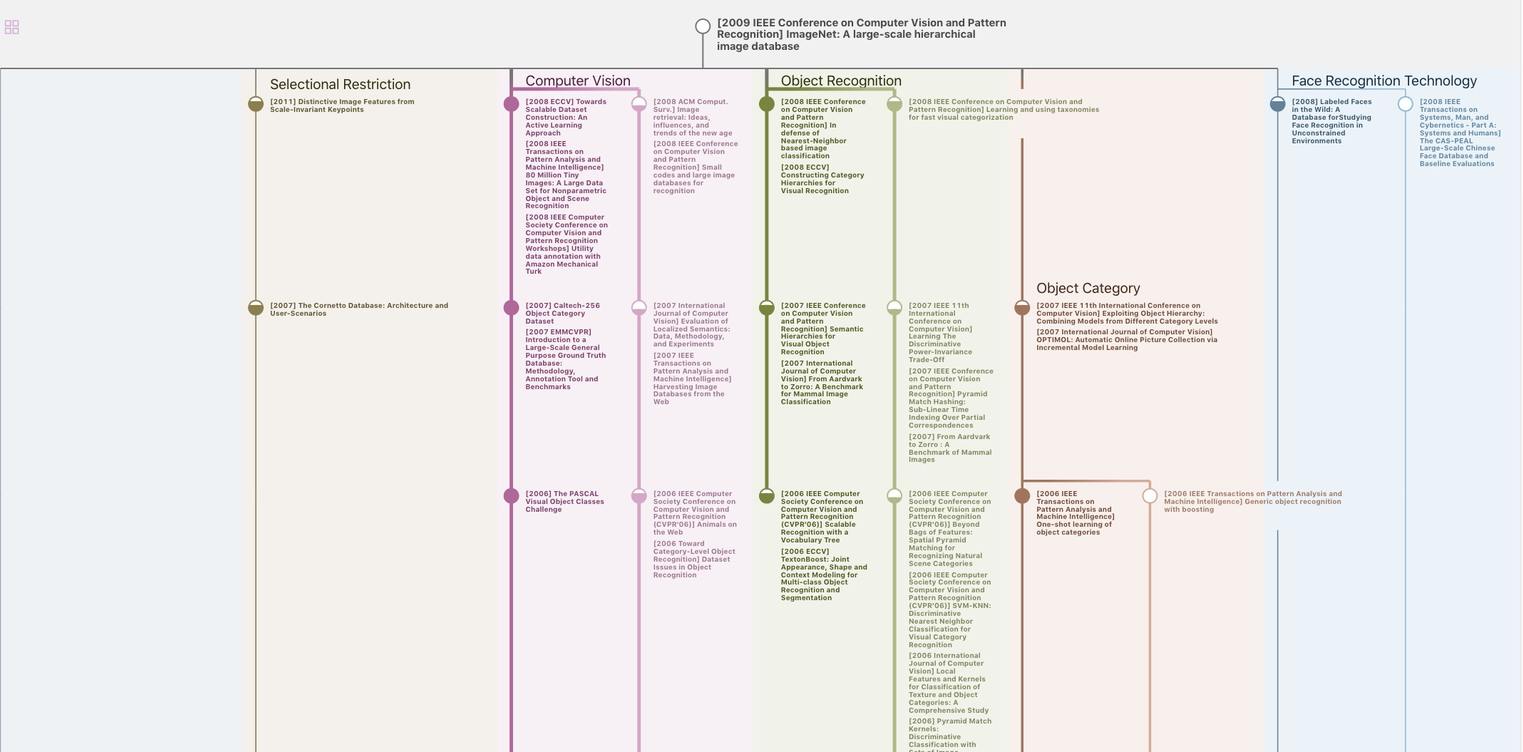
生成溯源树,研究论文发展脉络
Chat Paper
正在生成论文摘要