Establishing the Contaminating Effect of Metadata Feature Inclusion in Machine-Learned Network Intrusion Detection Models
GI International Conference on Detection of Intrusions and Malware & Vulnerability Assessment (DMIVA)(2022)
摘要
Modern datasets in intrusion detection are designed to be evaluated by machine learning techniques and often contain metadata features which ought to be removed prior to training. Unfortunately many published articles include (at least) one such metadata feature in their models, namely destination port. In this article, it is shown experimentally that this feature acts as a prime target for shortcut learning. When used as the only predictor, destination port can separate ten state of the art intrusion detection datasets (CIC collection, UNSW-NB15, CIDDS collection, CTU-13, NSL-KDD and ISCX-IDS2012) with 70 to 100% accuracy on class-balanced test sets. Any model that includes this feature will learn this strong relationship during training which is only meaningful within the dataset. Dataset authors can take countermeasures against this influence, but when applied properly, the feature becomes non-informative and could just as easily not have been part of the dataset in the first place. Consequently, this is the central recommendation in this article. Dataset users should not include destination port (or any other metadata feature) in their models and dataset authors should avoid giving their users the opportunity to use them.
更多查看译文
关键词
Intrusion detection,Machine learning,Shortcut learning,Dataset issues
AI 理解论文
溯源树
样例
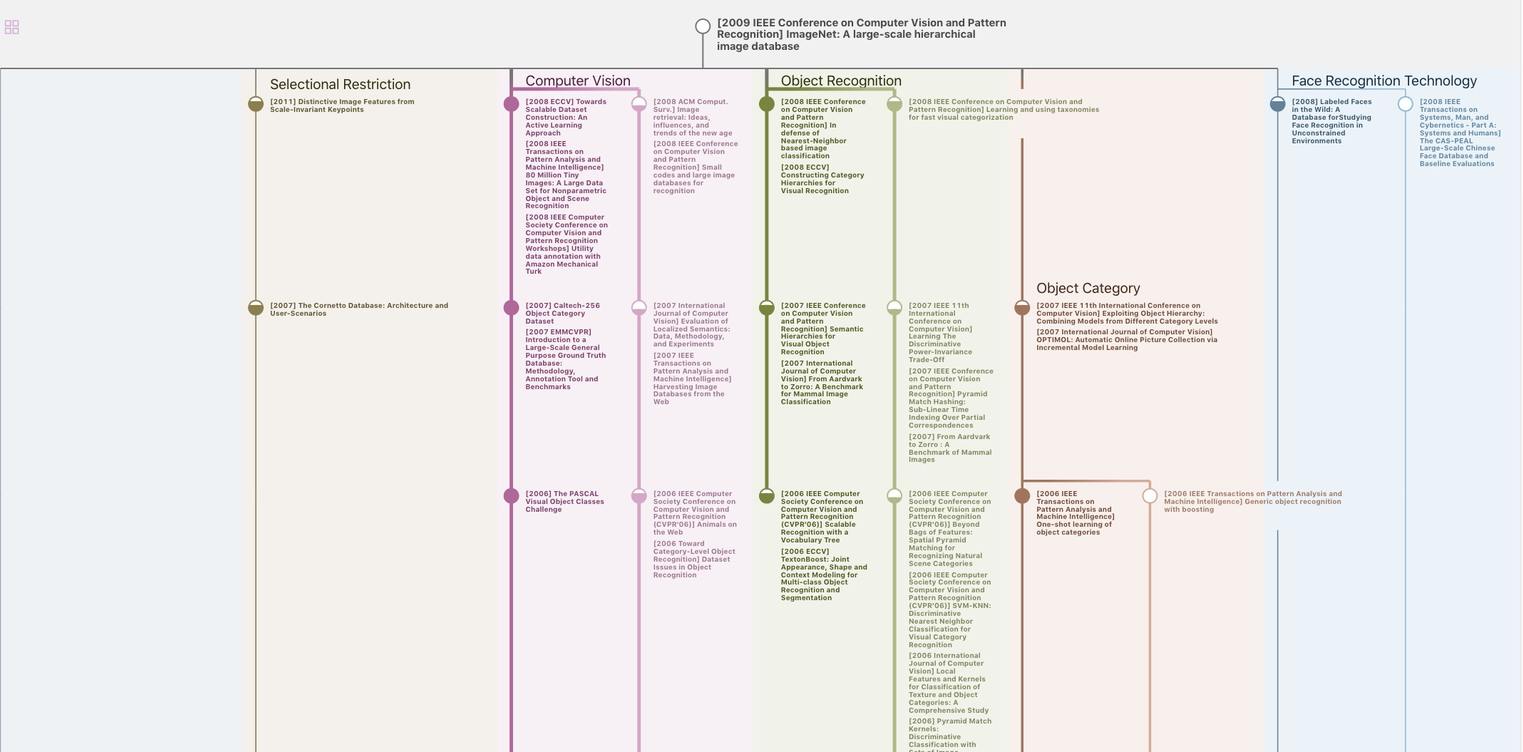
生成溯源树,研究论文发展脉络
Chat Paper
正在生成论文摘要