ODDITY: An Ensemble Framework Leverages Contrastive Representation Learning for Superior Anomaly Detection
International Conference on Information and Communication Security (ICICS)(2022)
摘要
Ensemble approaches are promising for anomaly detection due to the heterogeneity of network traffic. However, existing ensemble approaches lack applicability and efficiency. We propose ODDITY, a new end-to-end data-driven ensemble framework. ODDITY use Diverse Autoencoders trained on a pre-clustered subset with contrastive representation learning to encourage base-leaners to give distinct predictions. Then, ODDITY combines the extracted features with a supervised gradient boosting meta-learner. Experiments using benchmarking and real-world network traffic datasets demonstrate that ODDITY is superior in terms of efficiency and precision. ODDITY averages 0.8350 AUPRC on benchmarking datasets (10% better than traditional machine learning algorithms and 6% better than the state-of-the-art semi-supervised ensemble method). ODDITY also outperforms the state-of-the-art on real-world datasets regarding better detection accuracy and speed. Moreover, ODDITY is more resilient to evasion attacks and has a promising potential for unsupervised anomaly detection.
更多查看译文
关键词
Anomaly detection,Ensemble methods,Semi-supervised settings,Intrusion detection,Auto encoder
AI 理解论文
溯源树
样例
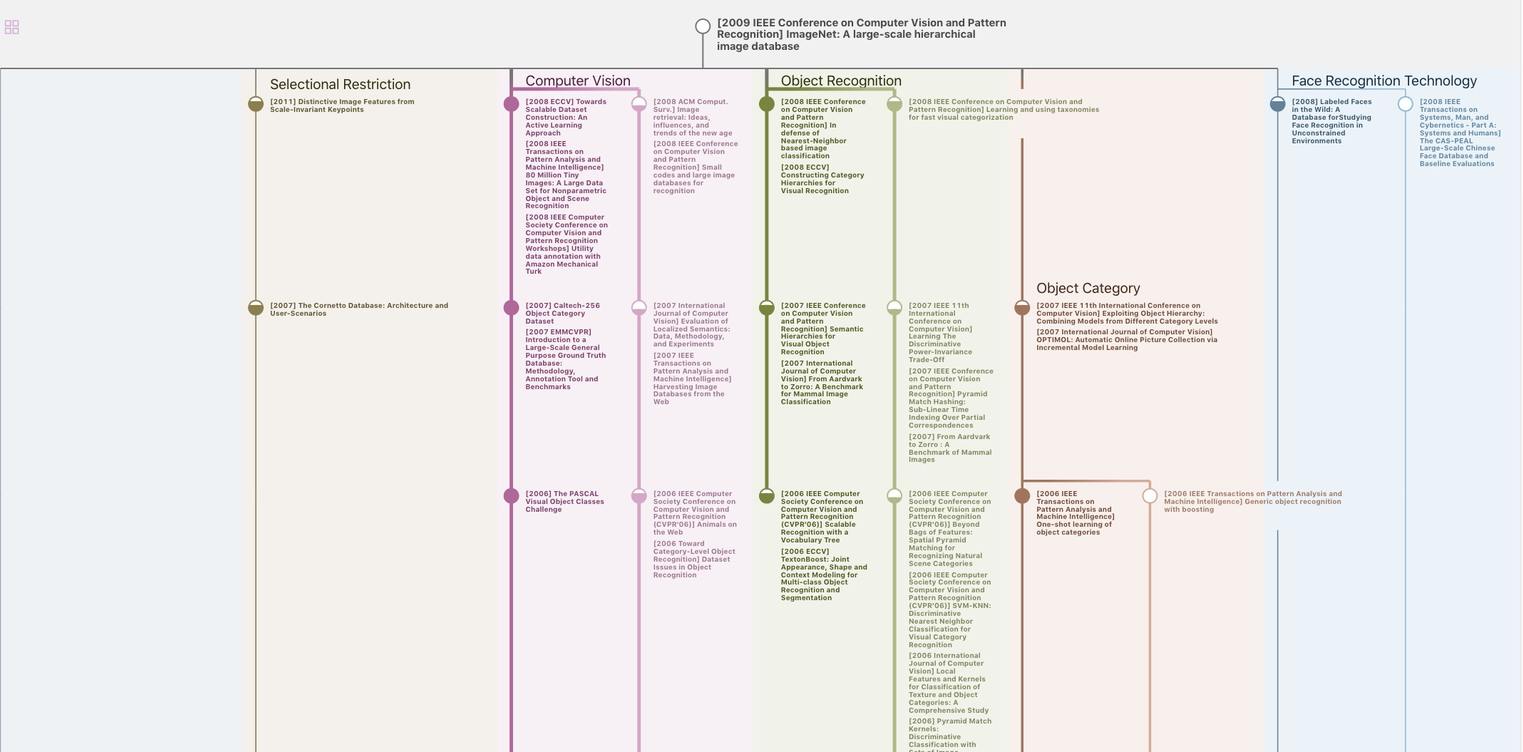
生成溯源树,研究论文发展脉络
Chat Paper
正在生成论文摘要