A Reinforcement Learning-Based Routing Strategy for Elastic Network Slices
IEEE International Conference on Communications (ICC)(2022)
摘要
This paper addresses a routing selection strategy for elastic network slices that dynamically adjust required resources over time. When admitting elastic initial slice requests, sufficient spare resources on the same path should be reserved to allow existing elastic slices to increase their bandwidth dynamically. We demonstrate a deep Reinforcement Learning (RL) model to intelligently make routing choice decisions for elastic slice requests and inelastic slice requests. This model achieves higher revenue and higher acceptance rates compared to traditional heuristic methods. Due to the lightness of this model, it can be deployed in an embedded system. We can also use a relatively small amount of data to train the model and achieve stable performance. Also, we introduce a Recurrent Neural Network to auto-encode the variable-size environment and train the encoder together with the RL model.
更多查看译文
关键词
network slice,reinforcement learning,routing,policy gradient
AI 理解论文
溯源树
样例
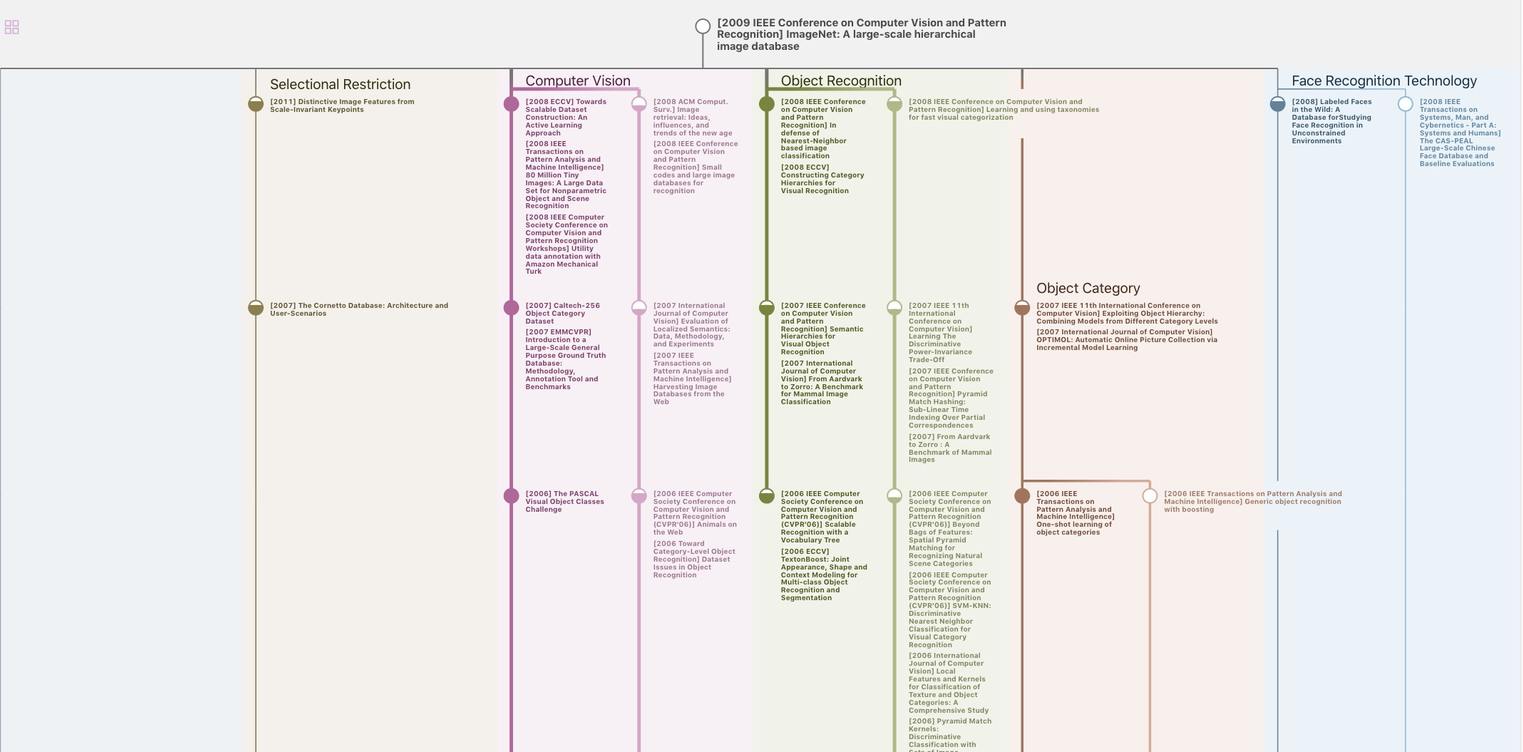
生成溯源树,研究论文发展脉络
Chat Paper
正在生成论文摘要