Moneo: Non-intrusive Fine-grained Monitor for AI Infrastructure
IEEE International Conference on Communications (ICC)(2022)
摘要
Cloud-based AI infrastructure is increasingly important, especially on large-scale distributed training. To improve its efficiency and serviceability, real-time monitoring of the infrastructure and profiling the workload are proved to be the effective approach empirically. However, cloud environment poses great challenges as service providers cannot interfere with their tenants' workloads or touch user data, thus previous instrumentation-based monitoring approach cannot be applied, nor does the workload trace collection. We propose Moneo, a non-intrusive cloud-friendly monitoring system for AI infrastructure. Moneo is capable of intelligently collecting the key architecture-level metrics at finer granularity in real-time without instrumenting or tracing the workloads, which has been deployed in real production cloud, Azure. We analyze the results reported by Moneo for typical large-scale distributed AI workloads from real deployment. Results demonstrate that Moneo can effectively help service providers understand the real resource usage patterns of various AI workloads and real networking requirements, so as to get valuable findings help improve the efficiency of cloud infrastructure and optimize the software stack with the consideration of the characteristic resource usage requirements for different AI workloads.
更多查看译文
关键词
AI infrastructure,monitor,cloud,distributed training
AI 理解论文
溯源树
样例
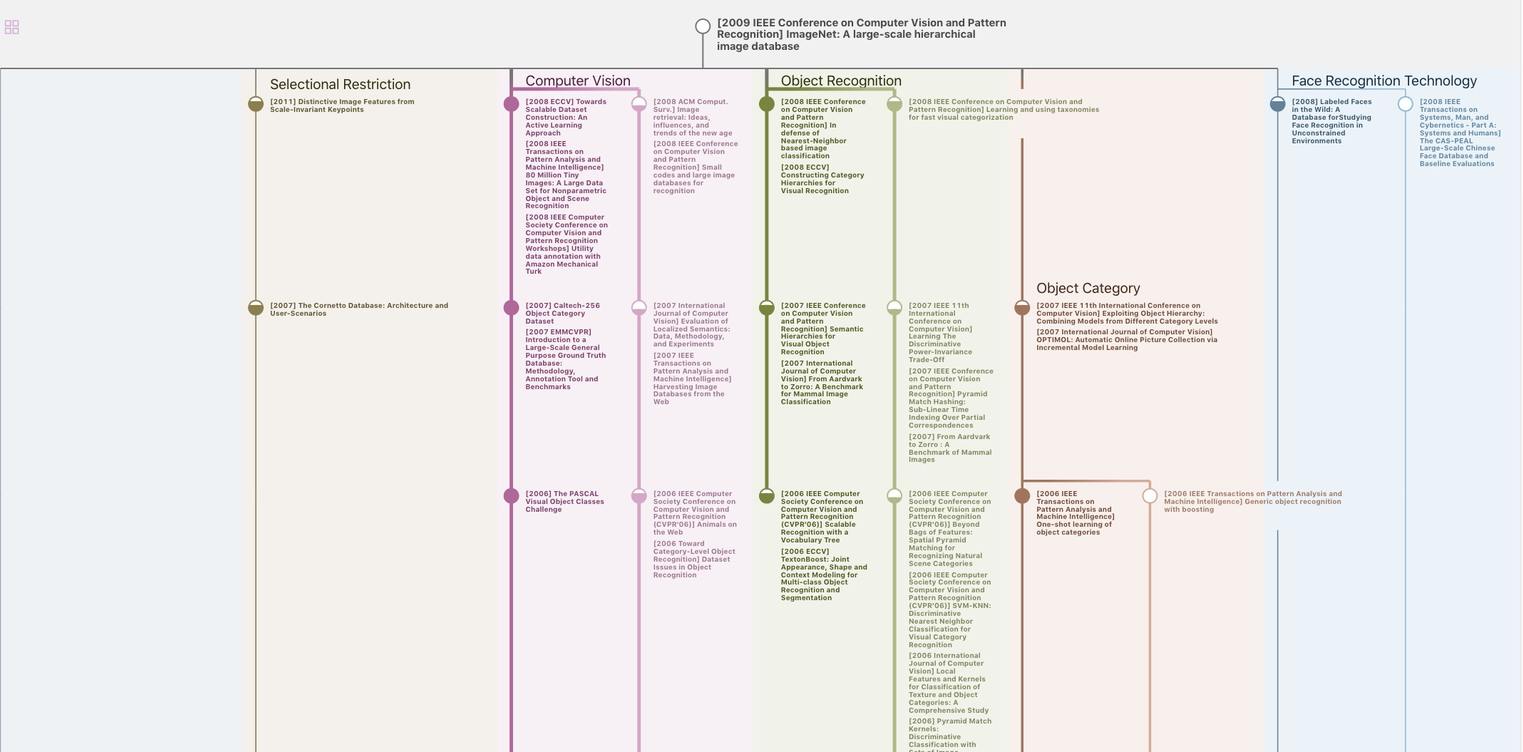
生成溯源树,研究论文发展脉络
Chat Paper
正在生成论文摘要